Computational Phenotypes for Patients with Opioid-Related Disorders Presenting to the Emergency Department
PloS one(2023)
摘要
Objective We aimed to discover computationally-derived phenotypes of opioid-related patient presentations to the emergency department (ED) via clinical notes and structured electronic health record (EHR) data.
Methods This was a retrospective study of ED visits from 2013-2020 across ten sites within a regional healthcare network. We derived phenotypes from visits for patients 18 years of age with at least one prior or current documentation of an opioid-related diagnosis. Natural language processing was used to extract clinical entities from notes, which were combined with structured data within the EHR to create a set of features. We performed Latent Dirichlet allocation to identify topics within these features. Groups of patient presentations with similar attributes were identified by cluster analysis.
Results In total 82,577 ED visits met inclusion criteria. The 30 topics discovered ranged from those related to substance use disorder, chronic conditions, mental health, and medical management. Clustering on these topics identified nine unique cohorts with one-year survivals ranging from 84.2-96.8%, rates of one-year ED returns from 9-34%, rates of one-year opioid event 10-17%, rates of medications for opioid use disorder from 17-43%, and a median Carlson comorbidity index of 2-8. Two cohorts of phenotypes were identified related to chronic substance use disorder, or acute overdose.
Conclusions Our results indicate distinct phenotypic clusters with varying patient-oriented outcomes which provide future targets better allocation of resources and therapeutics. This highlights the heterogeneity of the overall population, and the need to develop targeted interventions for each population.
### Competing Interest Statement
The authors have declared no competing interest.
### Funding Statement
This research was supported by the Center for the Clinical Trials Network, National Institute on Drug Abuse, National Institutes of Health through the NIH HEAL InitiativeSM under award number 3UG1DA015831-18S7 and the National Institute of Diabetes And Digestive And Kidney Diseases of the National Institutes of Health under Award Number T35DK104689
### Author Declarations
I confirm all relevant ethical guidelines have been followed, and any necessary IRB and/or ethics committee approvals have been obtained.
Yes
The details of the IRB/oversight body that provided approval or exemption for the research described are given below:
THe IRB of Yale University (IRB Registration #: 00011725) gave ethical approval for this work.
I confirm that all necessary patient/participant consent has been obtained and the appropriate institutional forms have been archived, and that any patient/participant/sample identifiers included were not known to anyone (e.g., hospital staff, patients or participants themselves) outside the research group so cannot be used to identify individuals.
Yes
I understand that all clinical trials and any other prospective interventional studies must be registered with an ICMJE-approved registry, such as ClinicalTrials.gov. I confirm that any such study reported in the manuscript has been registered and the trial registration ID is provided (note: if posting a prospective study registered retrospectively, please provide a statement in the trial ID field explaining why the study was not registered in advance).
Yes
I have followed all appropriate research reporting guidelines, such as any relevant EQUATOR Network research reporting checklist(s) and other pertinent material, if applicable.
Yes
All data produced in the present study are available upon reasonable request to the authors
更多查看译文
关键词
computational phenotypes,disorders,patients,opioid-related
AI 理解论文
溯源树
样例
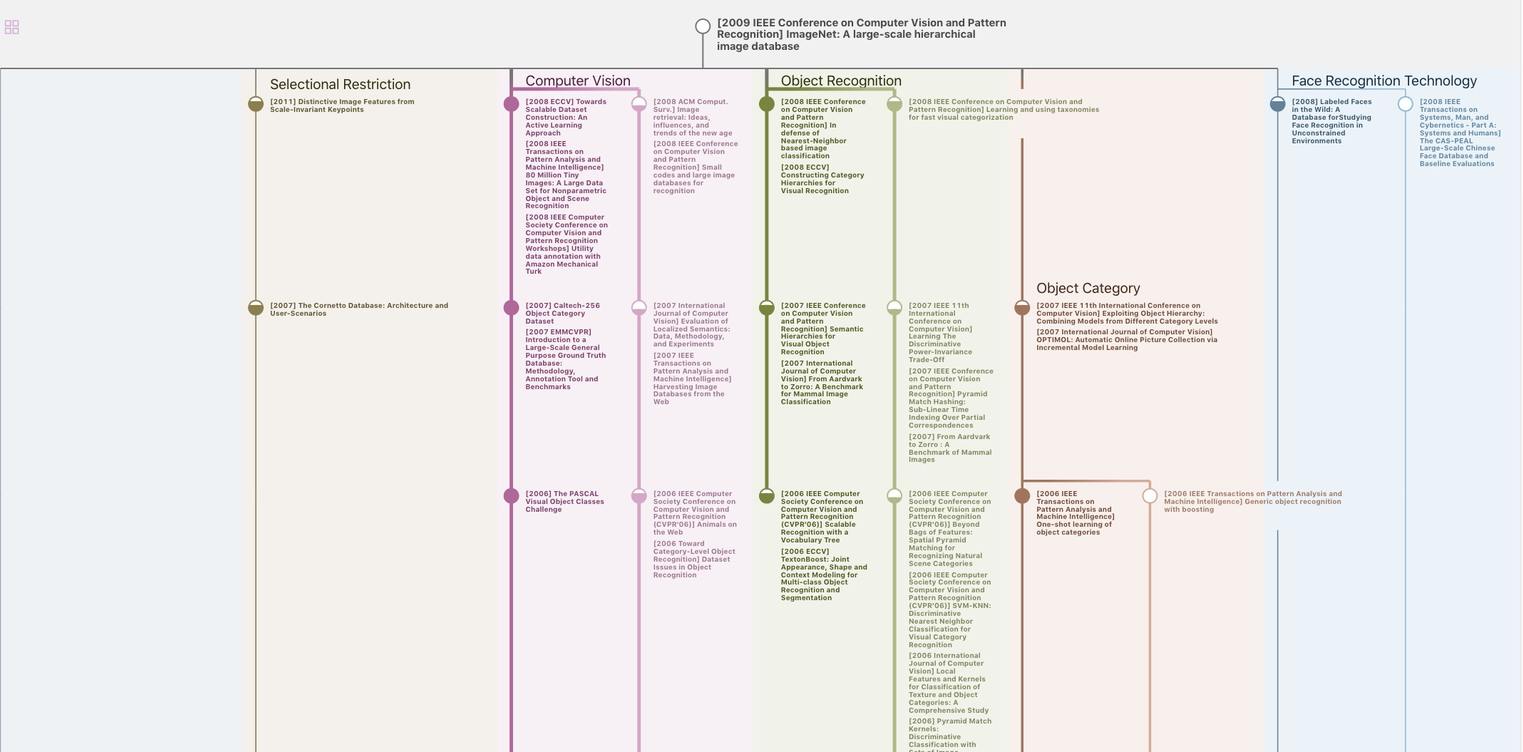
生成溯源树,研究论文发展脉络
Chat Paper
正在生成论文摘要