Kernel Selection for Gaussian Process in Cosmology: with Approximate Bayesian Computation Rejection and Nested Sampling
ASTROPHYSICAL JOURNAL SUPPLEMENT SERIES(2023)
摘要
Gaussian Process (GP) has gained much attention in cosmology due to its ability to reconstruct cosmological data in a model-independent manner. In this study, we compare two methods for GP kernel selection: Approximate Bayesian Computation (ABC) Rejection and nested sampling. We analyze three types of data: cosmic Chronometer data (CC), Type Ia Supernovae (SNIa), and Gamma Ray Burst (GRB), using five kernel functions. To evaluate the differences between kernel functions, we assess the strength of evidence using Bayes factors. Our results show that, for ABC Rejection, the Mat\'ern kernel with $\nu$=5/2 (M52 kernel) outperformes the commonly used Radial Basis Function (RBF) kernel in approximating all three datasets. Bayes factors indicate that the M52 kernel typically supports the observed data better than the RBF kernel, but with no clear advantage over other alternatives. However, nested sampling gives different results, with the M52 kernel losing its advantage. Nevertheless, Bayes factors indicate no significant dependence of the data on each kernel.
更多查看译文
关键词
gaussian process,bayesian computation rejection,cosmology,nested sampling
AI 理解论文
溯源树
样例
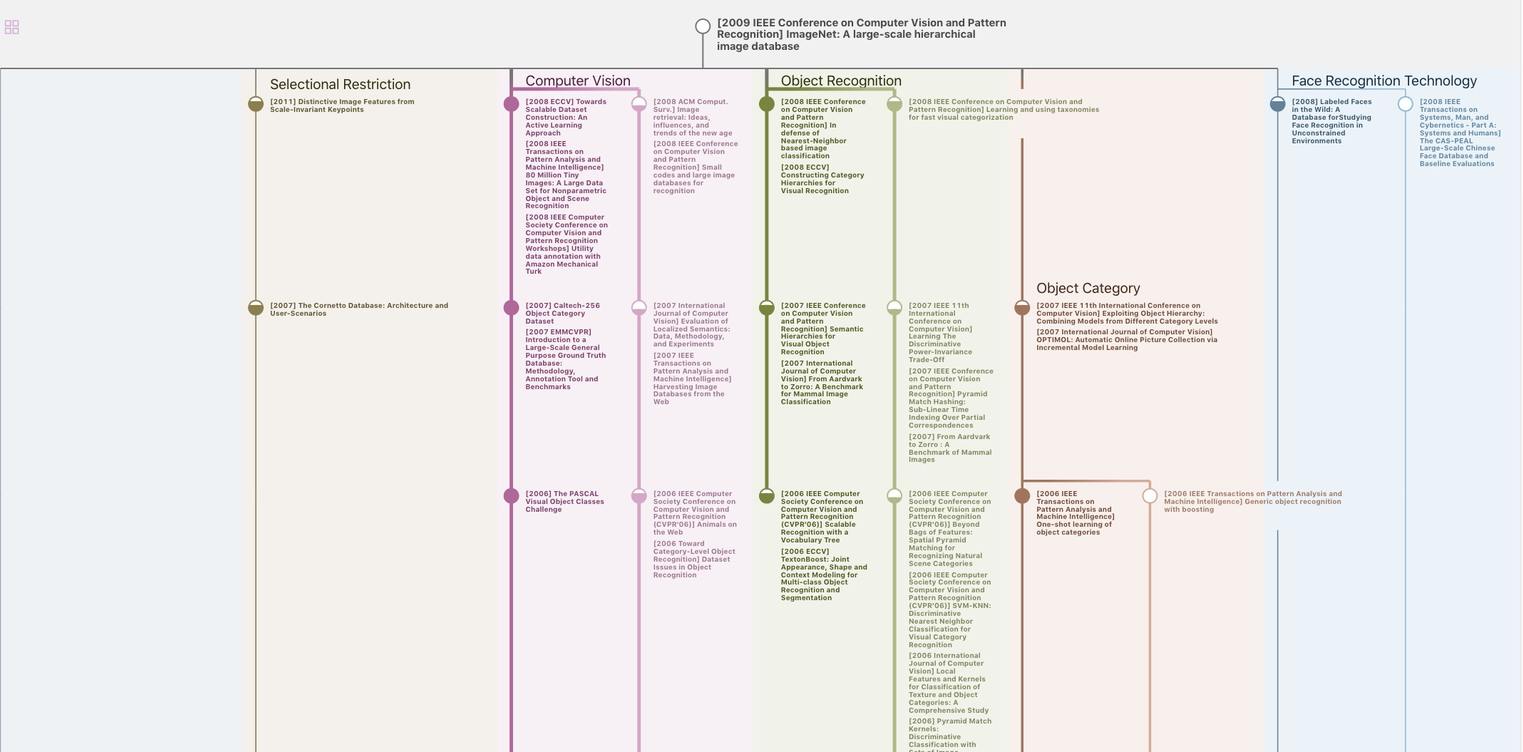
生成溯源树,研究论文发展脉络
Chat Paper
正在生成论文摘要