Iterative $\alpha$-(de)Blending: Learning a Deterministic Mapping Between Arbitrary Densities
ICLR 2023(2023)
摘要
We present a learning method that produces a mapping between arbitrary densities, such that random samples of a density can be mapped to random samples of another. In practice, our method is similar to deterministic diffusion processes where samples of the target density are blended with Gaussian noise. The originality of our approach is that, in contrast to several recent works, we do not rely on Langevin dynamics or score-matching concepts. We propose a simpler take on the topic, which is based solely on Bayes' theorem. By studying blended samples and their posteriors, we show that iteratively blending and deblending samples produces random paths between arbitrary densities. We prove that, for finite-variance densities, these paths converge towards a deterministic mapping that can be learnt with a neural network trained to deblend samples. Our method can thus be seen as a generalization of deterministic denoising diffusion where, instead of learning to denoise Gaussian noise, we learn to deblend arbitrary data.
更多查看译文
关键词
Deterministic Denoising Diffusion
AI 理解论文
溯源树
样例
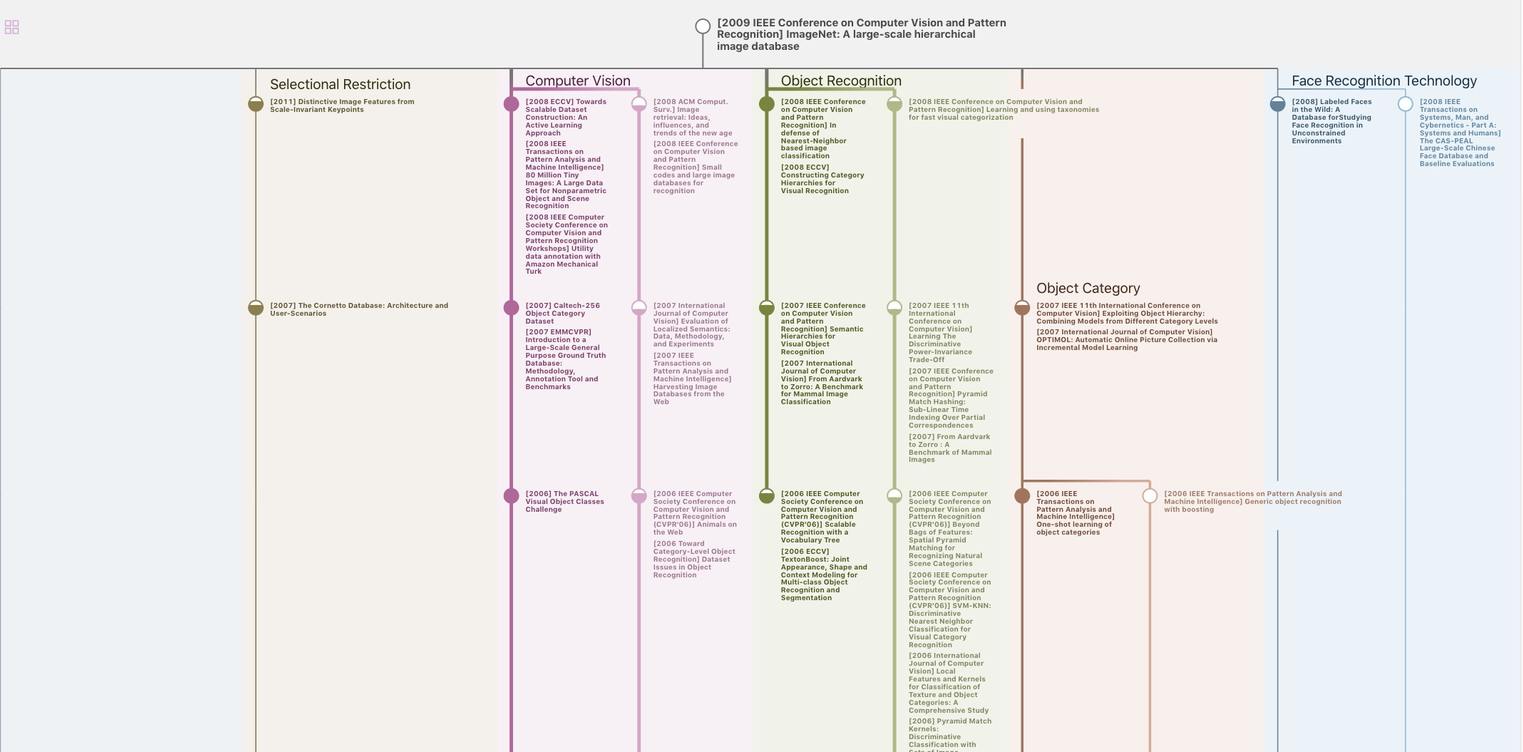
生成溯源树,研究论文发展脉络
Chat Paper
正在生成论文摘要