BanditQ -- No-Regret Learning with Guaranteed Per-User Rewards in Adversarial Environments
CoRR(2023)
摘要
Classic online prediction algorithms, such as Hedge, are inherently unfair by design, as they try to play the most rewarding arm as many times as possible while ignoring the sub-optimal arms to achieve sublinear regret. In this paper, we consider a fair online prediction problem in the adversarial setting with hard lower bounds on the rate of accrual of rewards for all arms. By combining elementary queueing theory with online learning, we propose a new online prediction policy, called BanditQ, that achieves the target rate constraints while achieving a regret of $O(T^{3/4})$ in the full-information setting. The design and analysis of BanditQ involve a novel use of the potential function method and are of independent interest.
更多查看译文
AI 理解论文
溯源树
样例
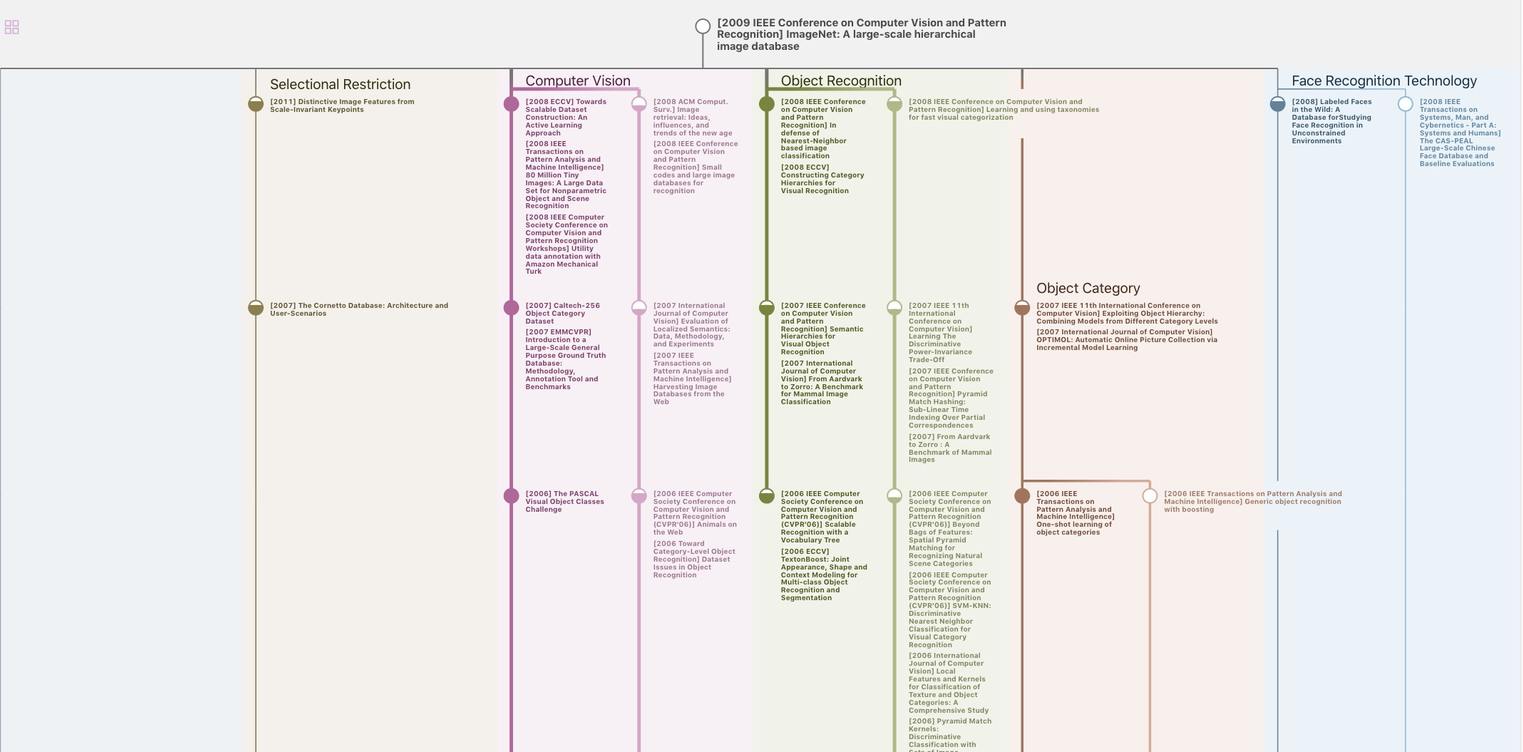
生成溯源树,研究论文发展脉络
Chat Paper
正在生成论文摘要