Performance drift is a major barrier to the safe use of machine learning in cardiac surgery
medRxiv (Cold Spring Harbor Laboratory)(2023)
摘要
Objectives The Society of Thoracic Surgeons (STS), and EuroSCORE II (ES II) risk scores, are the most commonly used risk prediction models for adult cardiac surgery post-operative in-hospital mortality. However, they are prone to miscalibration over time, and poor generalisation across datasets and their use remain controversial. It has been suggested that using Machine Learning (ML) techniques, a branch of Artificial intelligence (AI), may improve the accuracy of risk prediction. Despite increased interest, a gap in understanding the effect of dataset drift on the performance of ML over time remains a barrier to its wider use in clinical practice. Dataset drift occurs when a machine learning system underperforms because of a mismatch between the dataset it was developed and the data on which it is deployed. Here we analyse this potential concern in a large United Kingdom (UK) database.
Methods A retrospective analyses of prospectively routinely gathered data on adult patients undergoing cardiac surgery in the UK between 2012-2019. We temporally split the data 70:30 into a training and validation subset. ES II and five ML mortality prediction models were assessed for relationships between and within variable importance drift, performance drift and actual dataset drift using temporal and non-temporal invariant consensus scoring, combining geometric average results of all metrics as the Clinical Effective Metric (CEM).
Results A total of 227,087 adults underwent cardiac surgery during the study period with a mortality rate of 2.76%. There was a strong evidence of decrease in overall performance across all models (p < 0.0001). Xgboost (CEM 0.728 95CI: 0.728-0.729) and Random Forest (CEM 0.727 95CI 0.727-0.728) were the best overall performing models both temporally and non-temporally. ES II perfomed worst across all comparisons. Sharp changes in variable importance and dataset drift between 2017-10 to 2017-12, 2018-06 to 2018-07 and 2018-12 to 2019-02 mirrored effects of performance decrease across models.
Conclusions Combining the metrics covering all four aspects of discrimination, calibration, clinical usefulness and overall accuracy into a single consensus metric improved the efficiency of cognitive decision-making. All models show a decrease in at least 3 of the 5 individual metrics. CEM and variable importance drift detection demonstrate the limitation of logistic regression methods used for cardiac surgery risk prediction and the effects of dataset drift. Future work will be required to determine the interplay between ML and whether ensemble models could take advantage of their respective performance advantages.
Central message ML performance decreases over time due to dataset drift, but remains superior to ES II. Therefore regular assessment and modification of ML models may be preferable.
Prospective message A gap in understanding the effect of dataset drift on the performance of ML models over time presents a major barrier to their clinical application. Xgboost and Random Forest have shown superior performance both temporally and non-temporally against ES II. However, a decrease in model performance of all models due to dataset drift suggests the need for regular drift monitoring.
### Competing Interest Statement
The authors have declared no competing interest.
### Funding Statement
This work was supported by a grant from the BHF-Turing Institute and the NIHR Biomedical Research Centre at University Hospitals Bristol and Weston NHS Foundation Trust and the University of Bristol.
### Author Declarations
I confirm all relevant ethical guidelines have been followed, and any necessary IRB and/or ethics committee approvals have been obtained.
Yes
The details of the IRB/oversight body that provided approval or exemption for the research described are given below:
The study was approved by the Health Research Authority (HRA) and Health and Care Research Wales (HCRW) in 23 of July 2019, IRAS project ID: 257758 and a waiver for patients' consent was obtained.
I confirm that all necessary patient/participant consent has been obtained and the appropriate institutional forms have been archived, and that any patient/participant/sample identifiers included were not known to anyone (e.g., hospital staff, patients or participants themselves) outside the research group so cannot be used to identify individuals.
Yes
I understand that all clinical trials and any other prospective interventional studies must be registered with an ICMJE-approved registry, such as ClinicalTrials.gov. I confirm that any such study reported in the manuscript has been registered and the trial registration ID is provided (note: if posting a prospective study registered retrospectively, please provide a statement in the trial ID field explaining why the study was not registered in advance).
Yes
I have followed all appropriate research reporting guidelines and uploaded the relevant EQUATOR Network research reporting checklist(s) and other pertinent material as supplementary files, if applicable.
Yes
All data used in this study are from the National Adult Cardiac Surgery Audit (NACSA) dataset. These data may be requested from Healthcare Quality Improvement Partnership (HQIP).
* AUC
: area under receiver operating characteristic curve
CEM
: Clinical Effective Metric
ECE
: Expected Calibration Error
ES II
: Euroscore II
AI
: Artificial intelligence
ML
: machine learning
RF
: random forest
NN
: Neural Network (Neuronetwork)
SVM
: support vector machine
XGBoost
: extreme gradient boosted trees
: Ensemble using several models to derive a consensus prediction
SHAP
: (SHapley Additive exPlanations)
更多查看译文
关键词
cardiac surgery,machine learning,performance
AI 理解论文
溯源树
样例
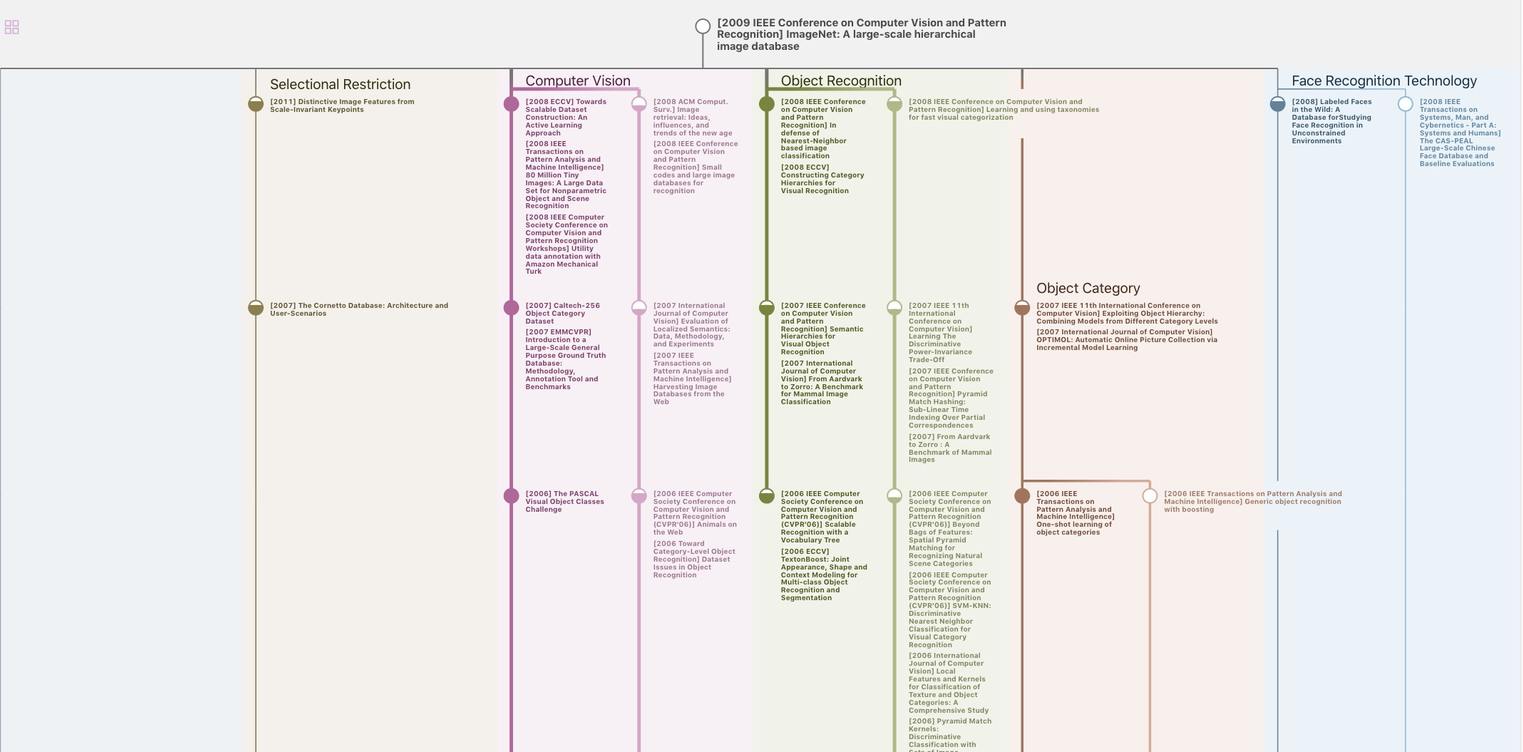
生成溯源树,研究论文发展脉络
Chat Paper
正在生成论文摘要