CLVOS23: A Long Video Object Segmentation Dataset for Continual Learning
CoRR(2023)
摘要
Continual learning in real-world scenarios is a major challenge. A general continual learning model should have a constant memory size and no predefined task boundaries, as is the case in semi-supervised Video Object Segmentation (VOS), where continual learning challenges particularly present themselves in working on long video sequences. In this article, we first formulate the problem of semi-supervised VOS, specifically online VOS, as a continual learning problem, and then secondly provide a public VOS dataset, CLVOS23, focusing on continual learning. Finally, we propose and implement a regularization-based continual learning approach on LWL, an existing online VOS baseline, to demonstrate the efficacy of continual learning when applied to online VOS and to establish a CLVOS23 baseline. We apply the proposed baseline to the Long Videos dataset as well as to two short video VOS datasets, DAVIS16 and DAVIS17. To the best of our knowledge, this is the first time that VOS has been defined and addressed as a continual learning problem.
更多查看译文
关键词
CLVOS23 dataset,continual learning,DAVIS16 dataset,DAVIS17 dataset,long video object segmentation dataset,long video sequences,regularization-based continual learning,semisupervised video object segmentation,semisupervised VOS
AI 理解论文
溯源树
样例
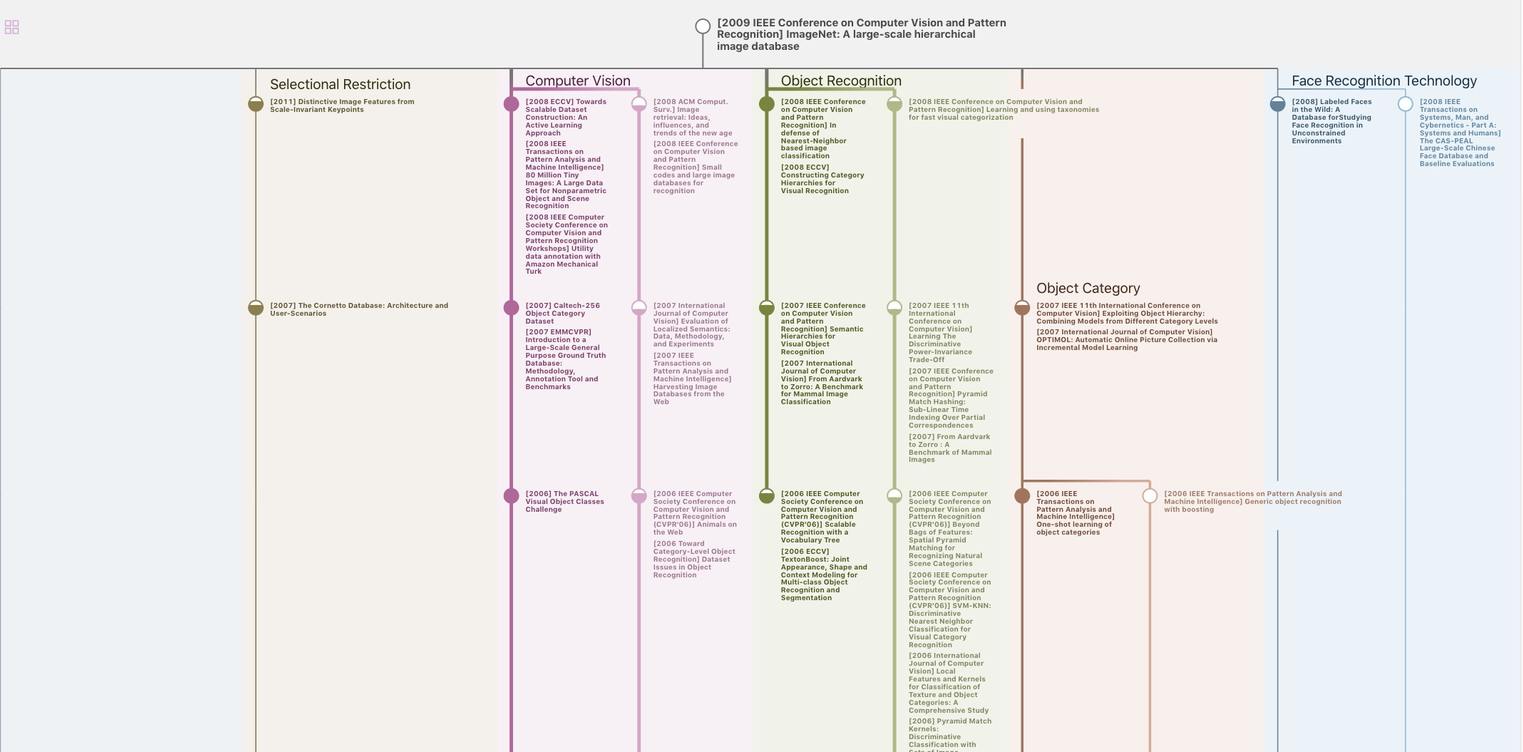
生成溯源树,研究论文发展脉络
Chat Paper
正在生成论文摘要