A deep learning and docking simulation-based virtual screening strategy enables the rapid identification of HIF-1 pathway activators from a marine natural product database
JOURNAL OF BIOMOLECULAR STRUCTURE & DYNAMICS(2024)
摘要
Artificial Intelligence is hailed as a cutting-edge technology for accelerating drug discovery efforts, and our goal was to validate its potential in predicting pharmacological inhibitors of EGLN1 using a deep learning-based architecture, one of its subsidiaries. Egl nine homolog 1 (EGLN1) inhibition prevents poly ubiquitination-mediated proteosomal destruction HIF-1a. The pharmacological interventions aimed at stabilizing HIF-1a have the potential to be a promising treatment option for a range of human diseases, including ischemic stroke. To unveil a novel EGLN1 inhibitor from marine natural products, a custom-based virtual screening was carried out using a Deep Convolutional Neural Network (DCNN) architecture, docking, and molecular dynamics simulation. The custom DCNN model was optimized and further employed to screen marine natural products from the CMNPD database. The docking was performed as a secondary strategy for screened hits. Molecular dynamics (MD) and molecular mechanics/generalized Born surface area (MM-GBSA) were used to analyze inhibitor binding and identify key interactions. The findings support the claim that deep learning-based virtual screening is a rapid, reliable and accurate method of identifying highly contributing drug candidates (EGLN1 inhibitors). This study demonstrates that deep learning architecture can significantly accelerate drug discovery and development, and provides a solid foundation for using (Z)-2-ethylhex-2-enedioic acid [(Z)-2-ethylhex-2-enedioic acid] as a potential EGLN1 inhibitor for treating various health complications.
更多查看译文
关键词
Deep convolutional neural network,EGLN1,docking,molecular dynamics simulation,hypoxia-inducible factor 1,ADME,toxicity,marine natural products
AI 理解论文
溯源树
样例
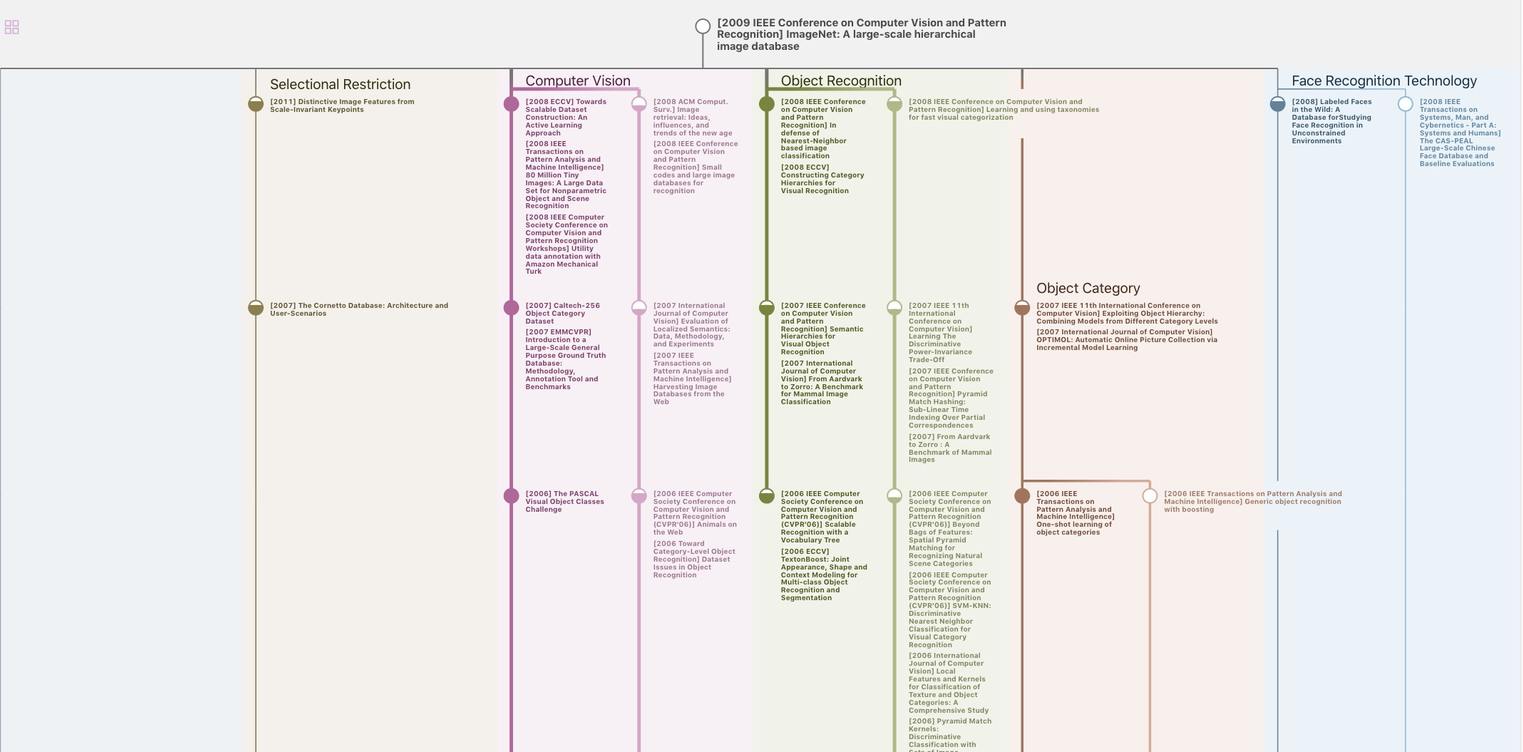
生成溯源树,研究论文发展脉络
Chat Paper
正在生成论文摘要