RSG-GCN: Predicting Semantic Relationships in Urban Traffic Scene With Map Geometric Prior
IEEE Open Journal of Intelligent Transportation Systems(2023)
摘要
Automated identification of the relationships between traffic actors and surrounding objects, in order to describe their behavior and predict their intentions, has become the focus of increasing attention in the field of autonomous driving. Therefore, in this work, we propose a Road Scene Graphs-Graph Convolutional Network (RSG-GCN) as a novel, graph-based model for predicting the topological graph structure of a given traffic scene. The status of the actors and HD map information are integrated as prior knowledge, allowing the edges linking the actor nodes to capture potential semantic relationships, such as “vehicle approaching pedestrian” and “pedestrian waiting at intersection”. To train this model, we created our own RSG dataset, as well as a relational dataset and benchmark derived from nuScenes. Our extensive range of experiments demonstrate that our model can more accurately predict semantic relationships and behavior in a given traffic scene than other popular traffic scene prediction models. In particular, regarding the use of HD map prior knowledge, we found that the resulting increase in accuracy significantly outweighs performance loss caused by the increase in graph size. The downstream applications of RSG include traffic scene retrieval and synthetic traffic scene generation, which are briefly described.
更多查看译文
关键词
Graph neural network,semantic relationship prediction,traffic scene understanding
AI 理解论文
溯源树
样例
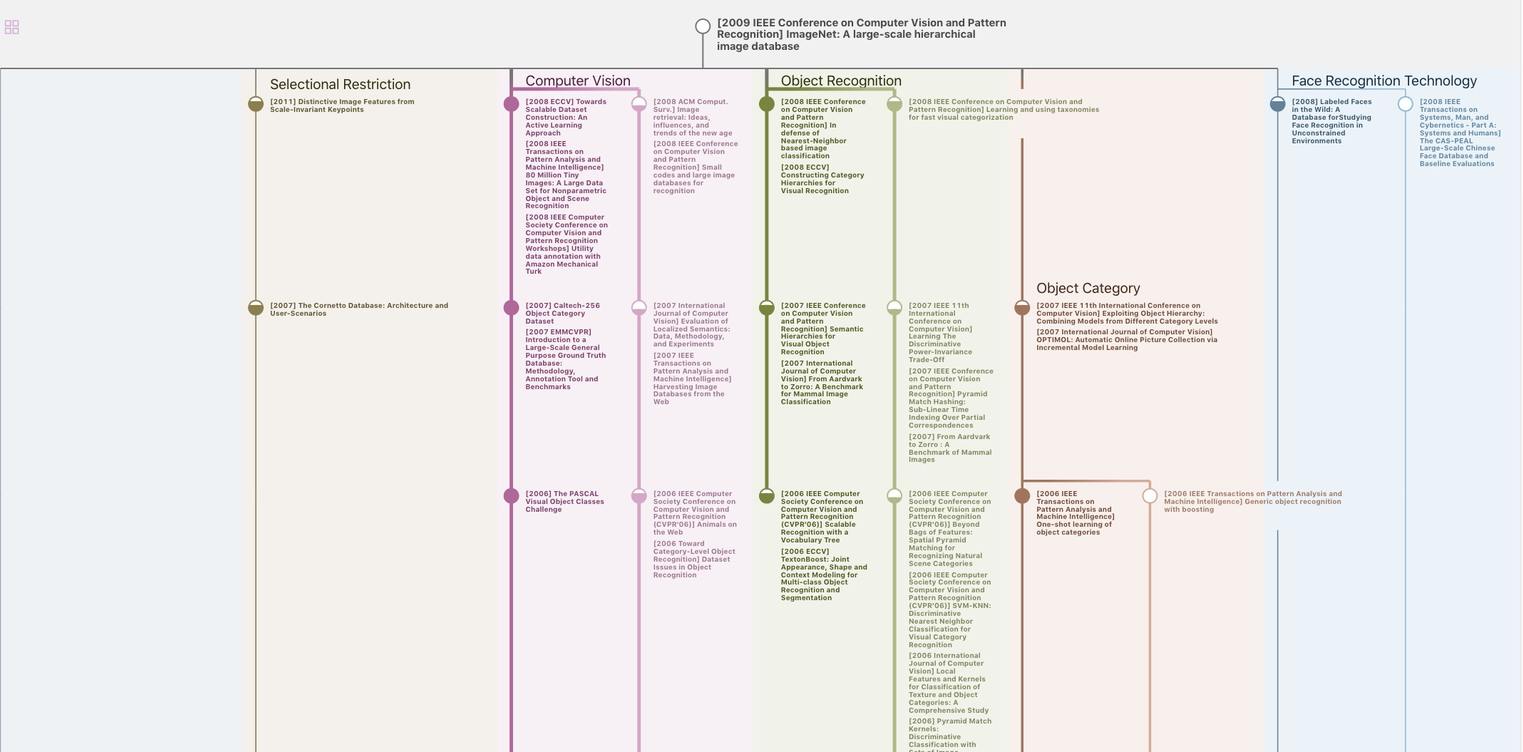
生成溯源树,研究论文发展脉络
Chat Paper
正在生成论文摘要