A Novel Collaborative Learning Model for Teeth and Fillings in Radiographs
medRxiv (Cold Spring Harbor Laboratory)(2023)
摘要
It is critical for dentists to identify and differentiate primary and permanent teeth, fillings, dental restorations and areas with pathological findings when reviewing dental radiographs to ensure that an accurate diagnosis is made and the optimal treatment can be planned. Unfortunately, dental radiographs are sometimes read incorrectly due to human error or low-quality images. While secondary or group review can help catch errors, many dentists work in practice alone and/or do not have time to review all of their patients’ radiographs with another dentist. Artificial intelligence may facilitate the accurate interpretation of radiographs. To help support the review of panoramic radiographs, we developed a novel collaborative learning model that simultaneously identifies and differentiates primary and permanent teeth and detects fillings. We used publicly accessible dental panoramic radiographic images and images obtained from the University of Missouri-Kansas City School of Dentistry to develop and optimize two high-performance classifiers: (1) a system for tooth segmentation that can differentiate primary and permanent teeth and (2) a system to detect dental fillings. By utilizing these high-performance classifiers, we created models that can identify primary and permanent teeth, as well as their associated dental fillings. We also designed a novel method for collaborative learning that utilizes these two classifiers to enhance recognition performance. Our model improves upon the existing machine learning models to simultaneously identify and differentiate primary and permanent teeth, and to identify any associated fillings.
### Competing Interest Statement
The authors have declared no competing interest.
### Funding Statement
The authors disclose receipt of the following financial support for the research, authorship, and/or publication of this article: E. Bumann is supported by a grant from the Robert Wood Johnson Foundations (RWJF) Harold Amos Medical Faculty Development Program and Y. Lee is supported by NSF Grant 1747751. The content is solely the responsibility of the authors and the views expressed here do not necessarily reflect the views of RWJF or NSF.
### Author Declarations
I confirm all relevant ethical guidelines have been followed, and any necessary IRB and/or ethics committee approvals have been obtained.
Yes
The details of the IRB/oversight body that provided approval or exemption for the research described are given below:
The IRB of University of Missouri-Kansas City reviewed and exempted ethical approval for this work.
I confirm that all necessary patient/participant consent has been obtained and the appropriate institutional forms have been archived, and that any patient/participant/sample identifiers included were not known to anyone (e.g., hospital staff, patients or participants themselves) outside the research group so cannot be used to identify individuals.
Yes
I understand that all clinical trials and any other prospective interventional studies must be registered with an ICMJE-approved registry, such as ClinicalTrials.gov. I confirm that any such study reported in the manuscript has been registered and the trial registration ID is provided (note: if posting a prospective study registered retrospectively, please provide a statement in the trial ID field explaining why the study was not registered in advance).
Yes
I have followed all appropriate research reporting guidelines, such as any relevant EQUATOR Network research reporting checklist(s) and other pertinent material, if applicable.
Yes
All data produced in the present study are available upon reasonable request to the authors.
更多查看译文
关键词
radiographs,novel collaborative learning model,teeth,fillings
AI 理解论文
溯源树
样例
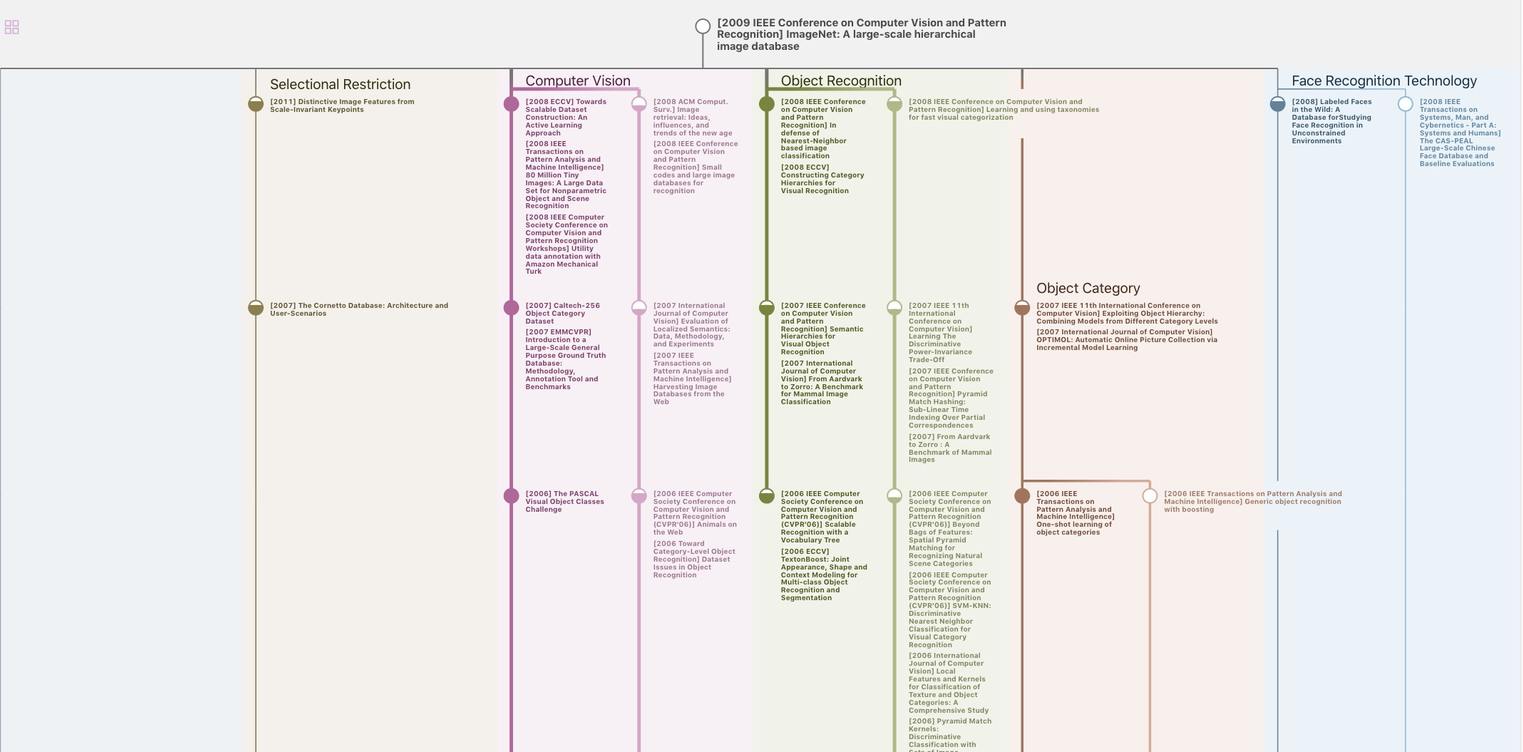
生成溯源树,研究论文发展脉络
Chat Paper
正在生成论文摘要