A spatiotemporal graph neural network with multi granularity for air quality prediction
ICLR 2023(2023)
摘要
Air quality prediction is a complex system engineering. How to fully consider the impact of meteorological, spatial and temporal factors on air quality is the core problem. To address this central conundrum, in an elaborate encoder-decoder architecture, we propose a new air quality prediction method based on multi-granularity spatiotemporal graph network. At the encoder, firstly, we use multi granularity graph and the well-known HYSPLIT model to build spatial relationship and dynamic edge relationship between nodes, respectively, while meteorological, temporal and topographic characteristics are used to build node features and LSTM (Long Short Term Memory) is used to learn the time-series relationship of pollutant concentration. At the decoder, secondly, we use the attention mechanism LSTM for decoding and forecasting of pollutant concentration. The proposed model is capable of tracking different influences on prediction resulting from the changes of air quality. On a project-based dataset, we validate the effectiveness of the proposed model and examine its abilities of capturing both fine-grained and long-term influences in pollutant process. We also compare the proposed model with the state-of-the-art air quality forecasting methods on the dataset of Yangtze River Delta city group, the experimental results show the appealing performance of our model over competitive baselines.
更多查看译文
关键词
Air quality prediction,graph neural network,long short term memory
AI 理解论文
溯源树
样例
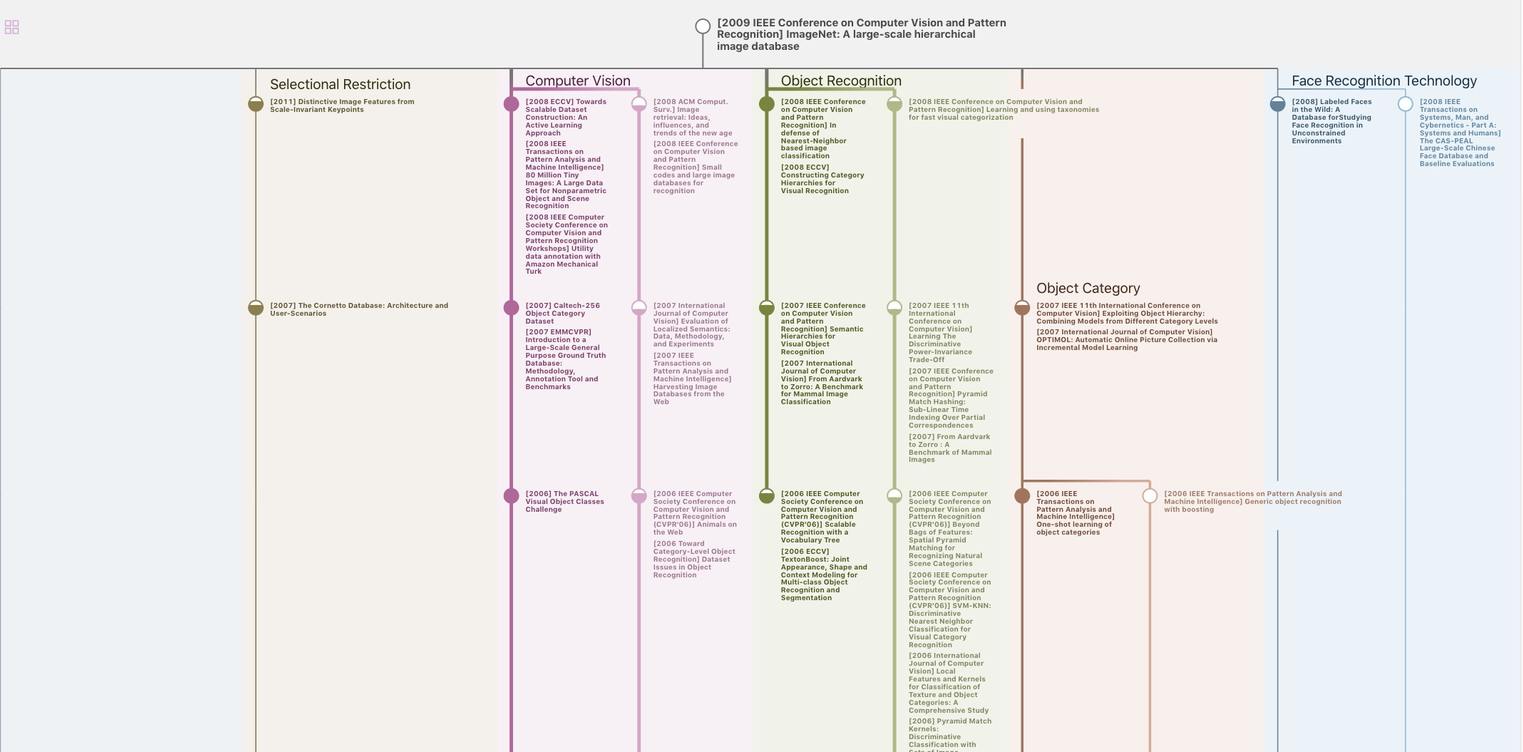
生成溯源树,研究论文发展脉络
Chat Paper
正在生成论文摘要