Active Learning with Controllable Augmentation Induced Acquisition
ICLR 2023(2023)
摘要
The mission of active learning is to iteratively identify the most informative data samples to annotate, and therefore to attain decent performance with much fewer samples. Despite the promise, the acquisition of informative unlabeled samples can be unreliable --- particularly during early cycles --- owning to limited data samples and sparse supervision. To tackle this, the data augmentation techniques seem straightforward yet promising to easily extend the exploration of the input space. In this work, we thoroughly study the coupling of data augmentation and active learning whereby we propose Controllable Augmentation ManiPulator for Active Learning. In contrast to the few prior work that touched on this line, CAMPAL emphasizes a tighten and better-controlled integration of data augmentation into the active learning framework, as in three folds: (i)-carefully designed data augmentation policies applied separately on labeled and unlabeled data pool in every cycle; (ii)-controlled and quantifiably optimizable augmentation strengths; (iii)-full but flexible coverage for most (if not all) active learning schemes. Through extensive empirical experiments, we bring the performance of active learning methods to a new level: an absolute performance boost of 16.99% on CIFAR-10 and 12.25% on SVHN with 1,000 annotated samples. Complementary to the empirical results, we further provide theoretical analysis and justification of CAMPAL.
更多查看译文
关键词
active learning,data augmentation,strength
AI 理解论文
溯源树
样例
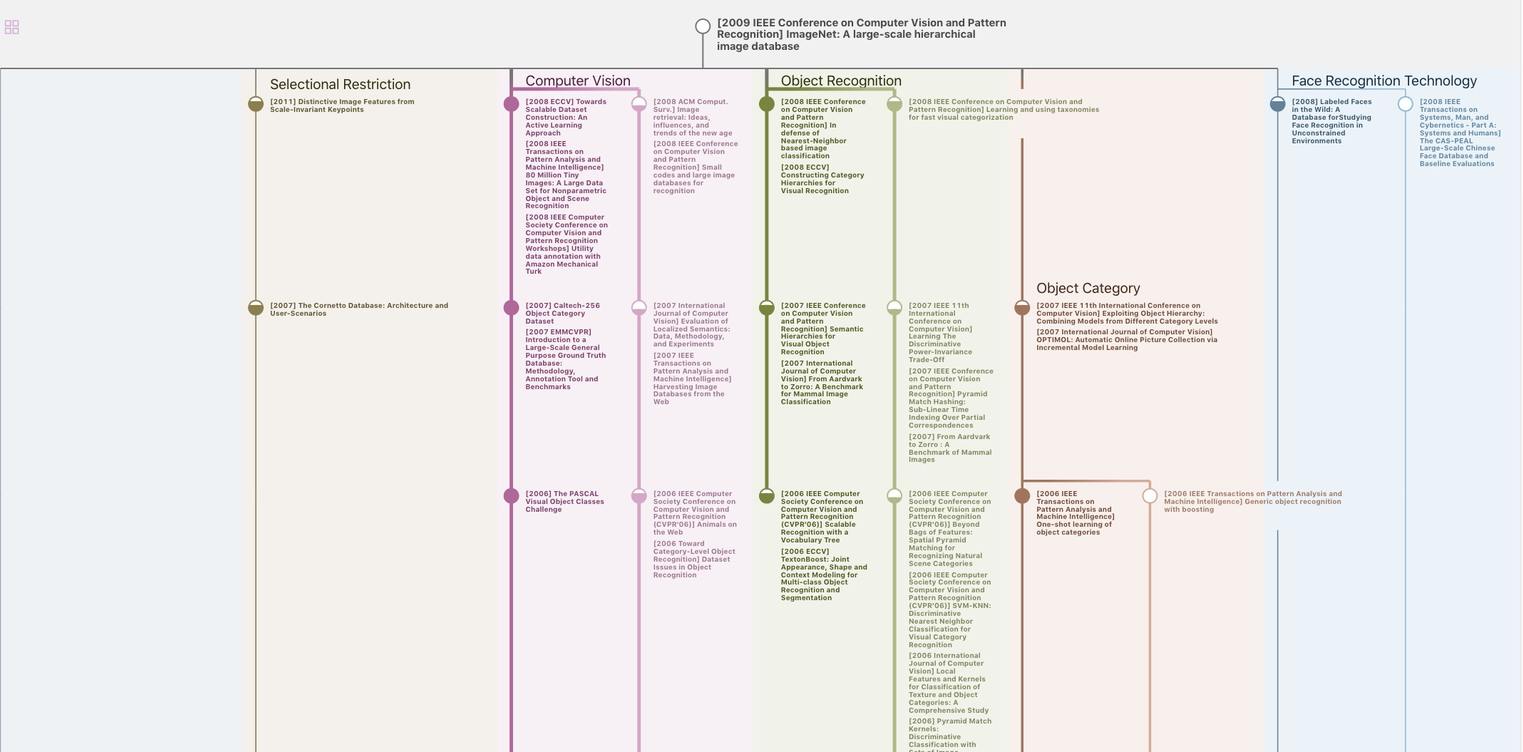
生成溯源树,研究论文发展脉络
Chat Paper
正在生成论文摘要