Automatic Kidney Segmentation Method Based on an Enhanced Generative Adversarial Network
Diagnostics(2023)
摘要
When deciding on a kidney tumor's diagnosis and treatment, it is critical to take its morphometry into account. It is challenging to undertake a quantitative analysis of the association between kidney tumor morphology and clinical outcomes due to a paucity of data and the need for the time-consuming manual measurement of imaging variables. To address this issue, an autonomous kidney segmentation technique, namely SegTGAN, is proposed in this paper, which is based on a conventional generative adversarial network model. Its core framework includes a discriminator network with multi-scale feature extraction and a fully convolutional generator network made up of densely linked blocks. For qualitative and quantitative comparisons with the SegTGAN technique, the widely used and related medical image segmentation networks U-Net, FCN, and SegAN are used. The experimental results show that the Dice similarity coefficient (DSC), volumetric overlap error (VOE), accuracy (ACC), and average surface distance (ASD) of SegTGAN on the Kits19 dataset reach 92.28%, 16.17%, 97.28%, and 0.61 mm, respectively. SegTGAN outscores all the other neural networks, which indicates that our proposed model has the potential to improve the accuracy of CT-based kidney segmentation.
更多查看译文
关键词
kidney segmentation,generative adversarial networks,dense block
AI 理解论文
溯源树
样例
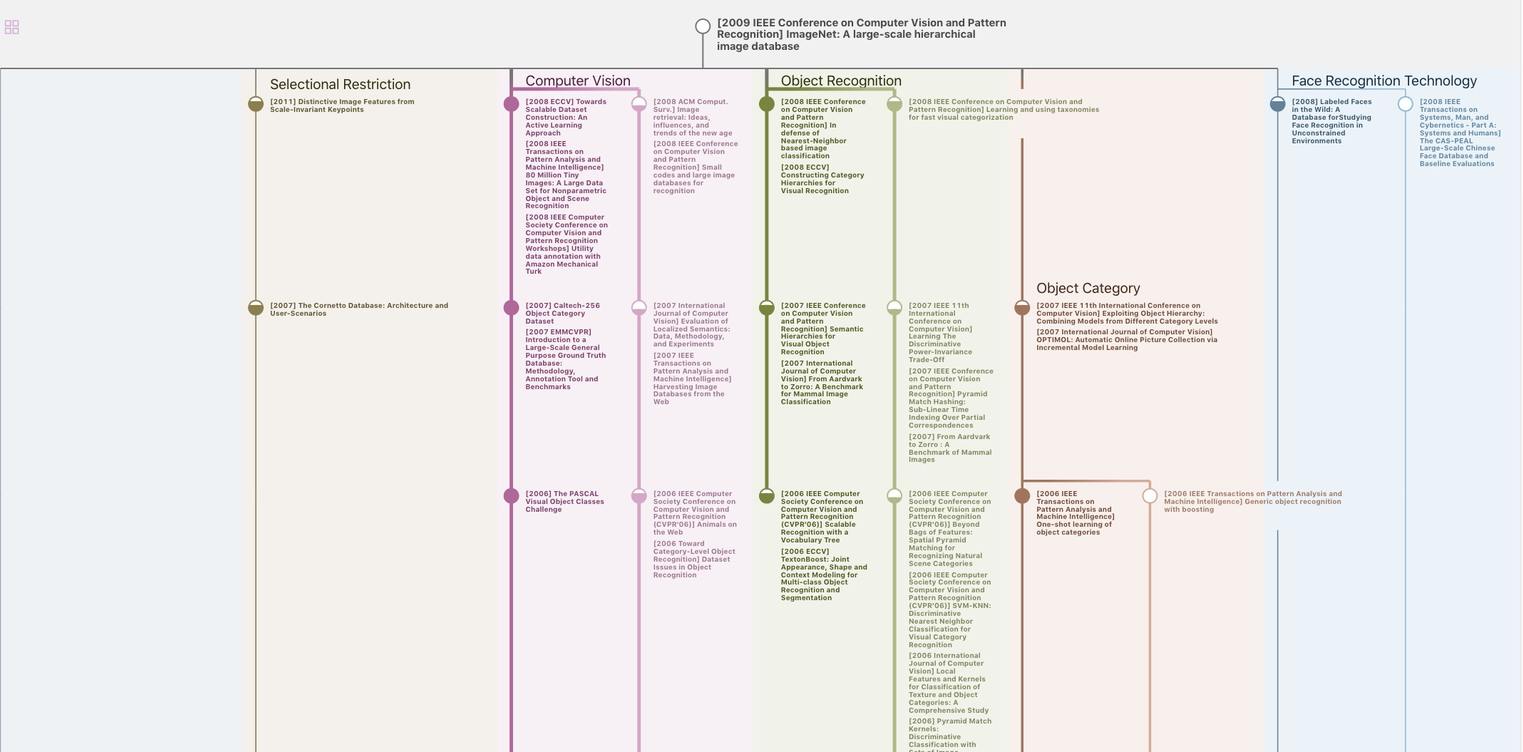
生成溯源树,研究论文发展脉络
Chat Paper
正在生成论文摘要