Deep Learning in the prediction of Angiography-proven Severe Coronary Stenosis in Patients with Apparently Normal Electrocardiograms
medrxiv(2022)
摘要
Patients with severe coronary artery stenosis may have apparently normal electrocardiograms (ECGs), making it difficult to detect the adverse health conditions during screening or physical examinations, resulting in them missing the optimal window of treatment. The goal of this study was to develop an artificial intelligence-based ECG model which can distinguish severe coronary stenosis (≥ 90%) from no or mild coronary stenosis (< 50%) in patients with apparently normal ECGs. Deep learning (DL) models trained from scratch with pre-trained parameters (transfer learning) were tested on ECG alone as well as on ECG along with clinical information (age, sex, hypertension, diabetes, dyslipidemia and smoking status). We also compared the performance of logistic regression for clinical information only and found that DL models trained from scratch with ECG alone can achieve a specificity of 0.746; however, they have low sensitivity, which is comparable to the performance of logistic regression with clinical data. Although adding clinical information to the ECG DL model trained from scratch can improve the sensitivity, it can reduce the specificity. Combining clinical information with the ECG transfer learning model provides the best performance, with a 0.847 AUC, 0.848 sensitivity, and 0.704 specificity.
### Competing Interest Statement
The authors have declared no competing interest.
### Funding Statement
This study was funded by the National Natural Science Foundation of China (No. 62102008), the Research Foundation of Major Science and Technology Projects of Tianjin Municipal Science and Technology Bureau (No. 18ZXRHSY00180), the Tianjin Municipal Natural Science Foundation (No. 21JCZDJC01080), and Tianjin Key Medical Discipline (Specialty) Construction Project (No. TJYXZDXK-029A).
### Author Declarations
I confirm all relevant ethical guidelines have been followed, and any necessary IRB and/or ethics committee approvals have been obtained.
Yes
The details of the IRB/oversight body that provided approval or exemption for the research described are given below:
Ethics committee/IRB of the Second Hospital of Tianjin Medical University (Tianjin, China) gave ethical approval for this work.
I confirm that all necessary patient/participant consent has been obtained and the appropriate institutional forms have been archived, and that any patient/participant/sample identifiers included were not known to anyone (e.g., hospital staff, patients or participants themselves) outside the research group so cannot be used to identify individuals.
Yes
I understand that all clinical trials and any other prospective interventional studies must be registered with an ICMJE-approved registry, such as ClinicalTrials.gov. I confirm that any such study reported in the manuscript has been registered and the trial registration ID is provided (note: if posting a prospective study registered retrospectively, please provide a statement in the trial ID field explaining why the study was not registered in advance).
Yes
I have followed all appropriate research reporting guidelines and uploaded the relevant EQUATOR Network research reporting checklist(s) and other pertinent material as supplementary files, if applicable.
Yes
Data used for this study are not publicly available due to privacy concerns. Part of the data is available upon request to the corresponding author.
更多查看译文
关键词
severe coronary stenosis,deep learning,prediction,angiography-proven
AI 理解论文
溯源树
样例
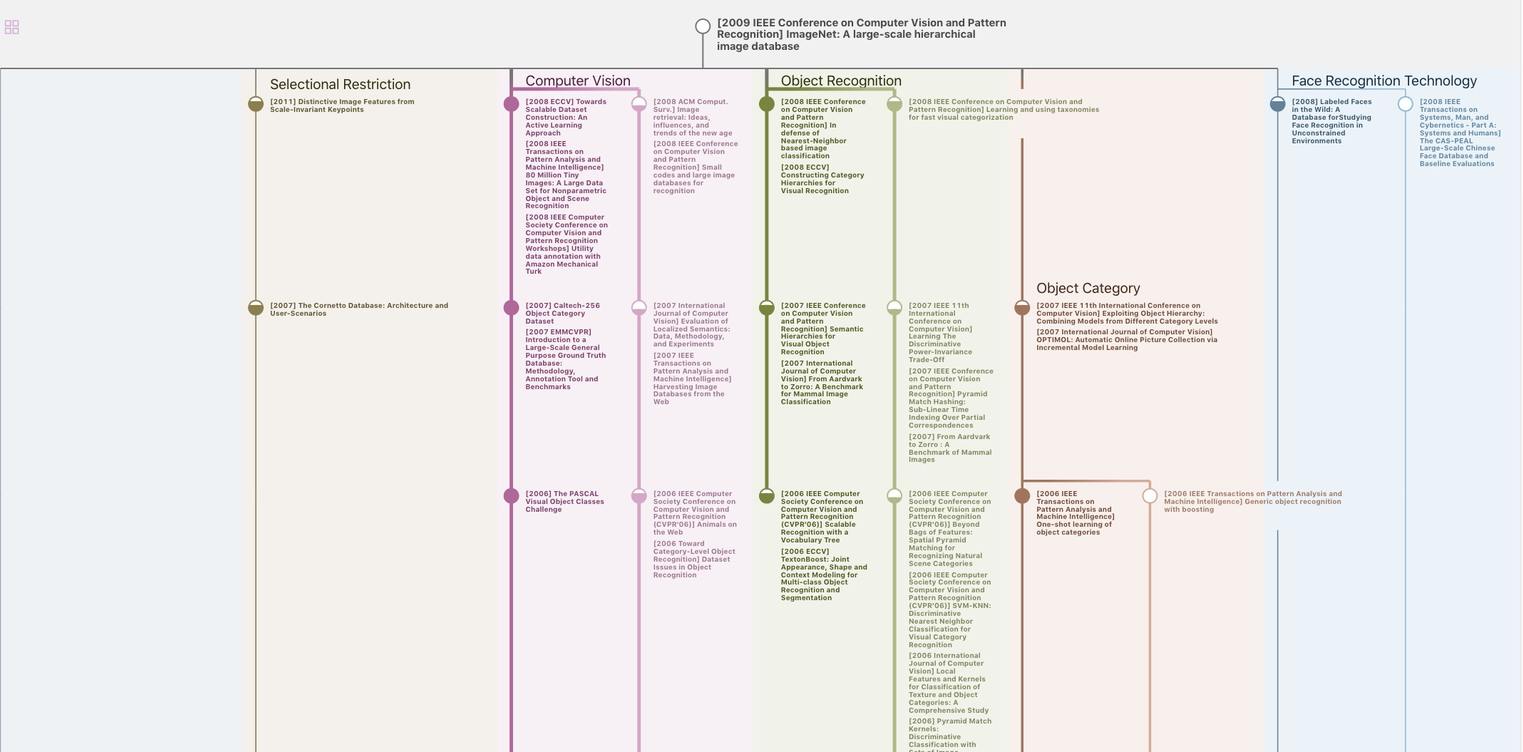
生成溯源树,研究论文发展脉络
Chat Paper
正在生成论文摘要