An Expert-guided Hierarchical Graph Attention Network for Post-traumatic Stress Disorder Highly-associative Genetic Biomarkers Identification
medRxiv (Cold Spring Harbor Laboratory)(2023)
摘要
Post-traumatic Stress Disorder (PTSD) is a common debilitating mental disorder, that occurs in some individuals following extremely traumatic events. Traditional identification of Genetic Markers (GM) for PTSD is mainly based on a statistical clinical approach by comparing PTSD patients with normal controls. However, these statistical studies present limitations, often generating inconsistent results. Few studies have yet examined thoroughly the role of somatic mutations, PTSD disease pathways and their relationships. Capitalizing on deep learning techniques, we have developed a novel hierarchical graph attention network to identify highly correlational GM (HGMs) of PTSD. The network presents the following novelties: First, both a hierarchical graph structure and a graph attention mechanism have been integrated into a model to develop a graph attention network (GAtN) model. Second, domain-specific knowledge, including somatic mutations, genes, PTSD pathways and their correlations have been incorporated into the graph structures. Third, 12 somatic mutations having high or moderate impacts on proteins or genes have been identified as the potential HGMs for PTSD. Fourth, our study is carefully guided by prominent PTSD literature or clinical experts of the field; any high saliency HGMs generated from our model are further verified by existing PTSD-related authoritative medical journals. Our study illustrates the utility and significance of a hybrid approach, integrating both AI and expert-guided/domain-specific knowledge for thorough identification of biomarkers of PTSD, while building on the nature of convergence and divergence of PTSD pathways. Our expert-guided AI-driven methodology can be extended to other pathological-based HGM identification studies; it will transform the methodology of biomarker identification for different life-threatening diseases to speed up the complex lengthy procedures of new biomarkers identification.
### Competing Interest Statement
The authors have declared no competing interest.
### Funding Statement
This research is supported in part by the US National Academy of Medicine Healthy Longevity Catalyst Award (Hong Kong), 2021 and 2022.
### Author Declarations
I confirm all relevant ethical guidelines have been followed, and any necessary IRB and/or ethics committee approvals have been obtained.
Yes
The details of the IRB/oversight body that provided approval or exemption for the research described are given below:
The study used gene expression data openly available at Gene Expression Omnibus ( ) with the accession number: GSE109409.
I confirm that all necessary patient/participant consent has been obtained and the appropriate institutional forms have been archived, and that any patient/participant/sample identifiers included were not known to anyone (e.g., hospital staff, patients or participants themselves) outside the research group so cannot be used to identify individuals.
Yes
I understand that all clinical trials and any other prospective interventional studies must be registered with an ICMJE-approved registry, such as ClinicalTrials.gov. I confirm that any such study reported in the manuscript has been registered and the trial registration ID is provided (note: if posting a prospective study registered retrospectively, please provide a statement in the trial ID field explaining why the study was not registered in advance).
Yes
I have followed all appropriate research reporting guidelines and uploaded the relevant EQUATOR Network research reporting checklist(s) and other pertinent material as supplementary files, if applicable.
Yes
All data produced in the present study are available upon reasonable request to the authors.
更多查看译文
关键词
hierarchical graph attention network,biomarkers,expert-guided,post-traumatic,highly-associative
AI 理解论文
溯源树
样例
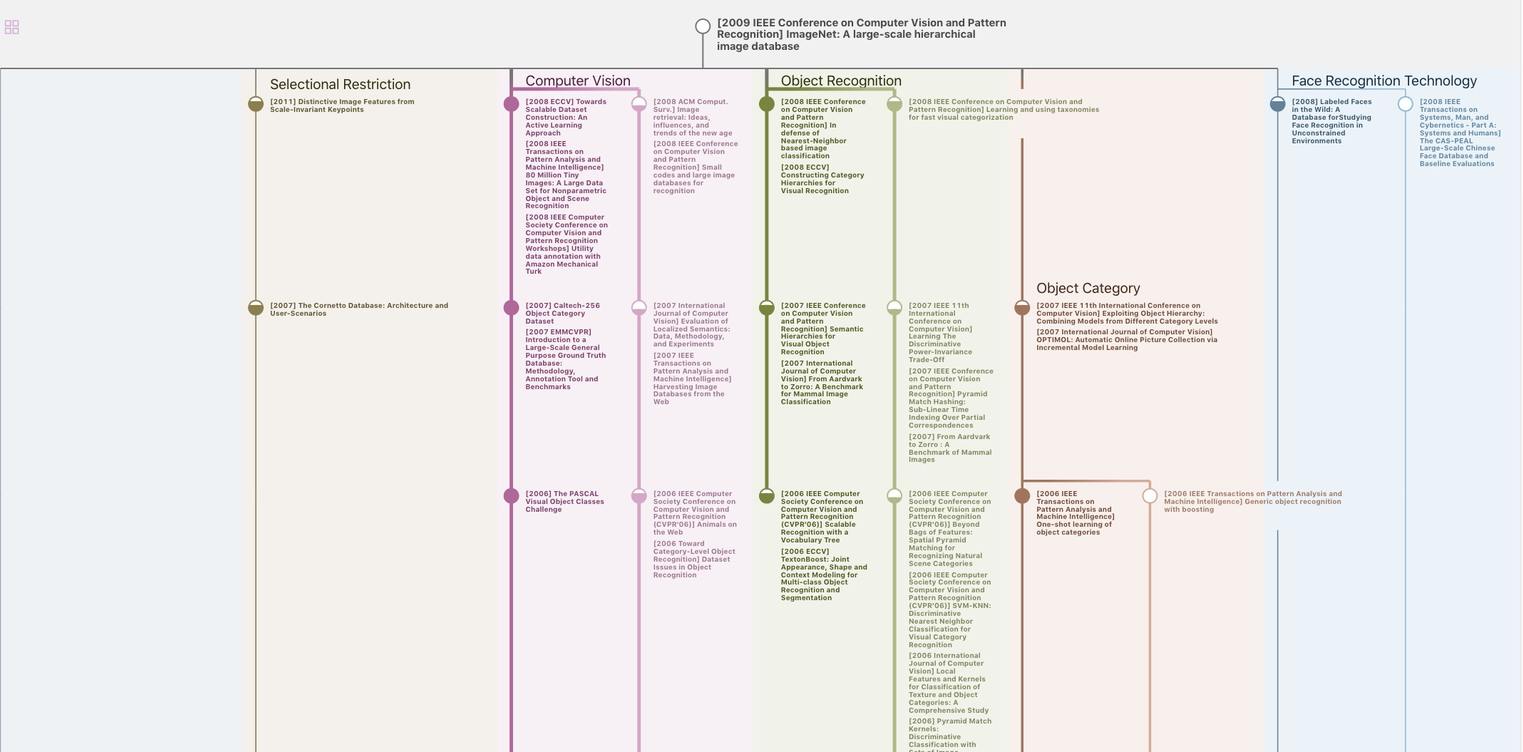
生成溯源树,研究论文发展脉络
Chat Paper
正在生成论文摘要