Deep Active Alignment of Knowledge Graph Entities and Schemata
CoRR(2023)
摘要
Knowledge graphs (KGs) store rich facts about the real world. In this paper, we study KG alignment, which aims to find alignment between not only entities but also relations and classes in different KGs. Alignment at the entity level can cross-fertilize alignment at the schema level. We propose a new KG alignment approach, called DAAKG, based on deep learning and active learning. With deep learning, it learns the embeddings of entities, relations and classes, and jointly aligns them in a semi-supervised manner. With active learning, it estimates how likely an entity, relation or class pair can be inferred, and selects the best batch for human labeling. We design two approximation algorithms for efficient solution to batch selection. Our experiments on benchmark datasets show the superior accuracy and generalization of DAAKG and validate the effectiveness of all its modules.
更多查看译文
关键词
knowledge graph entities,active
AI 理解论文
溯源树
样例
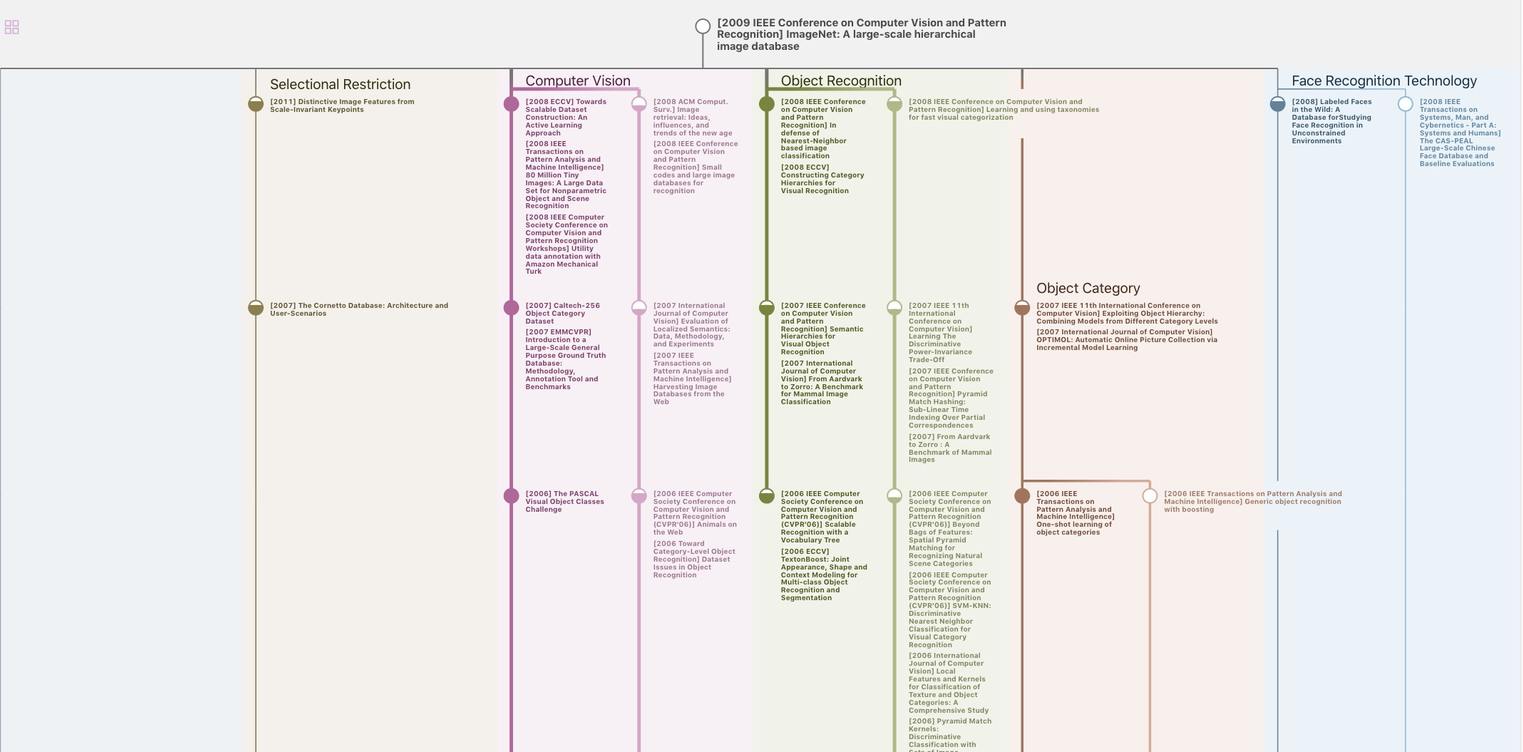
生成溯源树,研究论文发展脉络
Chat Paper
正在生成论文摘要