Learning a Universal Human Prior for Dexterous Manipulation from Human Preference
CoRR(2023)
摘要
Generating human-like behavior on robots is a great challenge especially in dexterous manipulation tasks with robotic hands. Even in simulation with no sample constraints, scripting controllers is intractable due to high degrees of freedom, and manual reward engineering can also be hard and lead to non-realistic motions. Leveraging the recent progress on Reinforcement Learning from Human Feedback (RLHF), we propose a framework to learn a universal human prior using direct human preference feedback over videos, for efficiently tuning the RL policy on 20 dual-hand robot manipulation tasks in simulation, without a single human demonstration. One task-agnostic reward model is trained through iteratively generating diverse polices and collecting human preference over the trajectories; it is then applied for regularizing the behavior of polices in the fine-tuning stage. Our method empirically demonstrates more human-like behaviors on robot hands in diverse tasks including even unseen tasks, indicating its generalization capability.
更多查看译文
关键词
universal human prior,dexterous manipulation,learning,preference
AI 理解论文
溯源树
样例
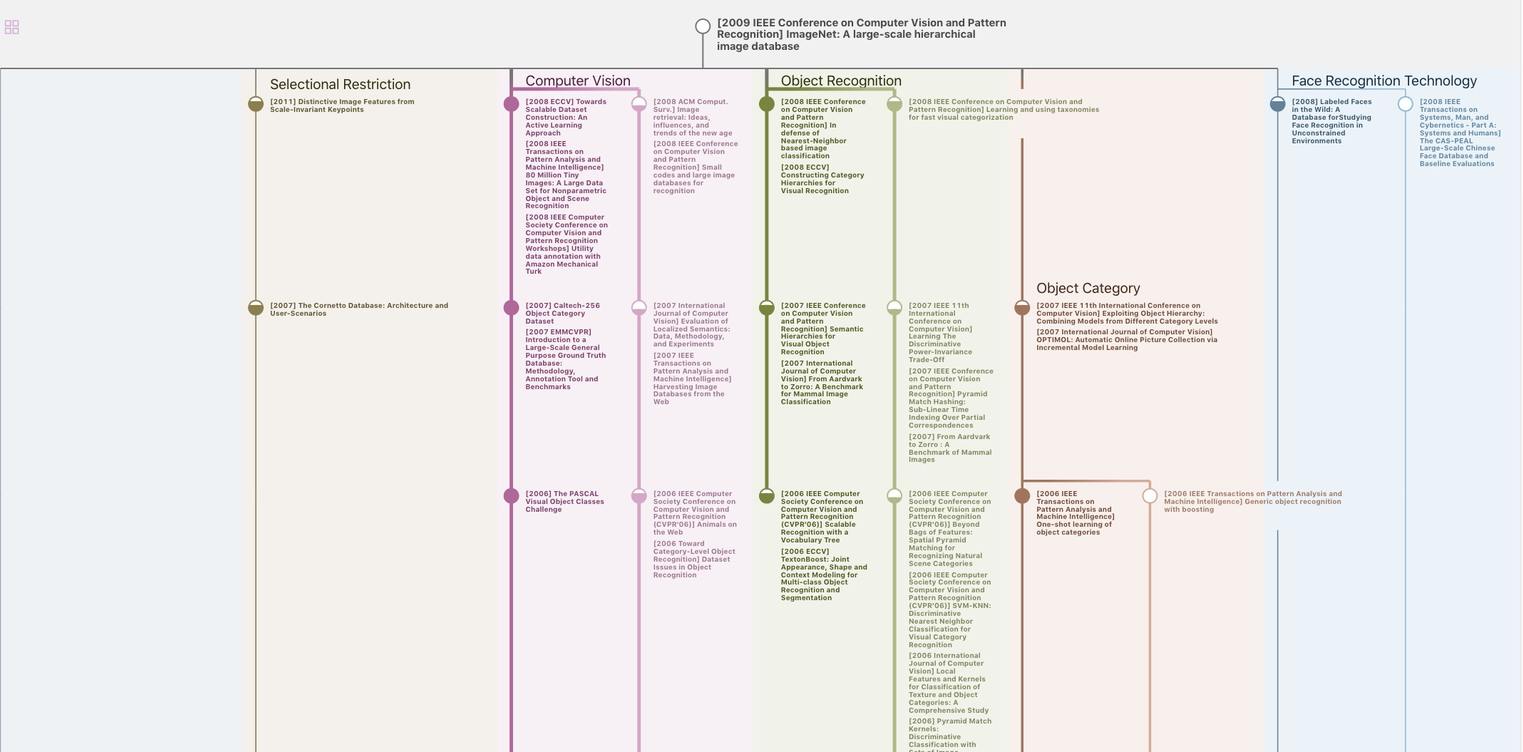
生成溯源树,研究论文发展脉络
Chat Paper
正在生成论文摘要