STGATE: Spatial-temporal graph attention network with a transformer encoder for EEG-based emotion recognition
Frontiers in Human Neuroscience(2023)
摘要
Electroencephalogram (EEG) is a crucial and widely utilized technique in neuroscience research. In this paper, we introduce a novel graph neural network called the spatial-temporal graph attention network with a transformer encoder (STGATE) to learn graph representations of emotion EEG signals and improve emotion recognition performance. In STGATE, a transformer-encoder is applied for capturing time-frequency features which are fed into a spatial-temporal graph attention for emotion classification. Using a dynamic adjacency matrix, the proposed STGATE adaptively learns intrinsic connections between different EEG channels. To evaluate the cross-subject emotion recognition performance, leave-one-subject-out experiments are carried out on three public emotion recognition datasets, i.e., SEED, SEED-IV, and DREAMER. The proposed STGATE model achieved a state-of-the-art EEG-based emotion recognition performance accuracy of 90.37% in SEED, 76.43% in SEED-IV, and 76.35% in DREAMER dataset, respectively. The experiments demonstrated the effectiveness of the proposed STGATE model for cross-subject EEG emotion recognition and its potential for graph-based neuroscience research.
更多查看译文
关键词
EEG-based emotion classification,EEG,deep learning,graph neural network,transformer encoder
AI 理解论文
溯源树
样例
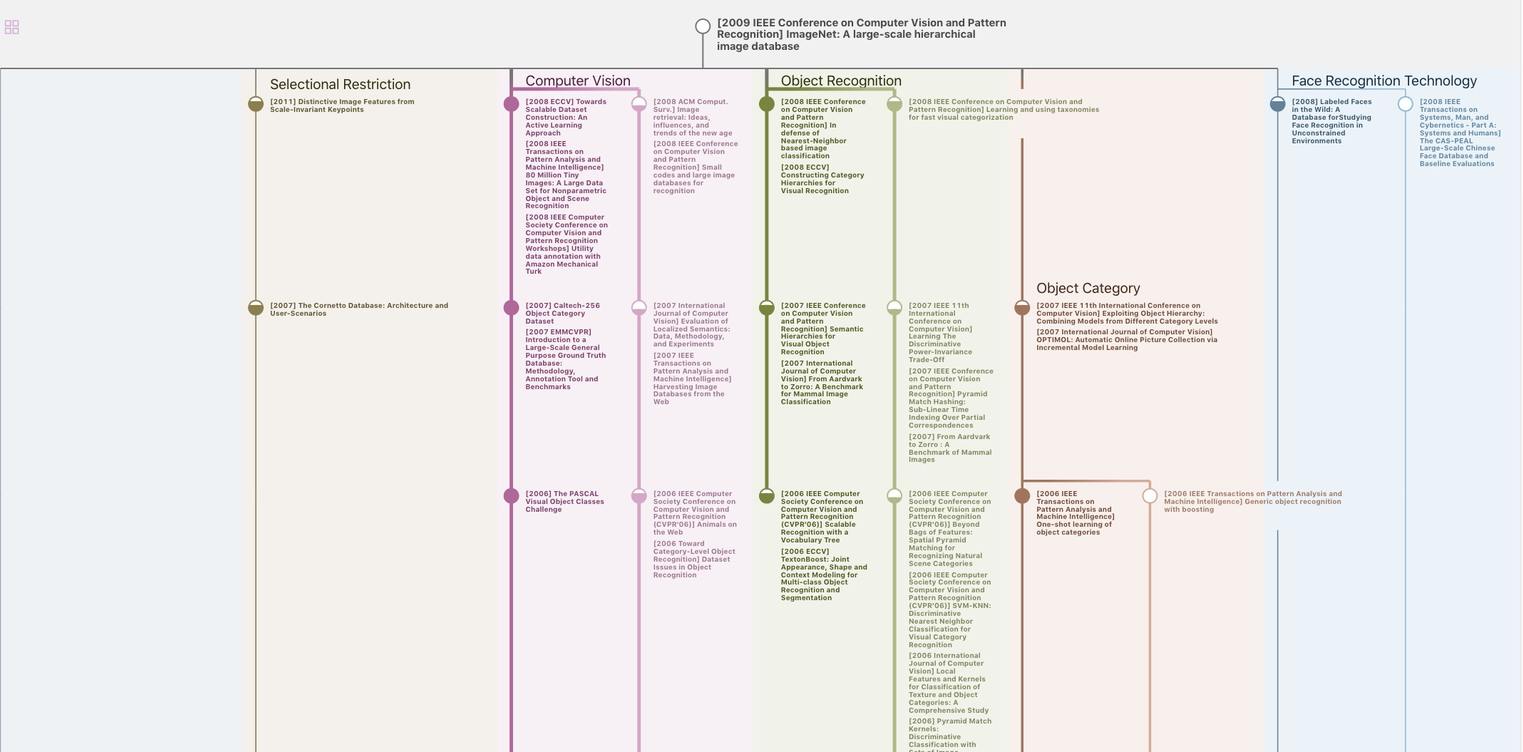
生成溯源树,研究论文发展脉络
Chat Paper
正在生成论文摘要