An adaptive sampling sequential quadratic programming method for nonsmooth stochastic optimization with upper-$\mathcal{C}^2$ objective
arxiv(2023)
摘要
We propose an optimization algorithm that incorporates adaptive sampling for stochastic nonsmooth nonconvex optimization problems with upper-$\mathcal{C}^2$ objective functions. Upper-$\mathcal{C}^2$ is a weakly concave property that exists naturally in many applications, particularly certain classes of solutions to parametric optimization problems, e.g., recourse of stochastic programming and projection into closed sets. Our algorithm is a stochastic sequential quadratic programming (SQP) method extended to nonsmooth problems with upper$\mathcal{C}^2$ objectives and is globally convergent in expectation with bounded algorithmic parameters. The capabilities of our algorithm are demonstrated by solving a joint production, pricing and shipment problem, as well as a realistic optimal power flow problem as used in current power grid industry practice.
更多查看译文
AI 理解论文
溯源树
样例
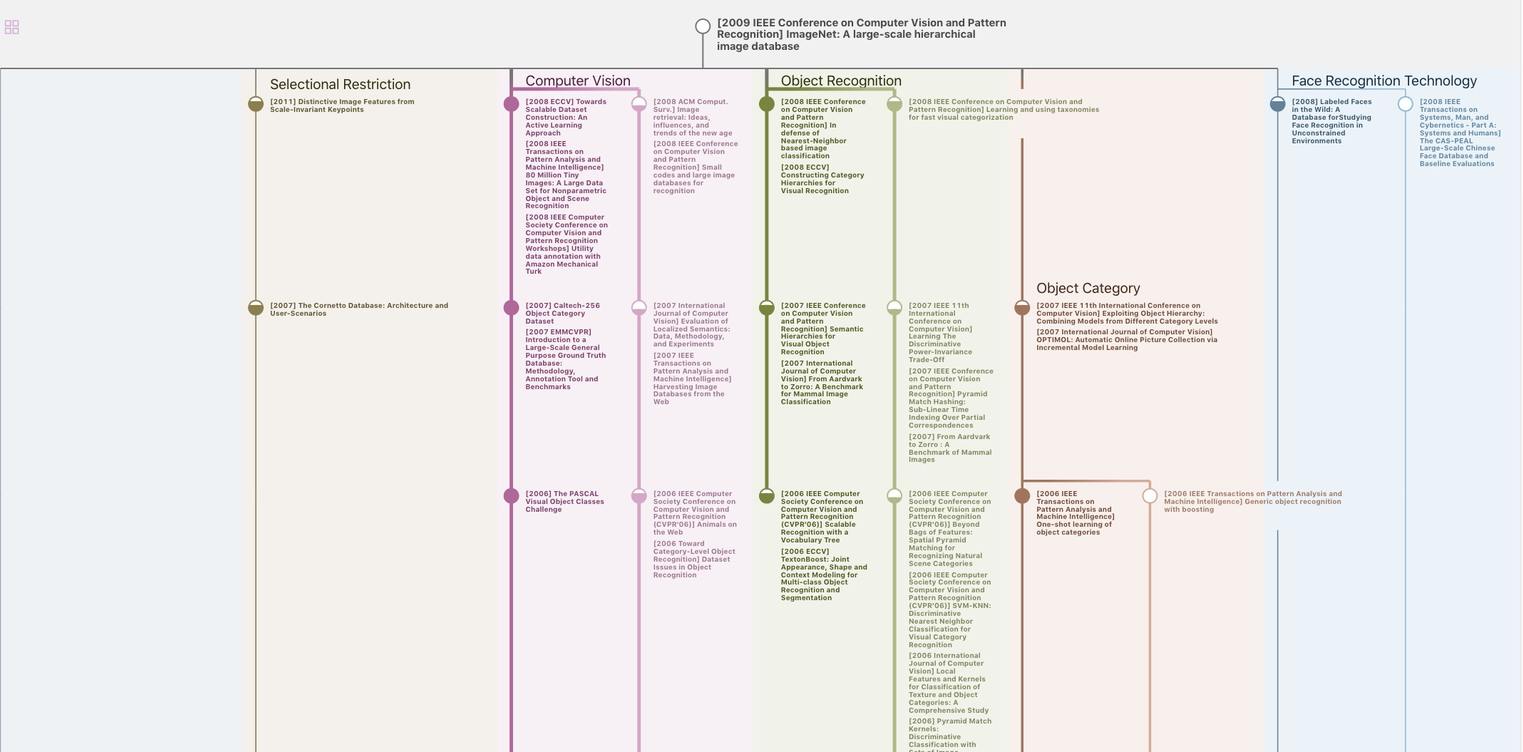
生成溯源树,研究论文发展脉络
Chat Paper
正在生成论文摘要