A global–local attention network for uncertainty analysis of ground penetrating radar modeling
Reliability Engineering & System Safety(2023)
摘要
A global–local attention-based feature reconstruction (GLAFR) surrogate model is proposed for uncertainty analysis (UA) in ground penetrating radar (GPR) simulation. The uncertain inputs are converted to electric fields by the surrogate model instead of the full-wave simulation, and the uncertainty of output is quantified effectively. In the model, the global feature scaling (GFS) and the local feature reconstruction (LFR) are employed to obtain the long-term and short-term relationships of features. In addition, a new loss function is proposed to accelerate the convergence of the model for training data with a wider range of input disturbances. The validity of the surrogate model is verified by the UA result from the Monte Carlo method (MCM). Compared with existing deep learning methods, the proposed approach can efficiently get higher quality predictions. Meanwhile, the Sobol indices evaluated by GLAFR are in agreement with those of MCM which requires running the full-wave simulation one thousand times to converge.
更多查看译文
关键词
Attention mechanism,Deep learning,Ground penetrating radar,Uncertainty analysis,Multi-scale
AI 理解论文
溯源树
样例
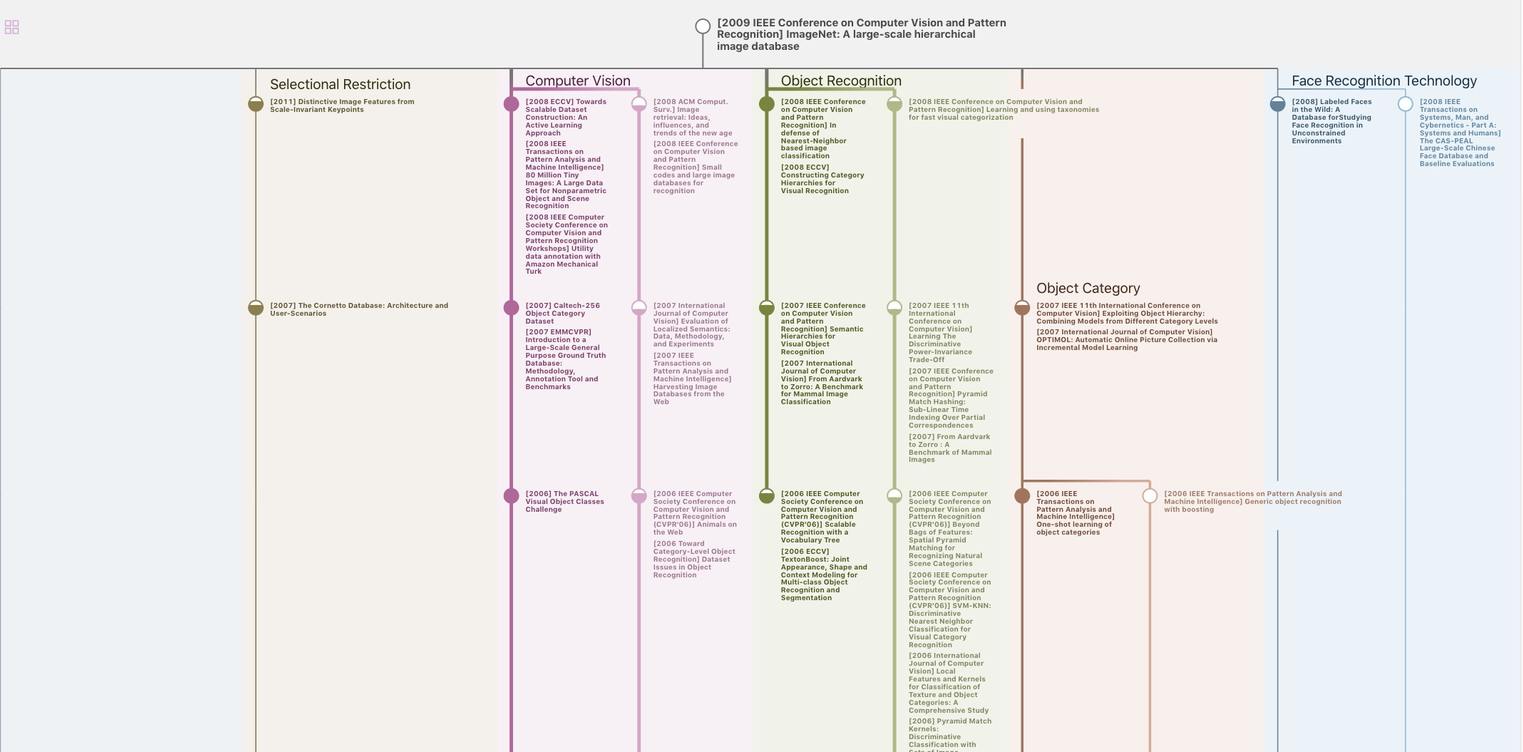
生成溯源树,研究论文发展脉络
Chat Paper
正在生成论文摘要