Estimation of Daily Arctic Winter Sea Ice Thickness from Thermodynamic Parameters Using a Self-Attention Convolutional Neural Network
Remote Sensing(2023)
摘要
Thermodynamic parameters play a crucial role in determining polar sea ice thickness (SIT); however, modeling their relationship is difficult due to the complexity of the influencing mechanisms. In this study, we propose a self-attention convolutional neural network (SAC-Net), which aims to model the relationship between thermodynamic parameters and SIT more parsimoniously, allowing us to estimate SIT directly from these parameters. SAC-Net uses a fully convolutional network as a baseline model to detect the spatial information of the thermodynamic parameters. Furthermore, a self-attention block is introduced to enhance the correlation among features. SAC-Net was trained on a dataset of SIT observations and thermodynamic data from the 2012-2019 freeze-up period, including surface upward sensible heat flux, surface upward latent heat flux, 2 m temperature, skin temperature, and surface snow temperature. The results show that our neural network model outperforms two thermodynamic-based SIT products in terms of accuracy and can provide reliable estimates of SIT. This study demonstrates the potential of the neural network to provide accurate and automated predictions of Arctic winter SIT from thermodynamic data, and, thus, the network can be used to support decision-making in certain fields, such as polar shipping, environmental protection, and climate science.
更多查看译文
关键词
sea ice thickness,thermodynamic parameters,neural network,Arctic
AI 理解论文
溯源树
样例
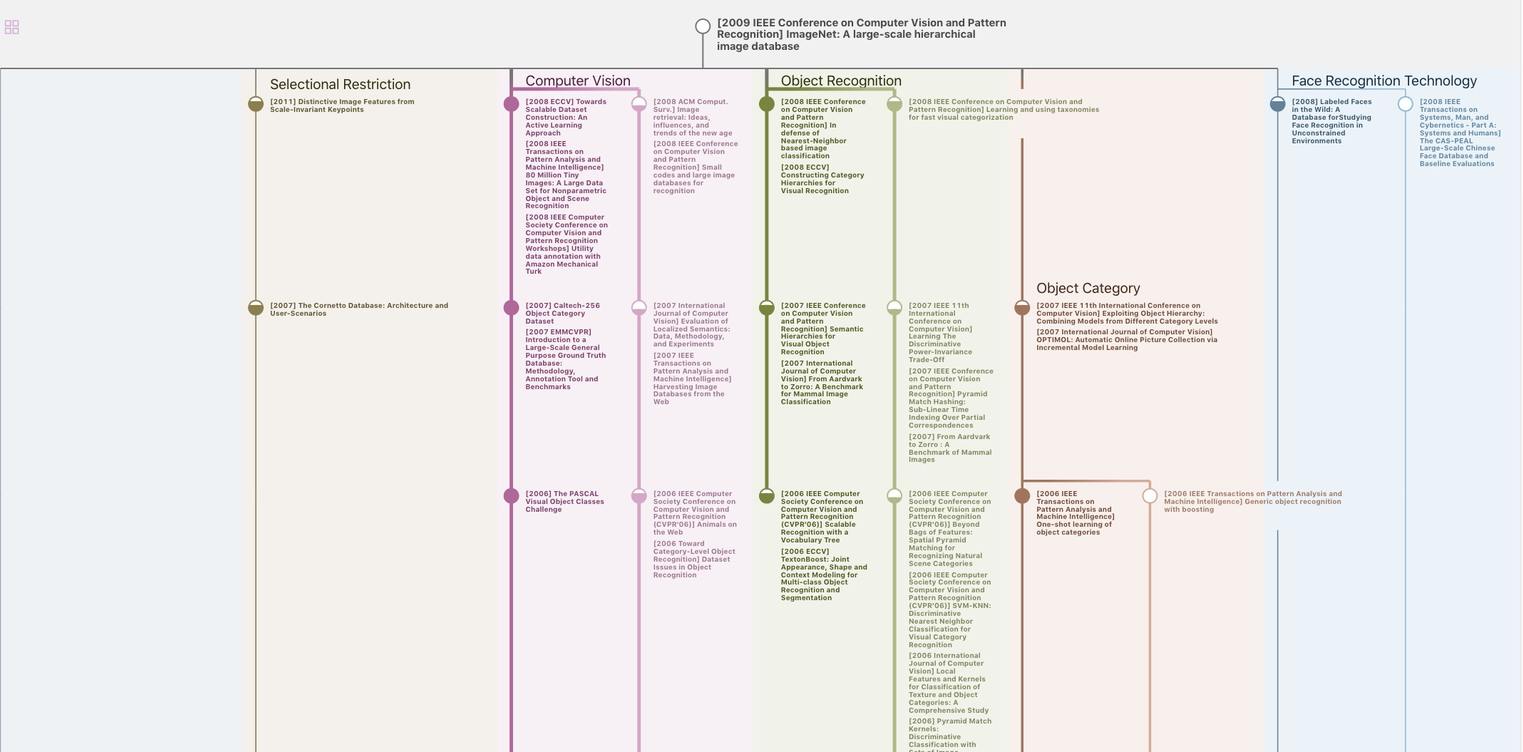
生成溯源树,研究论文发展脉络
Chat Paper
正在生成论文摘要