Equivariant Graph Neural Networks for Charged Particle Tracking
CoRR(2023)
摘要
Graph neural networks (GNNs) have gained traction in high-energy physics (HEP) for their potential to improve accuracy and scalability. However, their resource-intensive nature and complex operations have motivated the development of symmetry-equivariant architectures. In this work, we introduce EuclidNet, a novel symmetry-equivariant GNN for charged particle tracking. EuclidNet leverages the graph representation of collision events and enforces rotational symmetry with respect to the detector's beamline axis, leading to a more efficient model. We benchmark EuclidNet against the state-of-the-art Interaction Network on the TrackML dataset, which simulates high-pileup conditions expected at the High-Luminosity Large Hadron Collider (HL-LHC). Our results show that EuclidNet achieves near-state-of-the-art performance at small model scales (<1000 parameters), outperforming the non-equivariant benchmarks. This study paves the way for future investigations into more resource-efficient GNN models for particle tracking in HEP experiments.
更多查看译文
关键词
equivariant graph neural networks,tracking,particle,neural networks
AI 理解论文
溯源树
样例
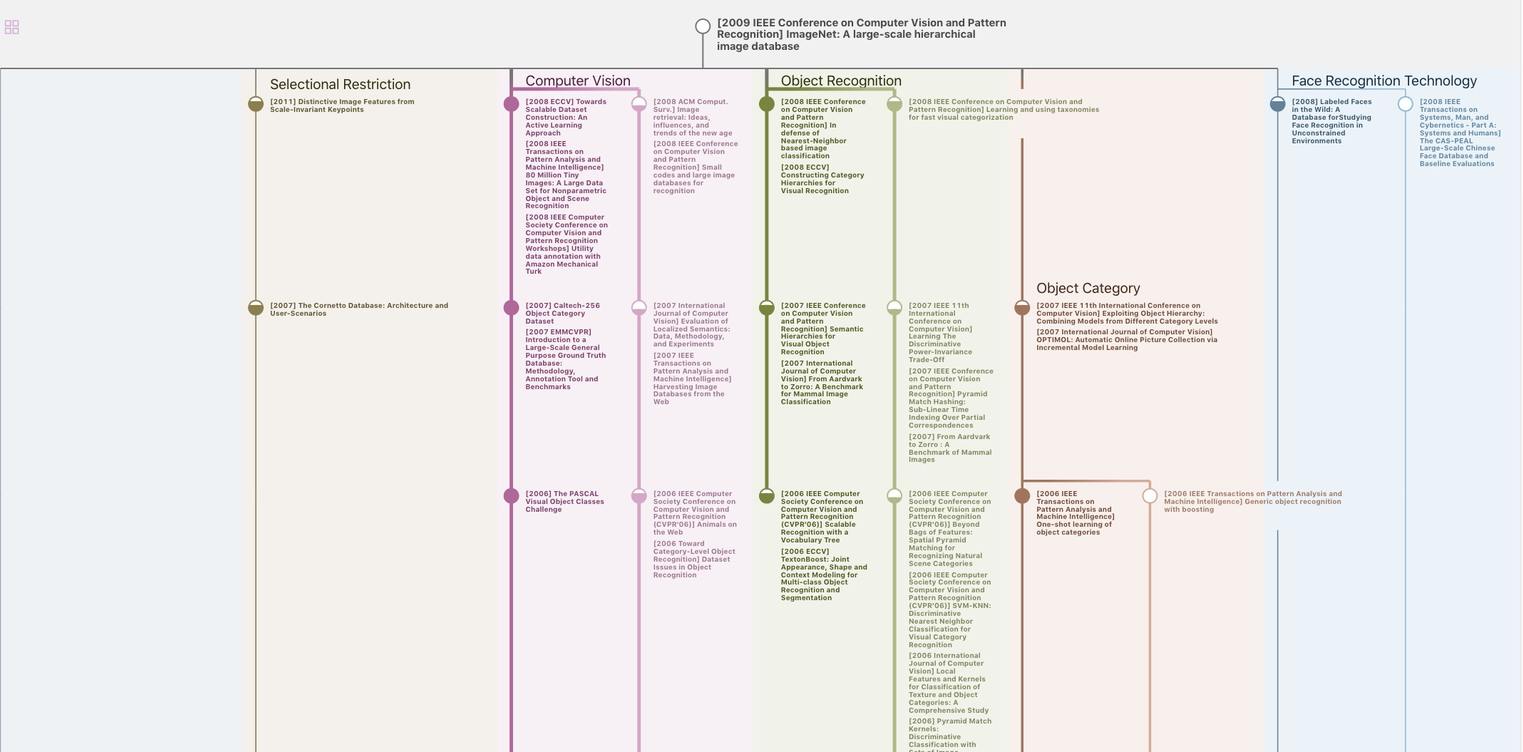
生成溯源树,研究论文发展脉络
Chat Paper
正在生成论文摘要