An Efficient Deep Learning Image Condition for Locating Earthquakes
arXiv (Cornell University)(2023)
摘要
Migration-based earthquake location methods may encounter the polarity reversal issue in the observed waveforms due to the non-explosive components of the seismic source, leading to an unfocused migration image. To address this issue, here we propose a novel Deep Learning Image Condition (DLIC). The proposed DLIC follows the geophysical principle that the best-aligned gathers utterly correspond to a best-imaged source location. The DLIC takes advantage of the general pattern recognition ability of the convolutional neural network and is demonstrated to be very effective in solving the polarity reversal issue as well as enhancing the focusing of migrated images. Both a synthetic test and real data application are used to validate the effectiveness and benefit of the DLIC neural network. By applying to a real data set of 212 earthquakes in southern California, we find that the DLIC can predict deep-learning images that show much better energy concentration than the popular source scanning algorithm. Further tests show that the DLIC is generally applicable to continuous seismic data, to regions with few historical earthquakes, and has the potential to locate small earthquakes. The proposed DLIC shall benefit the migration-based source location methods.
更多查看译文
关键词
deep learning,image
AI 理解论文
溯源树
样例
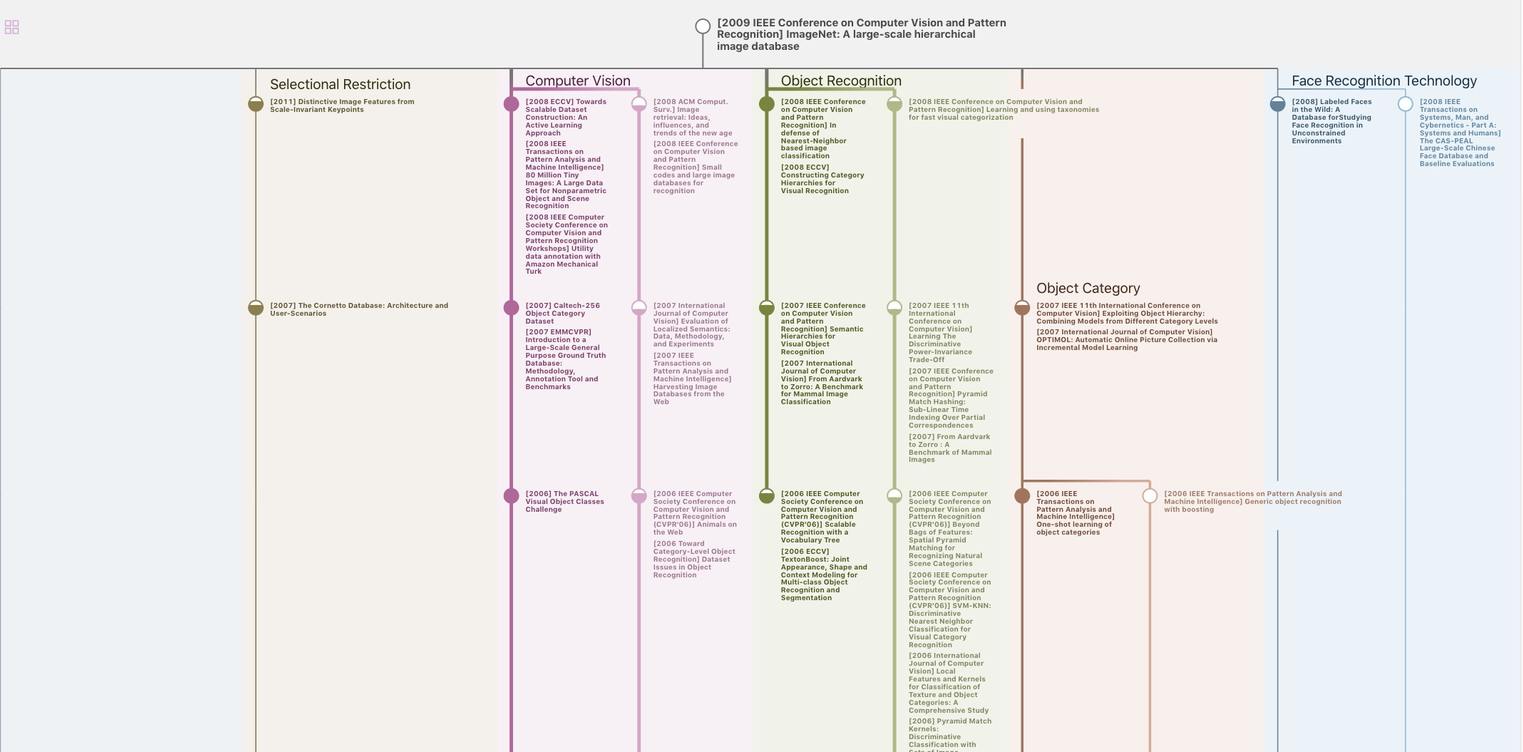
生成溯源树,研究论文发展脉络
Chat Paper
正在生成论文摘要