Computational-Unidentifiability in Representation for Fair Downstream Tasks
ICLR 2023(2023)
摘要
Deep representation learning methods are highlighted as they outperform classical algorithms in various downstream tasks, such as classification, clustering, generative models, etc. Due to their success and impact on the real world, fairness concern is rising with noticeable attention. However, the focus of the fairness problem was limited to a certain downstream task, mostly classification, and few were studied from the perspective of representation itself. We claim that the fairness problems to various downstream tasks originated from the input feature space, i.e., the learned representation space. While several studies explored fair representation for the classification task, the fair representation learning method for unsupervised learning is not actively discussed. To fill this gap, we define a new notion of fairness, computational-unidentifiability, which suggests the fairness of the representation as the distributional independence of the sensitive groups. We demonstrate motivating problems that achieving computationally-unidentifiable representation is critical for fair downstream tasks. Moreover, we propose a novel fairness metric, Fair Fréchet distance (FFD), to quantify the computational-unidentifiability and address the limitation of a well-known fairness metric for unsupervised learning, i.e., balance. The proposed metric is efficient in computation and preserves theoretical properties. We empirically validate the effectiveness of the computationally-unidentifiable representations in various downstream tasks.
更多查看译文
关键词
fairness,representation learning
AI 理解论文
溯源树
样例
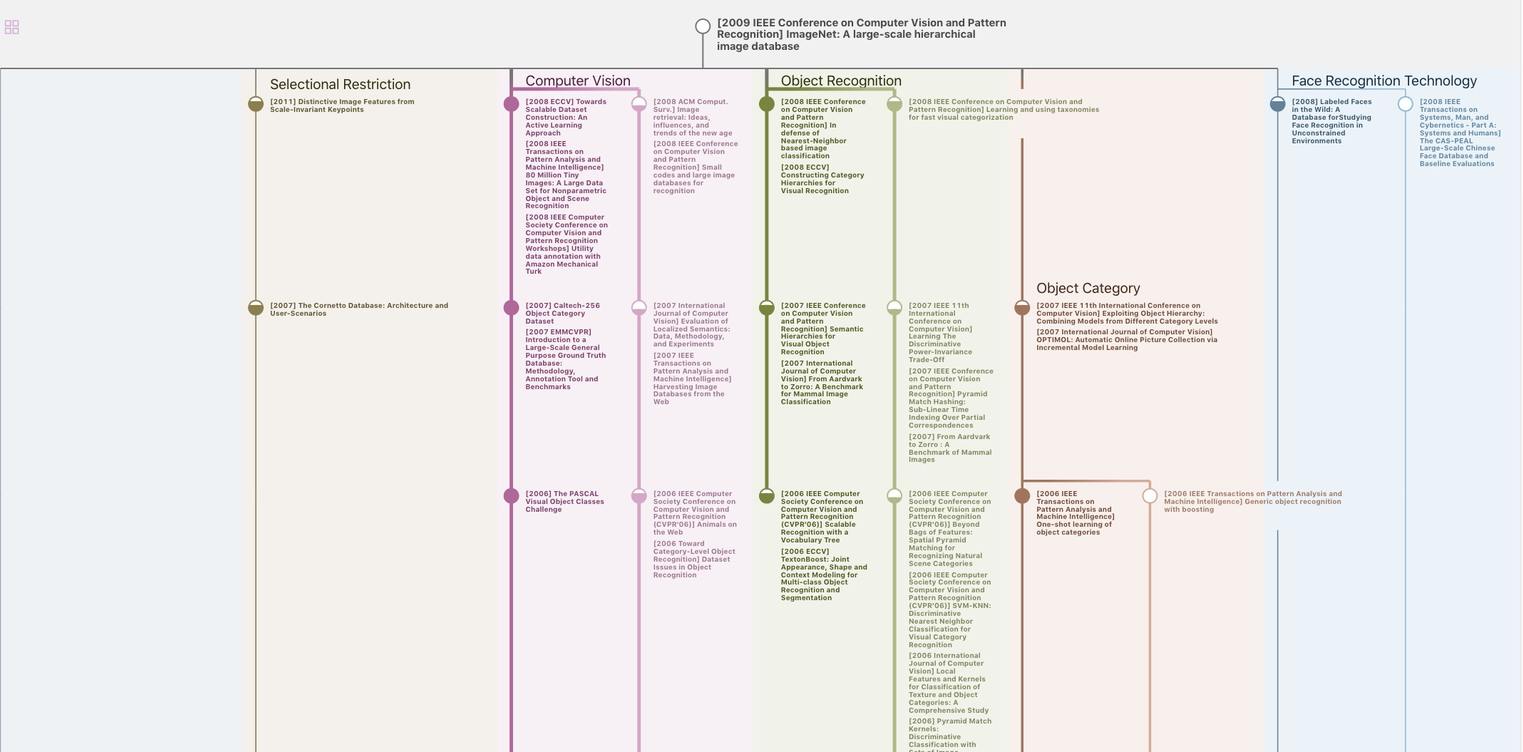
生成溯源树,研究论文发展脉络
Chat Paper
正在生成论文摘要