LiteST-Net: A Hybrid Model of Lite Swin Transformer and Convolution for Building Extraction from Remote Sensing Image
Remote Sensing(2023)
摘要
Extracting building data from remote sensing images is an efficient way to obtain geographic information data, especially following the emergence of deep learning technology, which results in the automatic extraction of building data from remote sensing images becoming increasingly accurate. A CNN (convolution neural network) is a successful structure after a fully connected network. It has the characteristics of saving computation and translation invariance with improved local features, but it has difficulty obtaining global features. Transformers can compensate for the shortcomings of CNNs and more effectively obtain global features. However, the calculation number of transformers is excessive. To solve this problem, a Lite Swin transformer is proposed. The three matrices Q, K, and V of the transformer are simplified to only a V matrix, and the v of the pixel is then replaced by the v with the largest projection value on the pixel feature vector. In order to better integrate global features and local features, we propose the LiteST-Net model, in which the features extracted by the Lite Swin transformer and the CNN are added together and then sampled up step by step to fully utilize the global feature acquisition ability of the transformer and the local feature acquisition ability of the CNN. The comparison experiments on two open datasets are carried out using our proposed LiteST-Net and some classical image segmentation models. The results show that compared with other networks, all metrics of LiteST-Net are the best, and the predicted image is closer to the label.
更多查看译文
关键词
litest-net swin transformer,remote sensing,building extraction,convolution
AI 理解论文
溯源树
样例
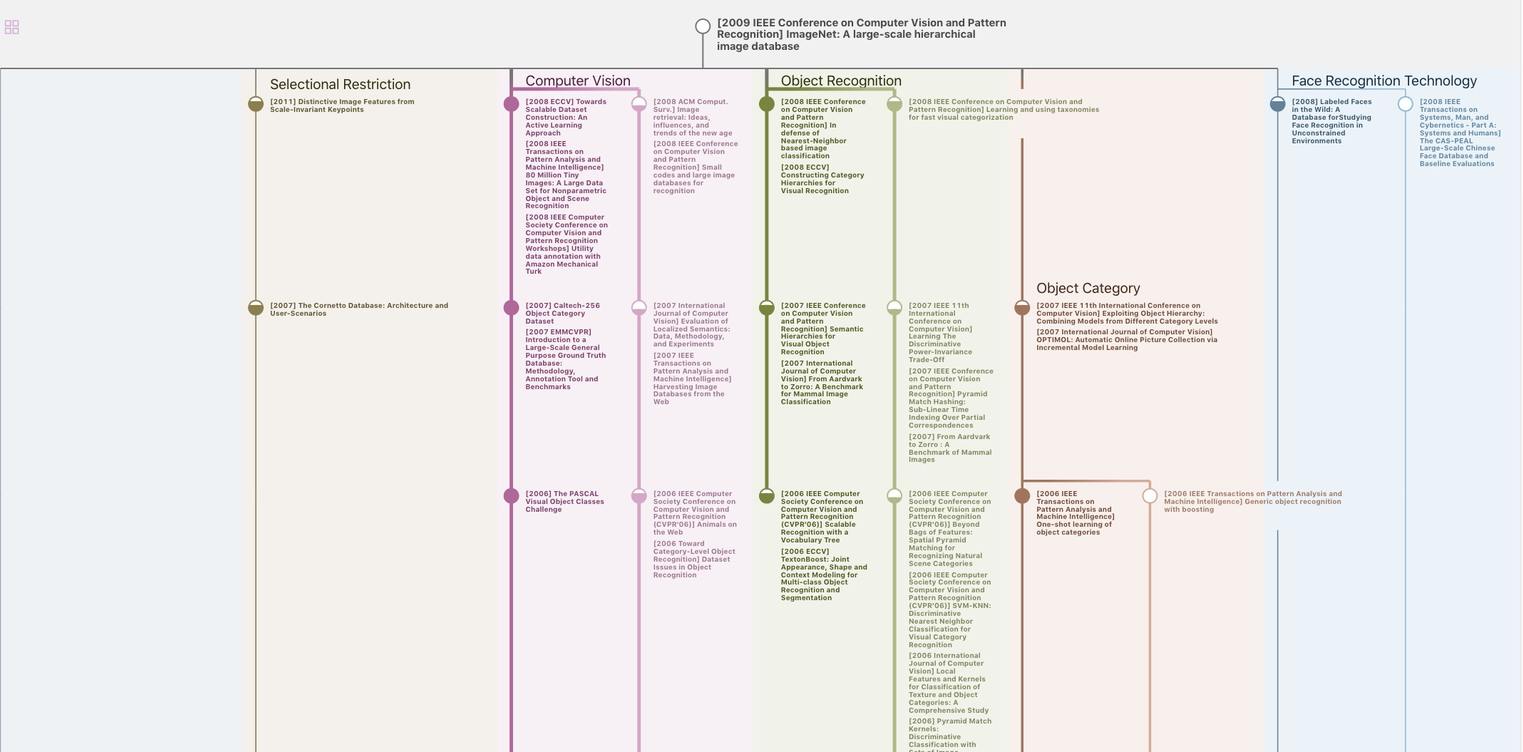
生成溯源树,研究论文发展脉络
Chat Paper
正在生成论文摘要