Make Memory Buffer Stronger in Continual Learning: A Continuous Neural Transformation Approach
ICLR 2023(2023)
摘要
Continual learning (CL) focuses on learning non-stationary data distribution without forgetting previous knowledge. However, the most widely used memory-replay approach often suffers from memory overfitting. To mitigate the memory overfitting, we propose a continuous and reversible memory transformation method so that the memory data is hard to overfit, thus improving generalization. The transformation is achieved by optimizing a bi-level optimization objective that jointly learns the CL model and memory transformer. Specifically, we propose a deterministic continuous memory transformer (DCMT) modeled by an ordinary differential equation, allowing for infinite memory transformation and generating diverse and hard memory data. Furthermore, we inject uncertainty into the transformation function and propose a stochastic continuous memory transformer (SCMT) modeled by a stochastic differential equation, which substantially enhances the diversity of the transformed memory buffer. The proposed neural transformation approaches have significant advantages over existing ones: (1) we can obtain infinite many transformed data, thus significantly increasing the memory buffer diversity; (2) the proposed continuous transformations are reversible, i.e., the original raw memory data could be restored from the transformed memory data without the need to make a replica of the memory data. Extensive experiments on both task-aware and task-free CL show significant improvement with our approach compared to strong baselines.
更多查看译文
关键词
Continual Learning
AI 理解论文
溯源树
样例
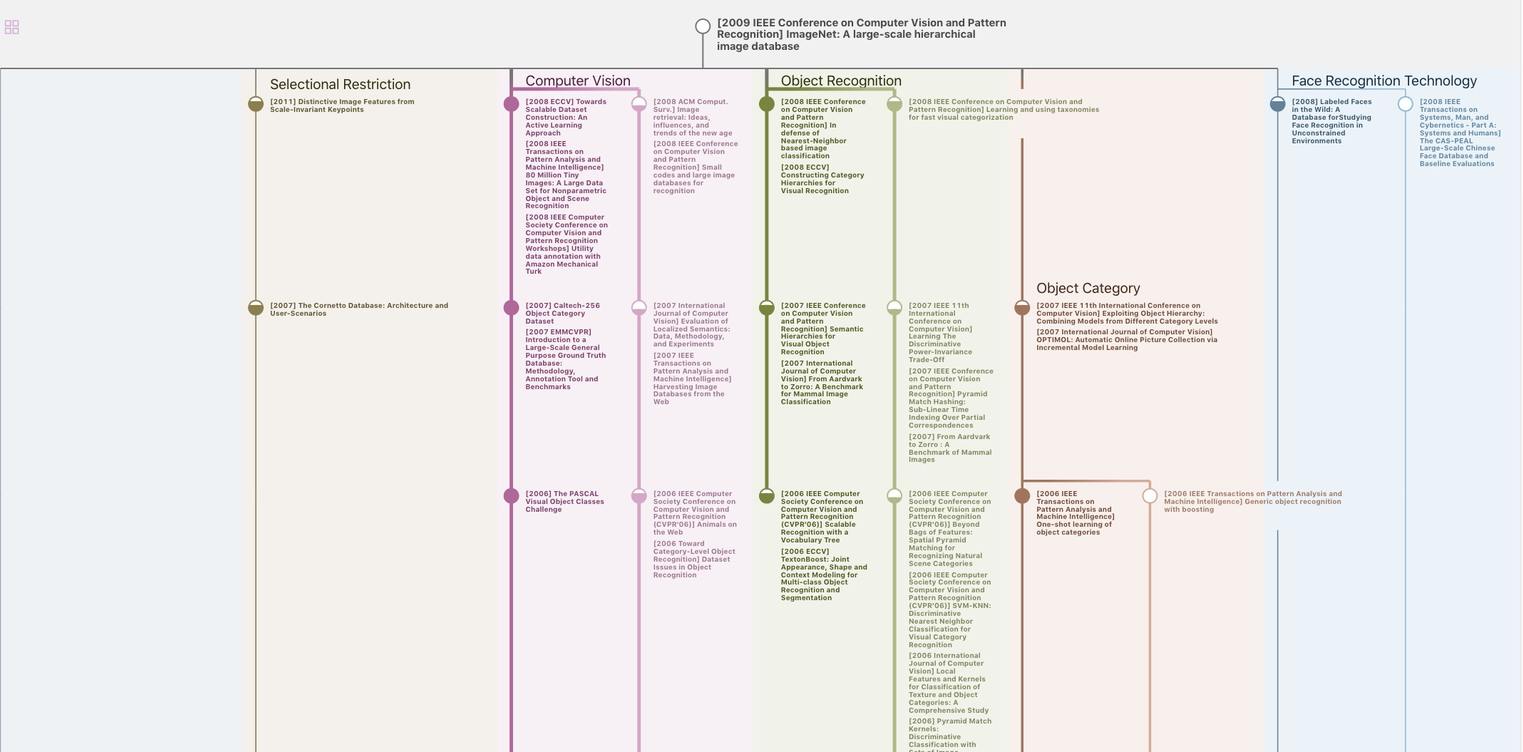
生成溯源树,研究论文发展脉络
Chat Paper
正在生成论文摘要