Masking important information to assess the robustness of a multimodal classifier for emotion recognition.
Frontiers in artificial intelligence(2023)
摘要
Deep neural networks have been proven effective in classifying human interactions into emotions, especially by encoding multiple input modalities. In this work, we assess the robustness of a transformer-based multimodal audio-text classifier for emotion recognition, by perturbing the input at inference time using attacks which we design specifically to corrupt information deemed important for emotion recognition. To measure the impact of the attacks on the classifier, we compare between the accuracy of the classifier on the perturbed input and on the original, unperturbed input. Our results show that the multimodal classifier is more resilient to perturbation attacks than the equivalent unimodal classifiers, suggesting that the two modalities are encoded in a way that allows the classifier to benefit from one modality even when the other one is slightly damaged.
更多查看译文
关键词
NLP,emotion-recognition,multimodal,perturbation,text-audio
AI 理解论文
溯源树
样例
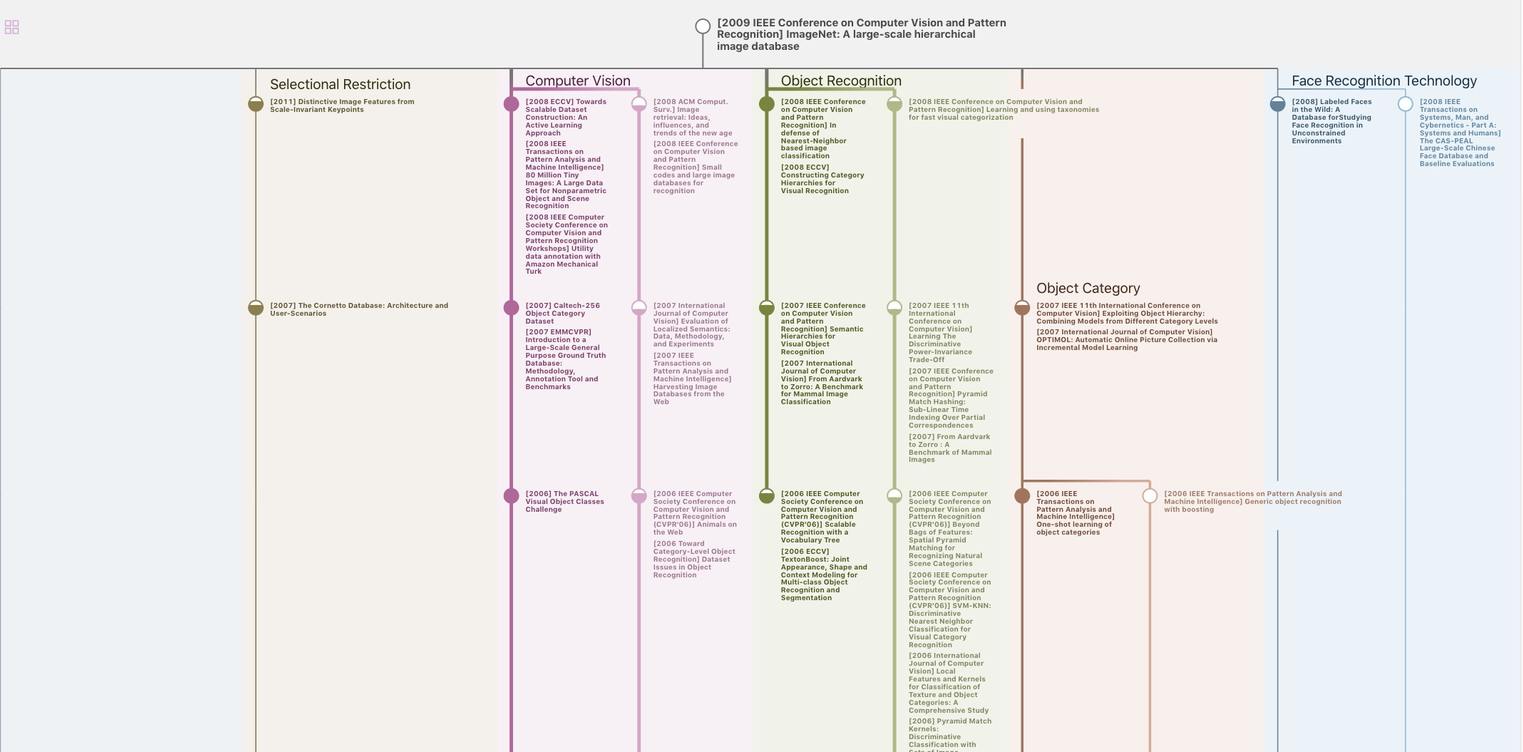
生成溯源树,研究论文发展脉络
Chat Paper
正在生成论文摘要