IDNet: Information Decomposition Network for Fast Panoptic Segmentation
IEEE TRANSACTIONS ON IMAGE PROCESSING(2024)
摘要
Traditional CNN -based pipelines for panoptic segmentation decompose the task into two subtasks, i.e., instance segmentation and semantic segmentation. In this way, they extract information with multiple branches, perform two subtasks separately and finally fuse the results. However, excessive feature extraction and complicated processes make them timeconsuming. We propose IDNet to decompose panoptic segmentation at information level. IDNet only extracts two kinds of information and directly completes panoptic segmentation task, saving the efforts to extract extra information and to fuse subtasks. By decomposing panoptic segmentation into category information and location information and recomposing them with a serial pipeline, the process for panoptic segmentation is simplified greatly and unified with regard to stuff and things. We also adopt two correction losses specially designed for our serial pipeline, guaranteeing the overall predicting performance. As a result, IDNet strikes a better balance between effectiveness and efficiency, achieving the fastest inference speed of 24.2 FPS at a resolution of 800 x 1333 on a Tesla V100 GPU and a PQ of 43.8, which is comparable in one -stage CNN -based methods. The code will be released at https://github.com/AronLin/IDNet.
更多查看译文
关键词
Pipelines,Task analysis,Data mining,Feature extraction,Head,Semantic segmentation,Symbols,Scene parsing,panoptic segmentation
AI 理解论文
溯源树
样例
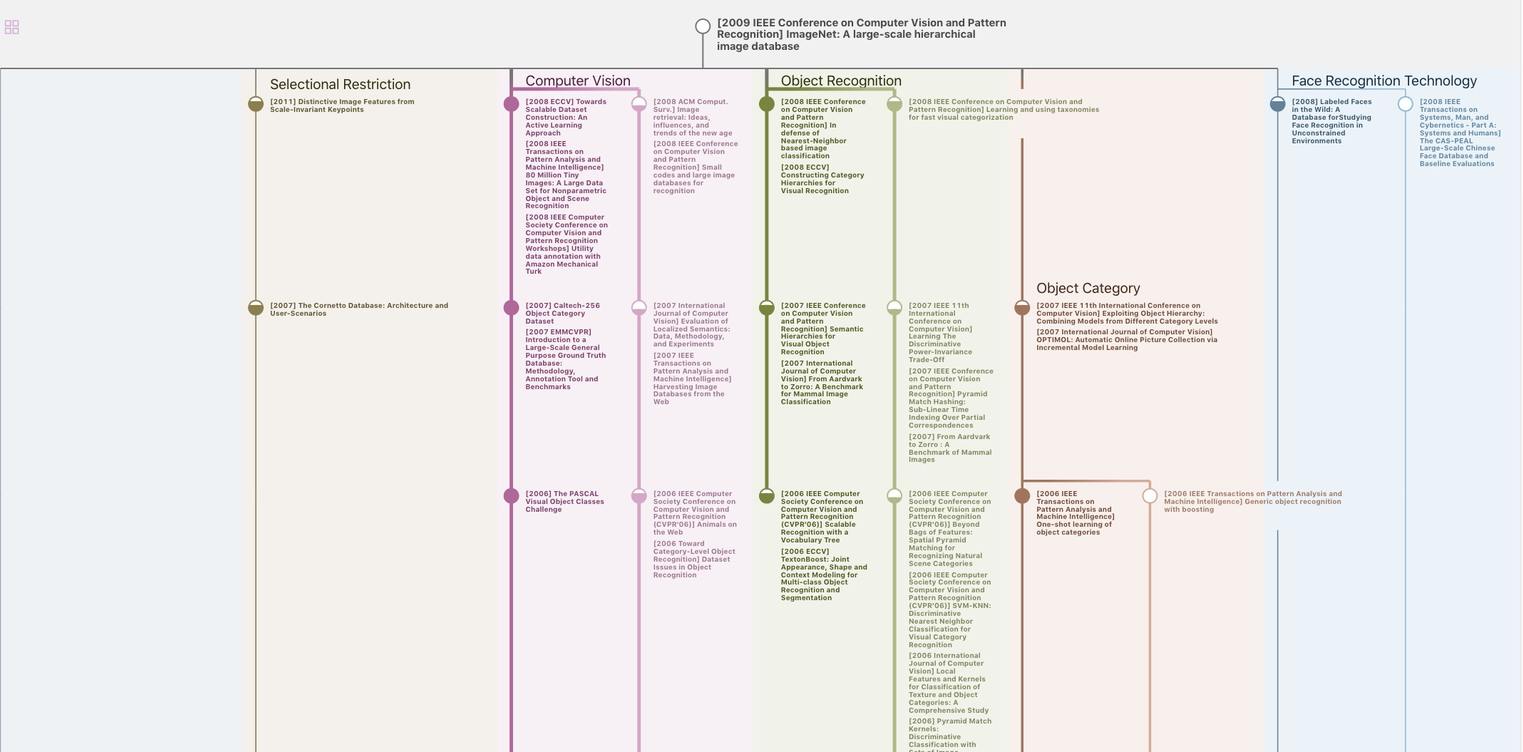
生成溯源树,研究论文发展脉络
Chat Paper
正在生成论文摘要