Revisiting Test Time Adaptation under Online Evaluation
CoRR(2023)
摘要
This paper proposes a novel online evaluation protocol for Test Time Adaptation (TTA) methods, which penalizes slower methods by providing them with fewer samples for adaptation. TTA methods leverage unlabeled data at test time to adapt to distribution shifts. Though many effective methods have been proposed, their impressive performance usually comes at the cost of significantly increased computation budgets. Current evaluation protocols overlook the effect of this extra computation cost, affecting their real-world applicability. To address this issue, we propose a more realistic evaluation protocol for TTA methods, where data is received in an online fashion from a constant-speed data stream, thereby accounting for the method's adaptation speed. We apply our proposed protocol to benchmark several TTA methods on multiple datasets and scenarios. Extensive experiments shows that, when accounting for inference speed, simple and fast approaches can outperform more sophisticated but slower methods. For example, SHOT from 2020 outperforms the state-of-the-art method SAR from 2023 under our online setting. Our online evaluation protocol emphasizes the need for developing TTA methods that are efficient and applicable in realistic settings.
更多查看译文
关键词
test time adaptation,evaluation,online
AI 理解论文
溯源树
样例
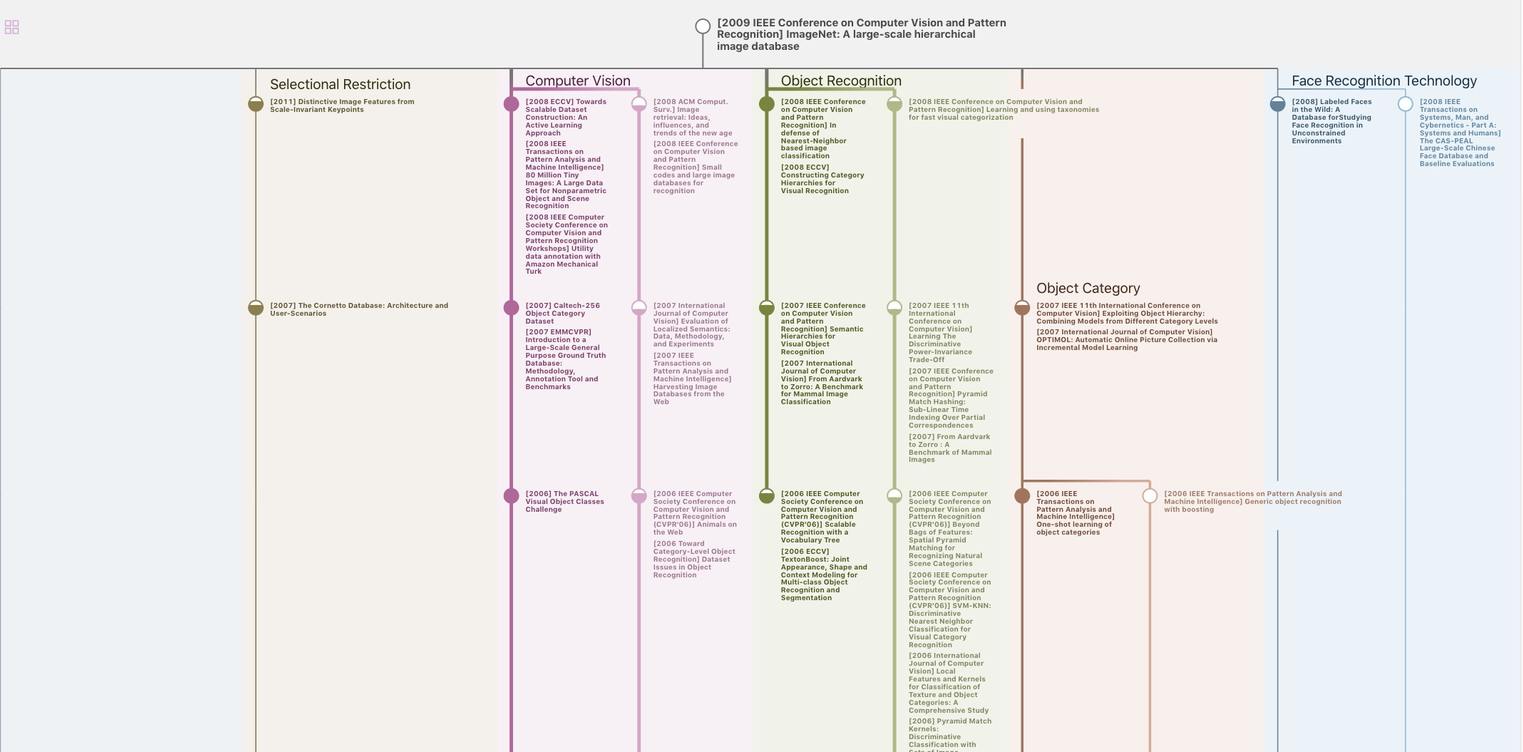
生成溯源树,研究论文发展脉络
Chat Paper
正在生成论文摘要