BioDecoder: A miRNA Bio-interpretable Neural Network Model for Noninvasive Diagnosis of Breast Cancer
medRxiv (Cold Spring Harbor Laboratory)(2023)
摘要
Early diagnosis of breast cancer remains a major clinical challenge. Liquid biopsy has become a powerful tool for cancer diagnosis by the aid of various the state-of-the-art detection technologies and artificial intelligence (AI) methods. Although the prediction performance is superior, the clinical application of existing AI models is greatly limited due to their poor interpretability. Here, we designed a miRNA-Gene-Module-Pathway-Disease biological decoding path, and developed BioDecoder thereof, a miRNA bio-interpretable neural network model for breast cancer early diagnosis. We demonstrated that BioDecoder could achieve early non-invasive diagnosis of breast cancer with a remarkable performance (AUC = 0.989) and showed strong generalizability in an external cohort (AUC = 0.890). Meanwhile, the biologically interpretable results of BioDecoder revealed that significant changes in metabolic pathway and oxidative phosphorylation were the main action pathways of circulating miRNA in breast cancer. Our study indicates that BioDecoder offers the promise of non-invasive early diagnosis of breast cancer and can be generalized to other cancers and corresponding biomarkers.
### Competing Interest Statement
The authors have declared no competing interest.
### Funding Statement
This work was supported by the National Natural Science Foundation of China (32200529 to Dingfeng Wu, 82170542 to Ruixin Zhu, 92251307 to Ruixin Zhu, 82000536 to Na Jiao), and the National Key Research and Development Program of China (2021YFF0703700/2021YFF0703702 to Ruixin Zhu).
### Author Declarations
I confirm all relevant ethical guidelines have been followed, and any necessary IRB and/or ethics committee approvals have been obtained.
Yes
The details of the IRB/oversight body that provided approval or exemption for the research described are given below:
The study used ONLY openly available human data that were originally located at: ArrayExpress (E-GEOD-73002, ) and .
I confirm that all necessary patient/participant consent has been obtained and the appropriate institutional forms have been archived, and that any patient/participant/sample identifiers included were not known to anyone (e.g., hospital staff, patients or participants themselves) outside the research group so cannot be used to identify individuals.
Yes
I understand that all clinical trials and any other prospective interventional studies must be registered with an ICMJE-approved registry, such as ClinicalTrials.gov. I confirm that any such study reported in the manuscript has been registered and the trial registration ID is provided (note: if posting a prospective study registered retrospectively, please provide a statement in the trial ID field explaining why the study was not registered in advance).
Yes
I have followed all appropriate research reporting guidelines, such as any relevant EQUATOR Network research reporting checklist(s) and other pertinent material, if applicable.
Yes
No new sequencing data was used in this paper. All the software packages used in this study are open source and publicly available and the code used in this study is available on GitHub at .
* AI
: Artificial Intelligence
AUC
: Area Under the ROC Curve
BH
: Benjamini-Hochberg
CTC
: Circulating Tumor Cells
ctDNA
: circulating tumor DNA
ctRNA
: circulating tumor RNA
GSEA
: Gene Set Enrichment Analysis
FC
: Fold Change
FCN
: Fully Connected Neural Network
FDR
: False Discovery Rate
KEGG
: Kyoto Encyclopedia of Genes and Genomes
miBDP
: miRNA Biological Decoding Path
OS
: Oversampling
miBDP
: miRNA Biological Decoding Path
RF
: random forest
ROC
: Receiver Operating Characteristic
RT-PCR
: Reverse Transcription-Polymerase Chain Reaction
SMOTE
: Synthetic Minority Oversampling Technique
XAI
: eXplainable Artificial Intelligence
更多查看译文
关键词
breast cancer,bio-interpretable
AI 理解论文
溯源树
样例
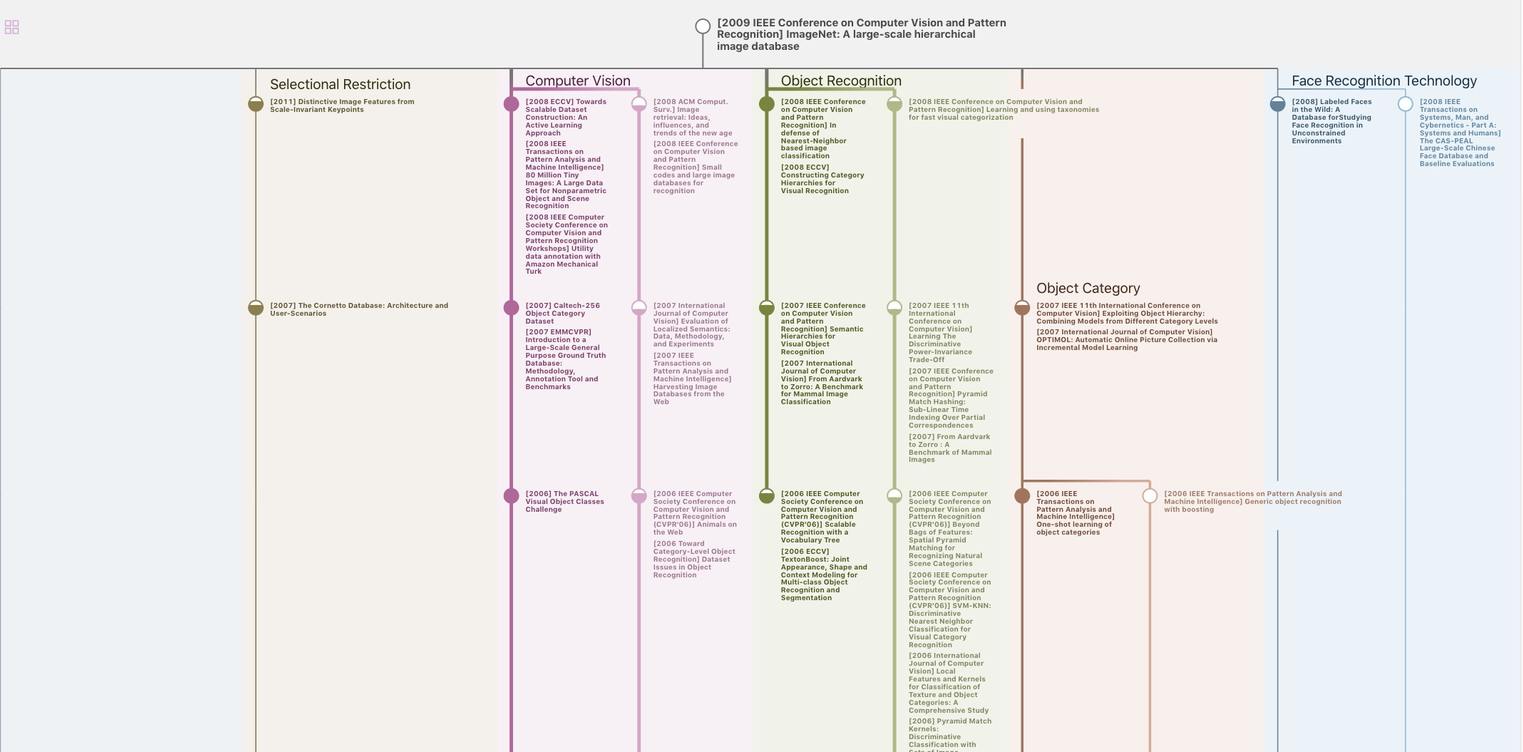
生成溯源树,研究论文发展脉络
Chat Paper
正在生成论文摘要