Temporary feature collapse phenomenon in early learning of MLPs
ICLR 2023(2023)
摘要
In this paper, we focus on a typical two-phase phenomenon in the learning of multi-layer perceptrons (MLPs). We discover and explain the reason for the feature collapse phenomenon in the first phase, i.e., the diversity of features over different samples keeps decreasing in the first phase, until samples of different categories share almost the same feature, which hurts the optimization of MLPs. We explain such a phenomenon in terms of the learning dynamics of MLPs. Furthermore, we theoretically analyze the reason why four typical operations can alleviate the feature collapse. The code has been attached with the submission.
更多查看译文
关键词
Neural Networks,Deep Learning Theory,Multi-Layer Perceptrons
AI 理解论文
溯源树
样例
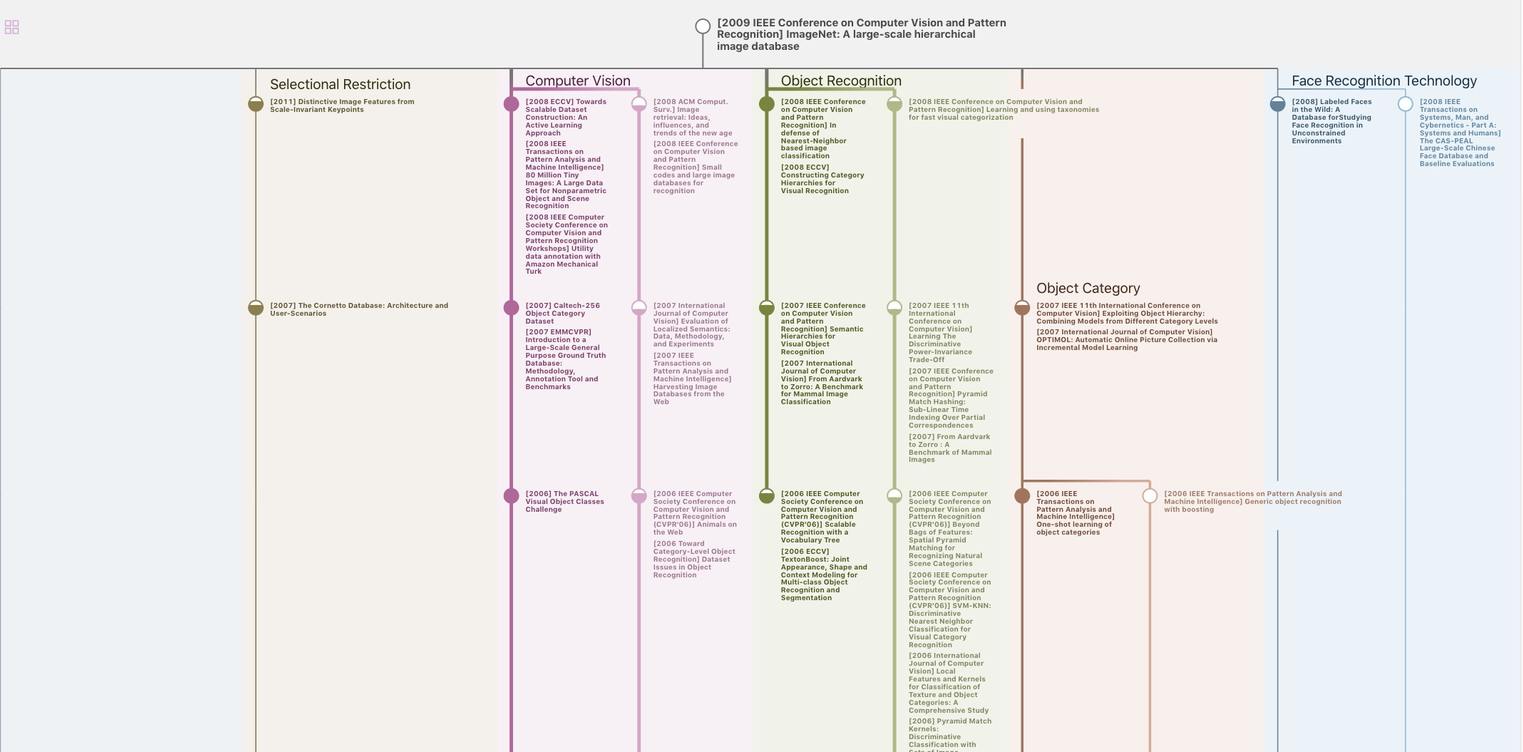
生成溯源树,研究论文发展脉络
Chat Paper
正在生成论文摘要