NEW TRAINING FRAMEWORK FOR SPEECH ENHANCEMENT USING REAL NOISY SPEECH
ICLR 2023(2023)
摘要
Recently, deep learning-based speech enhancement (SE) models have gained
significant improvements. However, the success is mainly based on using synthetic
training data created by adding clean speech with noise. On the other hand, in spite
of its large amount, real noisy speech is hard to be applied for SE model training
because of lack of its clean reference. In this paper, we propose a novel method
to utilize real noisy speech for SE model training based on a non-intrusive speech
quality prediction model. The SE model is trained through the guide of the quality
prediction model. We also find that a speech quality predictor with better accuracy
may not necessarily be an appropriate teacher to guide the SE model. In addition,
we show that if the quality prediction model is adversarially robust, then the
prediction model itself can also be served as a SE model by modifying the input
noisy speech through gradient backpropagation. Objective experiment results show
that, under the same SE model structure, the proposed new training method trained
on a large amount of real noisy speech can outperform the conventional supervised
model trained on synthetic noisy speech. Lastly, the two training methods can be
combined to utilize both benefits of synthetic noisy speech (easy to learn) and real
noisy speech (large amount) to form semi-supervised learning which can further
boost the performance both objectively and subjectively. The code will be released
after publication.
更多查看译文
关键词
Speech enhancement,Quality prediction,Semi-supervised learning,Adversarially robust
AI 理解论文
溯源树
样例
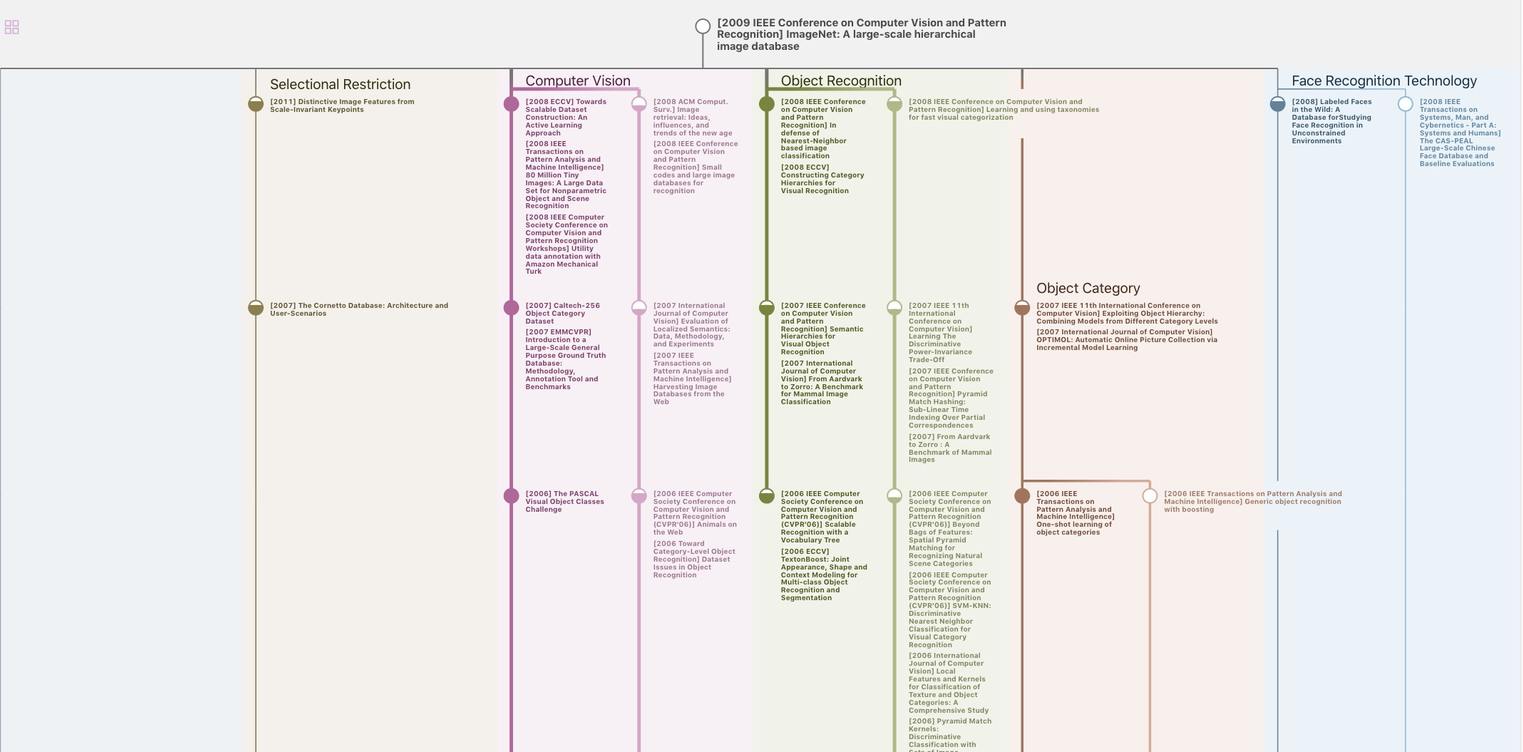
生成溯源树,研究论文发展脉络
Chat Paper
正在生成论文摘要