A Hybrid Residual Attention Convolutional Neural Network for Compressed Sensing Magnetic Resonance Image Reconstruction
Diagnostics(2023)
摘要
We propose a dual-domain deep learning technique for accelerating compressed sensing magnetic resonance image reconstruction. An advanced convolutional neural network with residual connectivity and an attention mechanism was developed for frequency and image domains. First, the sensor domain subnetwork estimates the unmeasured frequencies of k-space to reduce aliasing artifacts. Second, the image domain subnetwork performs a pixel-wise operation to remove blur and noisy artifacts. The skip connections efficiently concatenate the feature maps to alleviate the vanishing gradient problem. An attention gate in each decoder layer enhances network generalizability and speeds up image reconstruction by eliminating irrelevant activations. The proposed technique reconstructs real-valued clinical images from sparsely sampled k-spaces that are identical to the reference images. The performance of this novel approach was compared with state-of-the-art direct mapping, single-domain, and multi-domain methods. With acceleration factors (AFs) of 4 and 5, our method improved the mean peak signal-to-noise ratio (PSNR) to 8.67 and 9.23, respectively, compared with the single-domain Unet model; similarly, our approach increased the average PSNR to 3.72 and 4.61, respectively, compared with the multi-domain W-net. Remarkably, using an AF of 6, it enhanced the PSNR by 9.87 +/- 1.55 and 6.60 +/- 0.38 compared with Unet and W-net, respectively.
更多查看译文
关键词
MRI reconstruction,attention mechanism,compressed sensing,data augmentation,deep learning,dual residual CNN
AI 理解论文
溯源树
样例
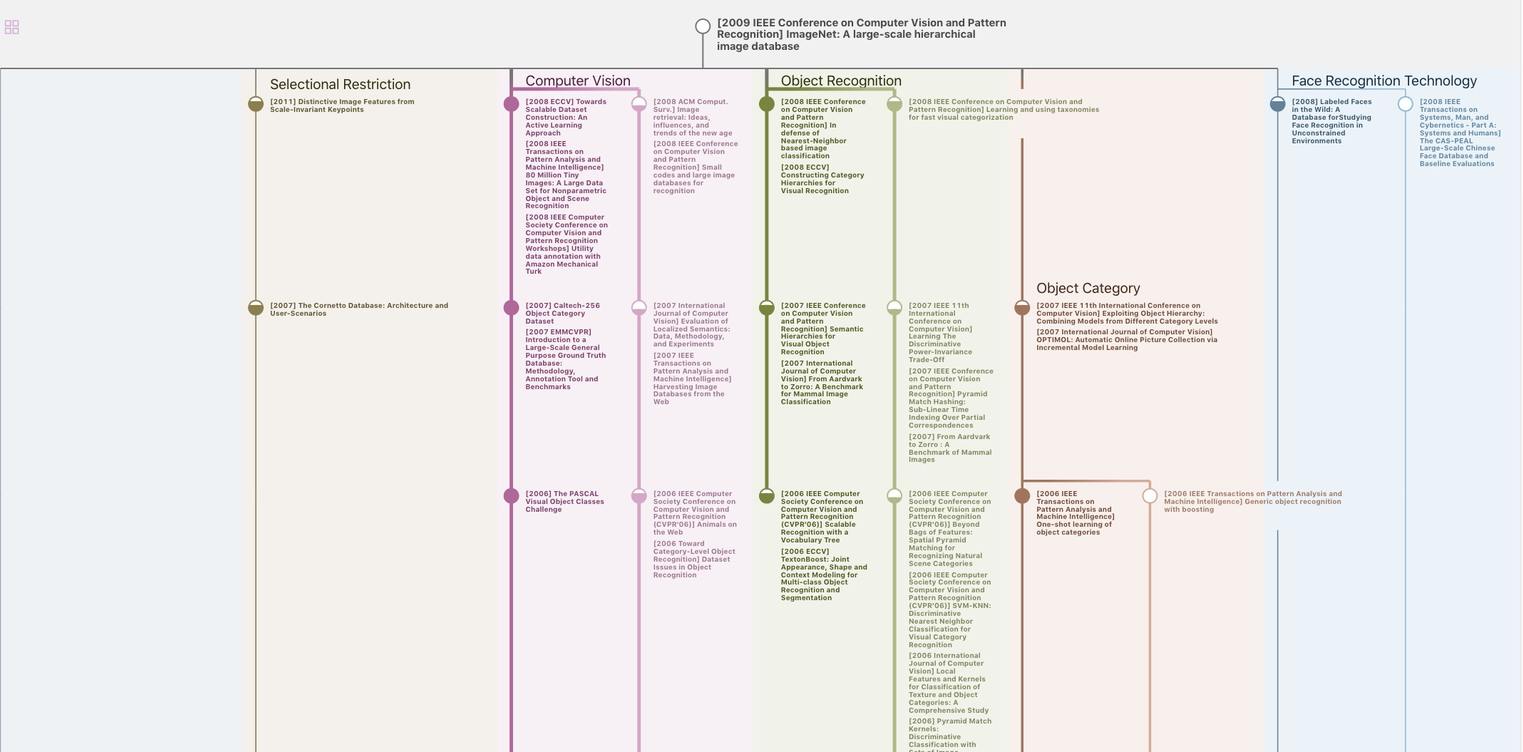
生成溯源树,研究论文发展脉络
Chat Paper
正在生成论文摘要