Predicting seizure outcome after epilepsy surgery: do we need more complex models, larger samples, or better data?
Epilepsia(2023)
摘要
Objective The accurate prediction of seizure freedom after epilepsy surgery remains challenging. We investigated if 1) training more complex models, 2) recruiting larger sample sizes, or 3) using data-driven selection of clinical predictors would improve our ability to predict post-operative seizure outcome. We also conducted the first external validation of a machine learning model trained to predict post-operative seizure outcome.
Methods We performed a retrospective cohort study of 797 children who had undergone resective or disconnective epilepsy surgery at a single tertiary center. We extracted patient information from medical records and trained three models – a logistic regression, a multilayer perceptron, and an XGBoost model – to predict one-year post-operative seizure outcome on our dataset. We evaluated the performance of a recently published XGBoost model on the same patients. We further investigated the impact of sample size on model performance, using learning curve analysis to estimate performance at samples up to N =2,000. Finally, we examined the impact of predictor selection on model performance.
Results Our logistic regression achieved an accuracy of 72% (95% CI=68-75%, AUC=0.72), while our multilayer perceptron and XGBoost both achieved accuracies of 71% (95% CIMLP=67-74%, AUCMLP=0.70; 95% CIXGBoost own=68-75%, AUCXGBoost own=0.70). There was no significant difference in performance between our three models (all P >0.4) and they all performed better than the external XGBoost, which achieved an accuracy of 63% (95% CI=59-67%, AUC=0.62; P LR=0.005, P MLP=0.01, P XGBoost own=0.01) on our data. All models showed improved performance with increasing sample size, with limited improvements above N =400. The best model performance was achieved with data-driven feature selection.
Significance We show that neither the deployment of complex machine learning models nor the assembly of thousands of patients alone is likely to generate significant improvements in our ability to predict post-operative seizure freedom. We instead propose that improved feature selection alongside collaboration, data standardization, and model sharing is required to advance the field.
### Competing Interest Statement
JHC has acted as an investigator for studies with GW Pharmaceuticals, Zogenix, Vitaflo, Ovid, Marinius, and Stoke Therapeutics. She has been a speaker and on advisory boards for GW Pharmaceuticals, Zogenix, Biocodex, Stoke Therapeutics, and Nutricia; all remuneration has been paid to her department. She is president of the International League against Epilepsy (2021-2025), and chair of the medical boards for Dravet UK, Hope 4 Hypothalamic Hamartoma, and Matthew's friends. MT has received grants from Royal Academy of Engineers and LifeArc. He has received honoraria from Medtronic. LM has received personal consultancy fees from Mendelian Ltd, outside the submitted work. AM has received honoraria from Biocodex and Nutricia, and provided consultancy to Biogen, outside the submitted work. All other authors report no disclosures relevant to the manuscript.
### Funding Statement
This research is supported by the National Institute for Health Research Biomedical Research Centre at Great Ormond Street Hospital (NIHR GOSH BRC). The views expressed are those of the authors and not necessarily those of the NHS, the NIHR or the Department of Health. The NIHR GOSH BRC had no role in the manuscript or the decision to submit it for publication. AC and RJP are supported by GOSH Children's Charity Surgeon Scientist Fellowships. SA is funded by the Rosetrees Trust (A2665). KW is supported by the Wellcome Trust (215901/Z/19/Z). MHE is supported by a Child Health Research Studentship, funded by NIHR GOSH BRC. All other authors report no competing interests.
### Author Declarations
I confirm all relevant ethical guidelines have been followed, and any necessary IRB and/or ethics committee approvals have been obtained.
Yes
The details of the IRB/oversight body that provided approval or exemption for the research described are given below:
The study was approved by the National Research Ethics Service and registered with the Joint Research and Development Office of UCL Great Ormond Street Institute of Child Health and Great Ormond Street Hospital. Informed patient consent for this retrospective assessment of our own clinical data was waived, provided that the data were handled anonymously by the clinical care team.
I confirm that all necessary patient/participant consent has been obtained and the appropriate institutional forms have been archived, and that any patient/participant/sample identifiers included were not known to anyone (e.g., hospital staff, patients or participants themselves) outside the research group so cannot be used to identify individuals.
Yes
I understand that all clinical trials and any other prospective interventional studies must be registered with an ICMJE-approved registry, such as ClinicalTrials.gov. I confirm that any such study reported in the manuscript has been registered and the trial registration ID is provided (note: if posting a prospective study registered retrospectively, please provide a statement in the trial ID field explaining why the study was not registered in advance).
Yes
I have followed all appropriate research reporting guidelines and uploaded the relevant EQUATOR Network research reporting checklist(s) and other pertinent material as supplementary files, if applicable.
Yes
The study's data dictionary, statistical analysis plan, and analytic code will be made available on GitHub (). The full data are not publicly available due to their sensitive nature. Deidentified data will be made available from the corresponding author upon reasonable request.
* ASM
: antiseizure medication
AUC
: Area under the (ROC) curve
CI
: confidence interval
CNV
: copy number variation
EEG
: Electroencephalography
fMRI
: Functional magnetic resonance imaging
IQR
: interquartile range
LR
: Logistic regression
MEG
: Magnetoencephalography
MLP
: Multilayer perceptron
MRI
: Magnetic resonance imaging
OR
: odds ratio
PET
: Positron emission tomography
ROC
: Receiver operating characteristic
SNV
: single nucleotide variation
SPECT
: Single-photon emission computed tomography
更多查看译文
关键词
seizure outcome,epilepsy surgery,better data
AI 理解论文
溯源树
样例
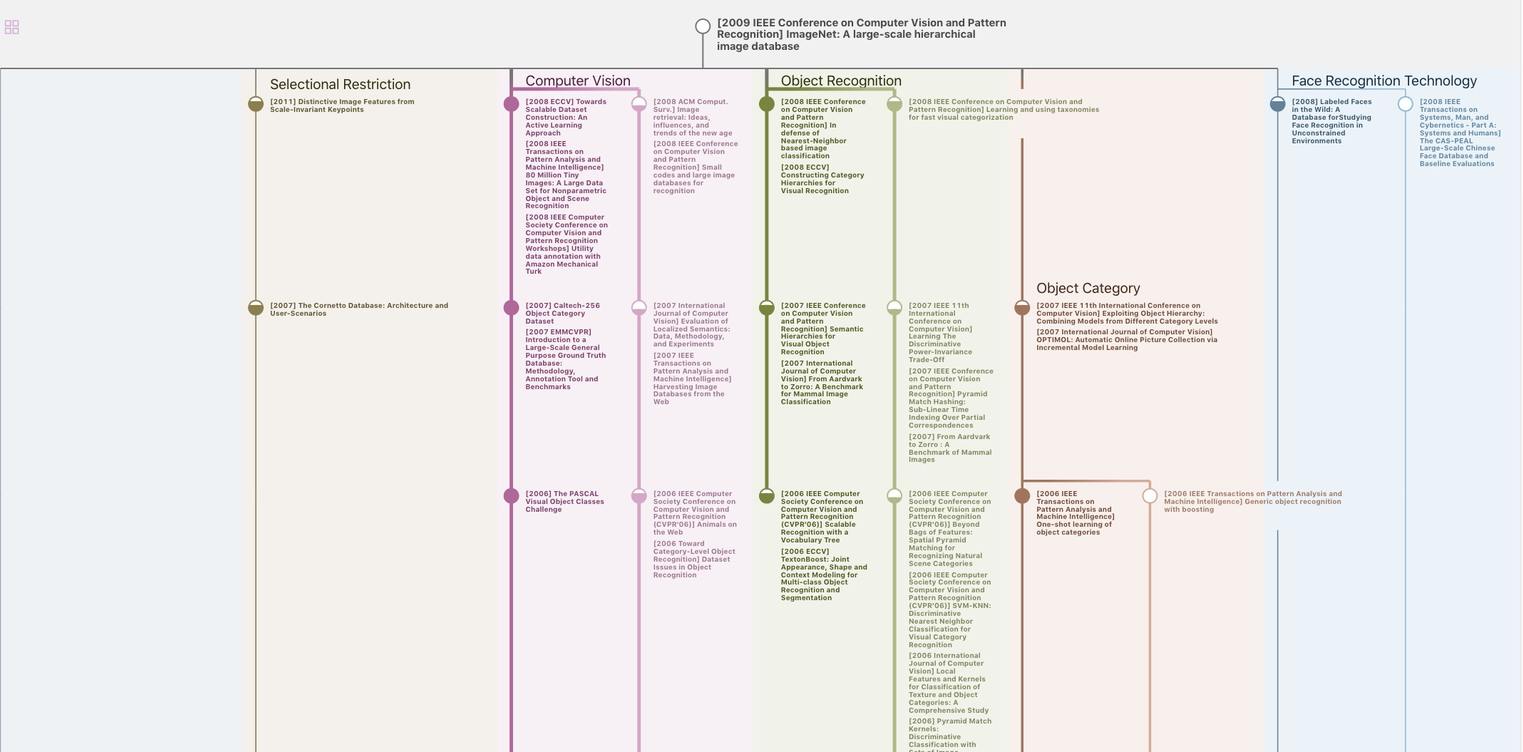
生成溯源树,研究论文发展脉络
Chat Paper
正在生成论文摘要