Unboxing Industry-Standard AI Models for Male Fertility Prediction with SHAP
Healthcare(2023)
摘要
Infertility is a social stigma for individuals, and male factors cause approximately 30% of infertility. Despite this, male infertility is underrecognized and underrepresented as a disease. According to the World Health Organization (WHO), changes in lifestyle and environmental factors are the prime reasons for the declining rate of male fertility. Artificial intelligence (AI)/machine learning (ML) models have become an effective solution for early fertility detection. Seven industry-standard ML models are used: support vector machine, random forest (RF), decision tree, logistic regression, naïve bayes, adaboost, and multi-layer perception to detect male fertility. Shapley additive explanations (SHAP) are vital tools that examine the feature’s impact on each model’s decision making. On these, we perform a comprehensive comparative study to identify good and poor classification models. While dealing with the all-above-mentioned models, the RF model achieves an optimal accuracy and area under curve (AUC) of 90.47% and 99.98%, respectively, by considering five-fold cross-validation (CV) with the balanced dataset. Furthermore, we provide the SHAP explanations of existing models that attain good and poor performance. The findings of this study show that decision making (based on ML models) with SHAP provides thorough explanations for detecting male fertility, as well as a reference for clinicians for further treatment planning.
更多查看译文
关键词
male infertility,oversampling,explainable artificial intelligence (XAI),Shapley additive explanations (SHAP)
AI 理解论文
溯源树
样例
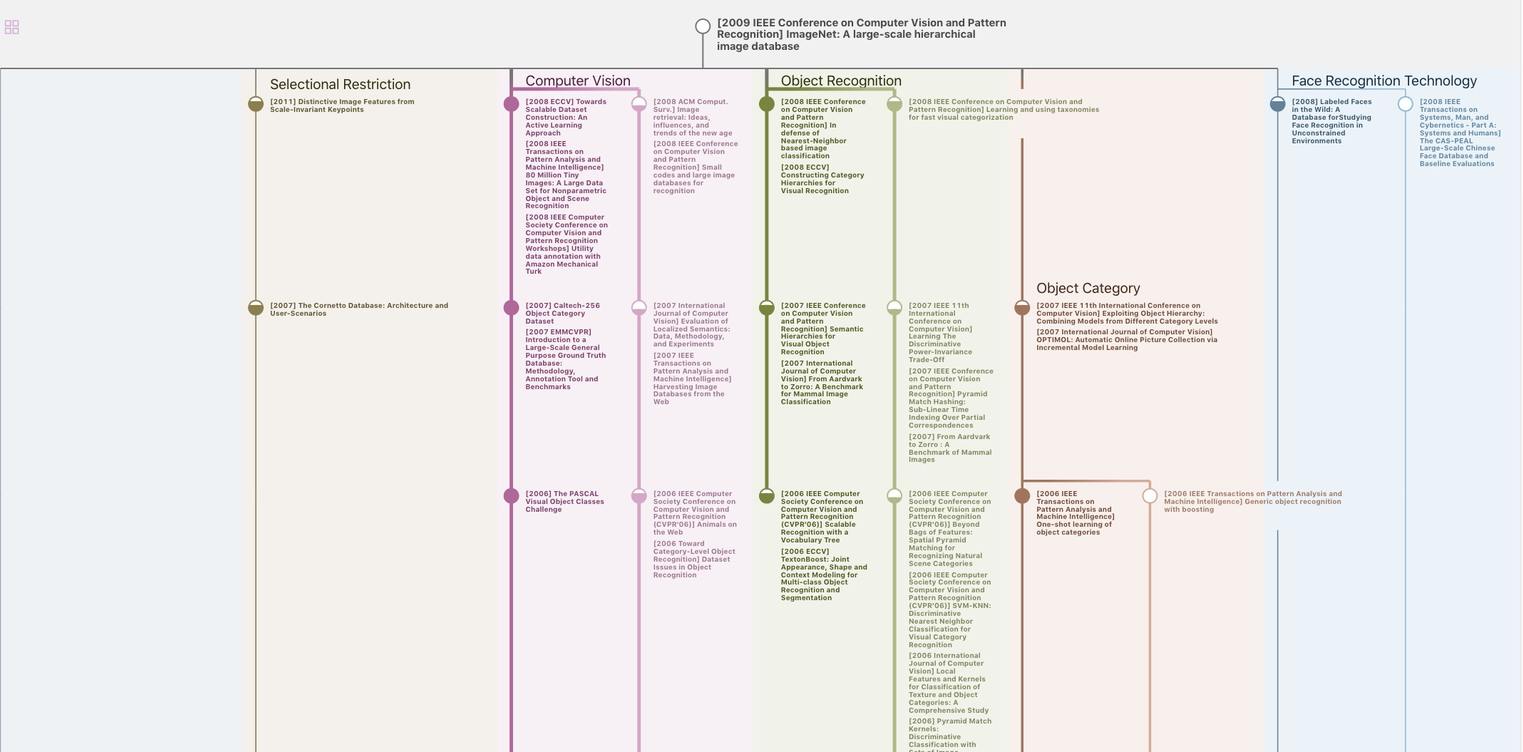
生成溯源树,研究论文发展脉络
Chat Paper
正在生成论文摘要