GDP nowcasting with artificial neural networks: How much does long-term memory matter?
CoRR(2023)
摘要
We apply artificial neural networks (ANNs) to nowcast quarterly GDP growth
for the U.S. economy. Using the monthly FRED-MD database, we compare the
nowcasting performance of five different ANN architectures: the multilayer
perceptron (MLP), the one-dimensional convolutional neural network (1D CNN),
the Elman recurrent neural network (RNN), the long short-term memory network
(LSTM), and the gated recurrent unit (GRU). The empirical analysis presents
results from two distinctively different evaluation periods. The first (2012:Q1
– 2019:Q4) is characterized by balanced economic growth, while the second
(2012:Q1 – 2022:Q4) also includes periods of the COVID-19 recession. According
to our results, longer input sequences result in more accurate nowcasts in
periods of balanced economic growth. However, this effect ceases above a
relatively low threshold value of around six quarters (eighteen months). During
periods of economic turbulence (e.g., during the COVID-19 recession), longer
input sequences do not help the models' predictive performance; instead, they
seem to weaken their generalization capability. Combined results from the two
evaluation periods indicate that architectural features enabling long-term
memory do not result in more accurate nowcasts. Comparing network
architectures, the 1D CNN has proved to be a highly suitable model for GDP
nowcasting. The network has shown good nowcasting performance among the
competitors during the first evaluation period and achieved the overall best
accuracy during the second evaluation period. Consequently, first in the
literature, we propose the application of the 1D CNN for economic nowcasting.
更多查看译文
关键词
long-term
AI 理解论文
溯源树
样例
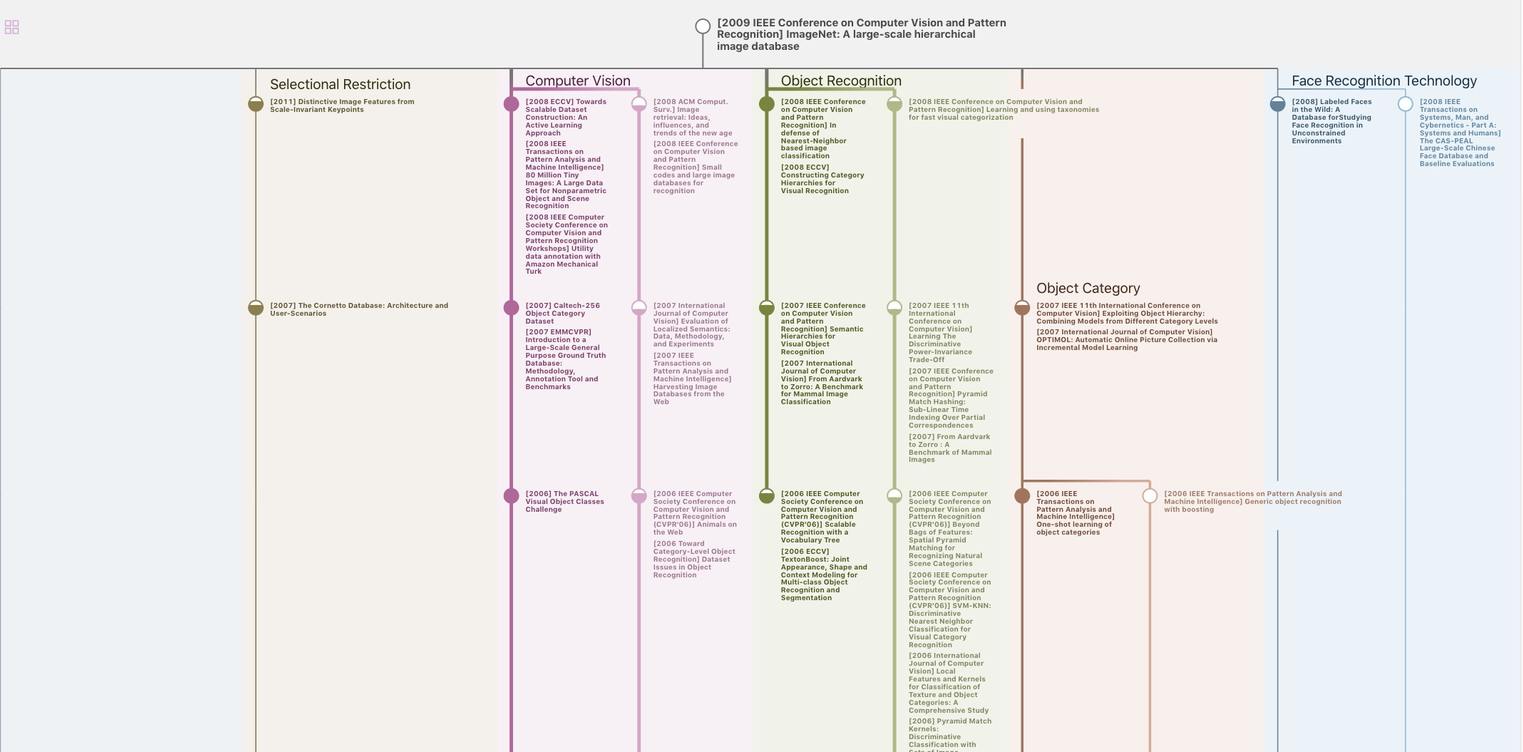
生成溯源树,研究论文发展脉络
Chat Paper
正在生成论文摘要