MC-ViViT: Multi-branch Classifier-ViViT to detect Mild Cognitive Impairment in older adults using facial videos
EXPERT SYSTEMS WITH APPLICATIONS(2024)
摘要
Deep machine learning models including Convolutional Neural Networks (CNN) have been successful in the detection of Mild Cognitive Impairment (MCI) using medical images, questionnaires, and videos. This paper proposes a novel Multi-branch Classifier-Video Vision Transformer (MC-ViViT) model to distinguish MCI from those with normal cognition by analyzing facial features. The data comes from the I-CONECT, a behavioral intervention trial aimed at improving cognitive function by providing frequent video chats. MC-ViViT extracts spatiotemporal features of videos in one branch and augments representations by the MC module. The I-CONECT dataset is challenging as the dataset is imbalanced containing Hard-Easy and Positive-Negative samples, which impedes the performance of MC-ViViT. We propose a loss function for Hard-Easy and PositiveNegative Samples (HP Loss) by combining Focal loss and AD-CORRE loss to address the imbalanced problem. Our experimental results on the I-CONECT dataset show the great potential of MC-ViViT in predicting MCI with a high accuracy of 90.63% accuracy on some of the interview videos.
更多查看译文
关键词
Deep learning,Facial expression features,Inter-and intra-class imbalance,Mild Cognitive Impairment,Multi-branch Classifier,Transformer,ViViT
AI 理解论文
溯源树
样例
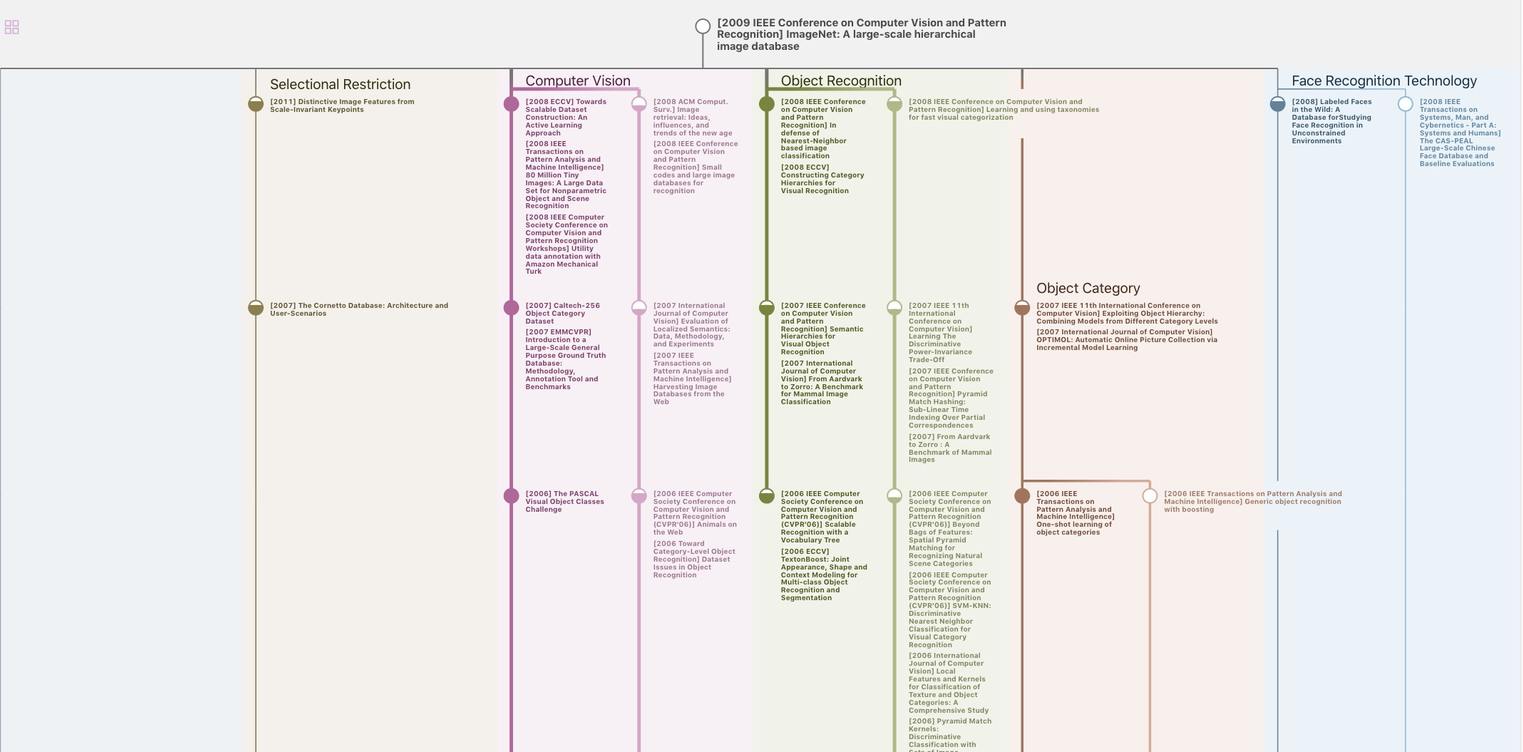
生成溯源树,研究论文发展脉络
Chat Paper
正在生成论文摘要