Breaking Beyond COCO Object Detection
ICLR 2023(2023)
摘要
COCO dataset has become the de facto standard for training and evaluating object
detectors. According to the recent benchmarks, however, performance on this
dataset is still far from perfect, which raises the following questions, a) how far can
we improve the accuracy on this dataset using deep learning, b) what is holding
us back in making progress in object detection, and c) what are the limitations
of the COCO dataset and how can they be mitigated. To answer these questions,
first, we propose a systematic approach to determine the empirical upper bound
in AP over COCOval2017, and show that this upper bound is significantly higher
than the state-of-the-art mAP (78.2% vs. 58.8%). Second, we introduce two
complementary datasets to COCO: i) COCO_OI, composed of images from COCO
and OpenImages (from 80 classes in common) with 1,418,978 training bounding
boxes over 380,111 images, and 41,893 validation bounding boxes over 18,299
images, and ii) ObjectNet_D containing objects in daily life situations (originally
created for object recognition known as ObjectNet; 29 categories in common with
COCO). We evaluate models on these datasets and pinpoint the annotation errors
on the COCO validation set. Third, we characterize the sources of errors in modern
object detectors using a recently proposed error analysis tool (TIDE) and find that
models behave differently on these datasets compared to COCO. For instance,
missing objects are more frequent in the new datasets. We also find that models
lack out of distribution generalization. Code and data will be shared.
更多查看译文
关键词
object detection,deep learning,performance analysis
AI 理解论文
溯源树
样例
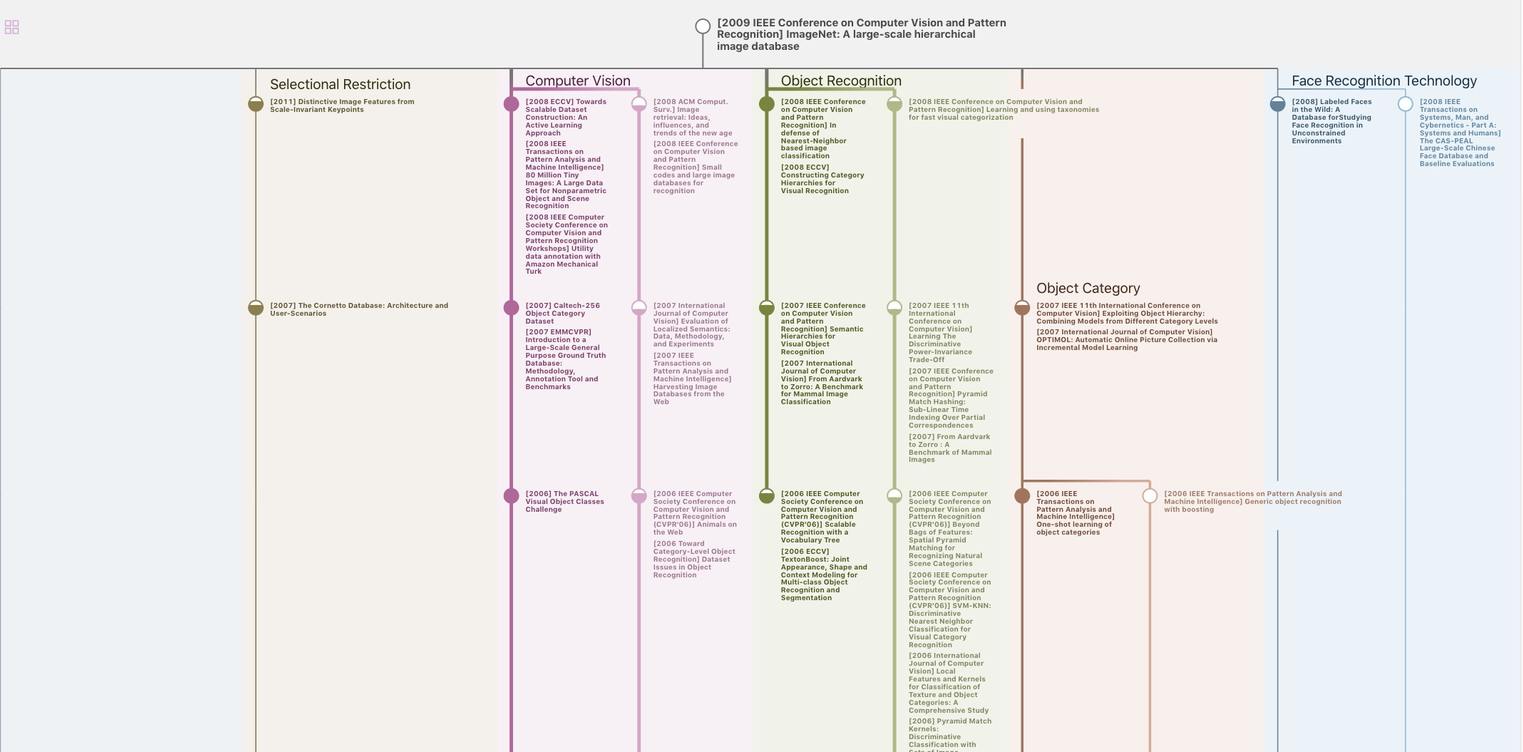
生成溯源树,研究论文发展脉络
Chat Paper
正在生成论文摘要