Pie-Net: Prior-information-enabled deep learning noise reduction for coronary CT angiography acquired with a photon counting detector CT
MEDICAL PHYSICS(2023)
摘要
Background: Photon-counting-detector CT (PCD-CT) enables the production of virtual monoenergetic images (VMIs) at a high spatial resolution (HR) via simultaneous acquisition of multi-energy data. However, noise levels in these HR VMIs are markedly increased. Purpose: To develop a deep learning technique that utilizes a lower noise VMI as prior information to reduce image noise in HR, PCD-CT coronary CT angiography (CTA). Methods: Coronary CTA exams of 10 patients were acquired using PCD-CT (NAEOTOM Alpha, Siemens Healthineers). A prior-information-enabled neural network (Pie-Net) was developed, treating one lower-noise VMI (e.g., 70 keV) as a prior input and one noisy VMI (e.g., 50 keV or 100 keV) as another. For data preprocessing, noisy VMIs were reconstructed by filtered back-projection (FBP) and iterative reconstruction ( IR), which were then subtracted to generate "noise-only" images. Spatial decoupling was applied to the noise-only images to mitigate overfitting and improve randomization. Thicker slice averaging was used for the IR and prior images. The final training inputs for the convolutional neural network (CNN) inside the Pie-Net consisted of thicker-slice signal images with the reinsertion of spatially decoupled noise-only images and the thicker-slice prior images. The CNN training labels consisted of the corresponding thicker-slice label images without noise insertion. Pie-Net's performance was evaluated in terms of image noise, spatial detail preservation, and quantitative accuracy, and compared to a U-net-based method that did not include prior information. Results: Pie-Net provided strong noise reduction, by 95 +/- 1% relative to FBP and by 60 +/- 8% relative to IR. For HR VMIs at different keV (e.g., 50 keV or 100 keV), Pie-Net maintained spatial and spectral fidelity. The inclusion of prior information from the PCD-CT data in the spectral domain was able to improve a robust deep learning-based denoising performance compared to the U-net-based method, which caused some loss of spatial detail and introduced some artifacts. Conclusion: The proposed Pie-Net achieved substantial noise reduction while preserving HR VMI's spatial and spectral properties.
更多查看译文
关键词
coronary computed tomography angiography, deep learning, high resolution, noise reduction, photon counting detector CT, prior information
AI 理解论文
溯源树
样例
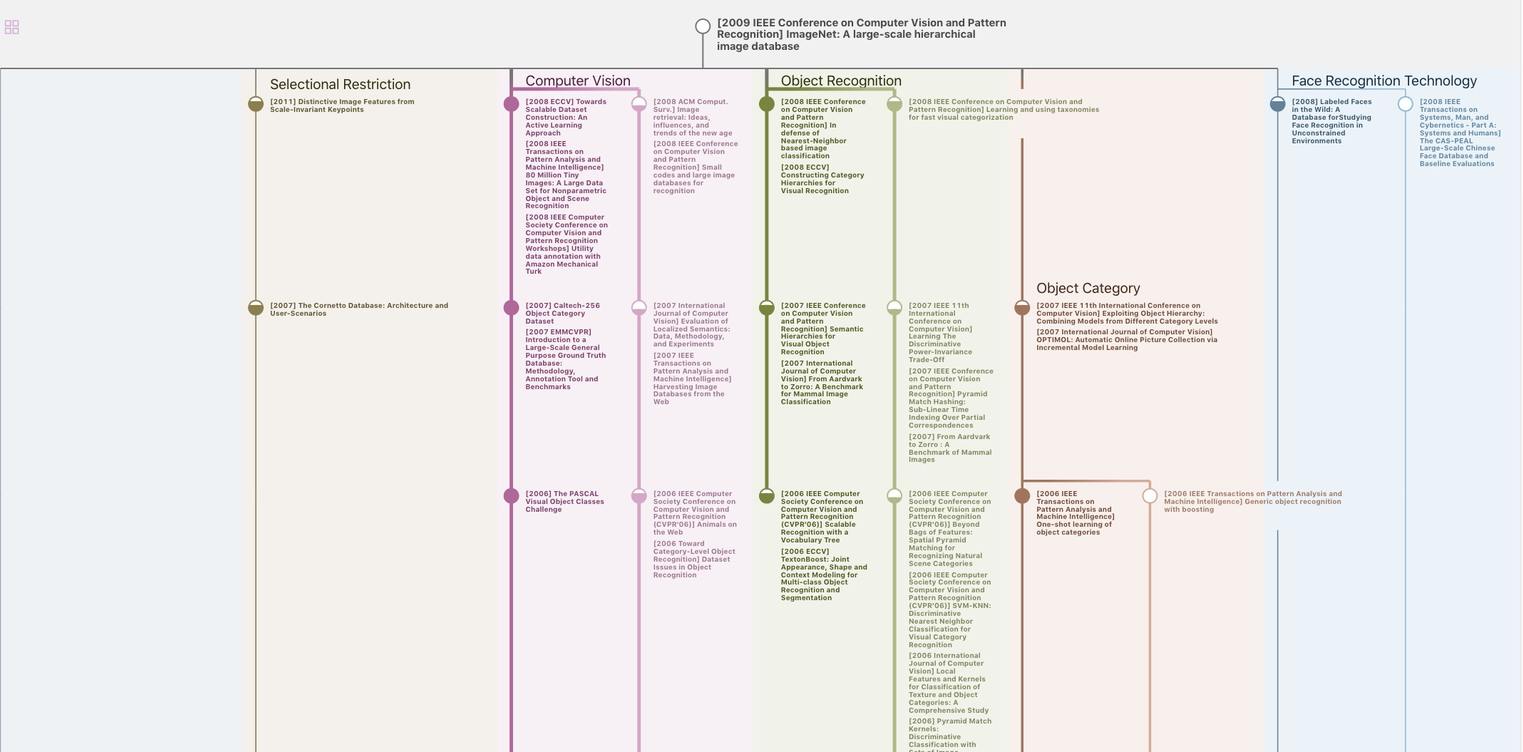
生成溯源树,研究论文发展脉络
Chat Paper
正在生成论文摘要