Do We Need Neural Collapse? Learning Diverse Features for Fine-grained and Long-tail Classification
ICLR 2023(2023)
摘要
Feature extractors learned from supervised training of deep neural networks have demonstrated superior performance over handcrafted ones. Recently, it is shown that such learned features have a neural collapse property, where within-class features collapse to the class mean and different class means are maximally separated. This paper examines the neural collapse property in the context of fine-grained classification tasks, where a feature extractor pretrained from a classification task with coarse labels is used for generating features for a downstream classification task with fine-grained labels. We argue that the within-class feature collapse is an undesirable property for fine-grained classification. Hence, we introduce a geometric arrangement of features called the maximal-separating-cone, where within-class features lie in a cone of nontrivial radius instead of collapsing to the class mean, and cones of different classes are maximally separated. We present a technique based on classifier weight and training loss design to produce such an arrangement. Experimentally we demonstrate an improved fine-grained classification performance with a feature extractor pretrained by our method. Moreover, our technique also provides benefits for the classification on data with long-tail distribution over classes. Our work may motivate future efforts on the design of better geometric arrangements of deep features.
更多查看译文
关键词
Neural Collapse,Diverse deep learning features,Finegrained transfer learning
AI 理解论文
溯源树
样例
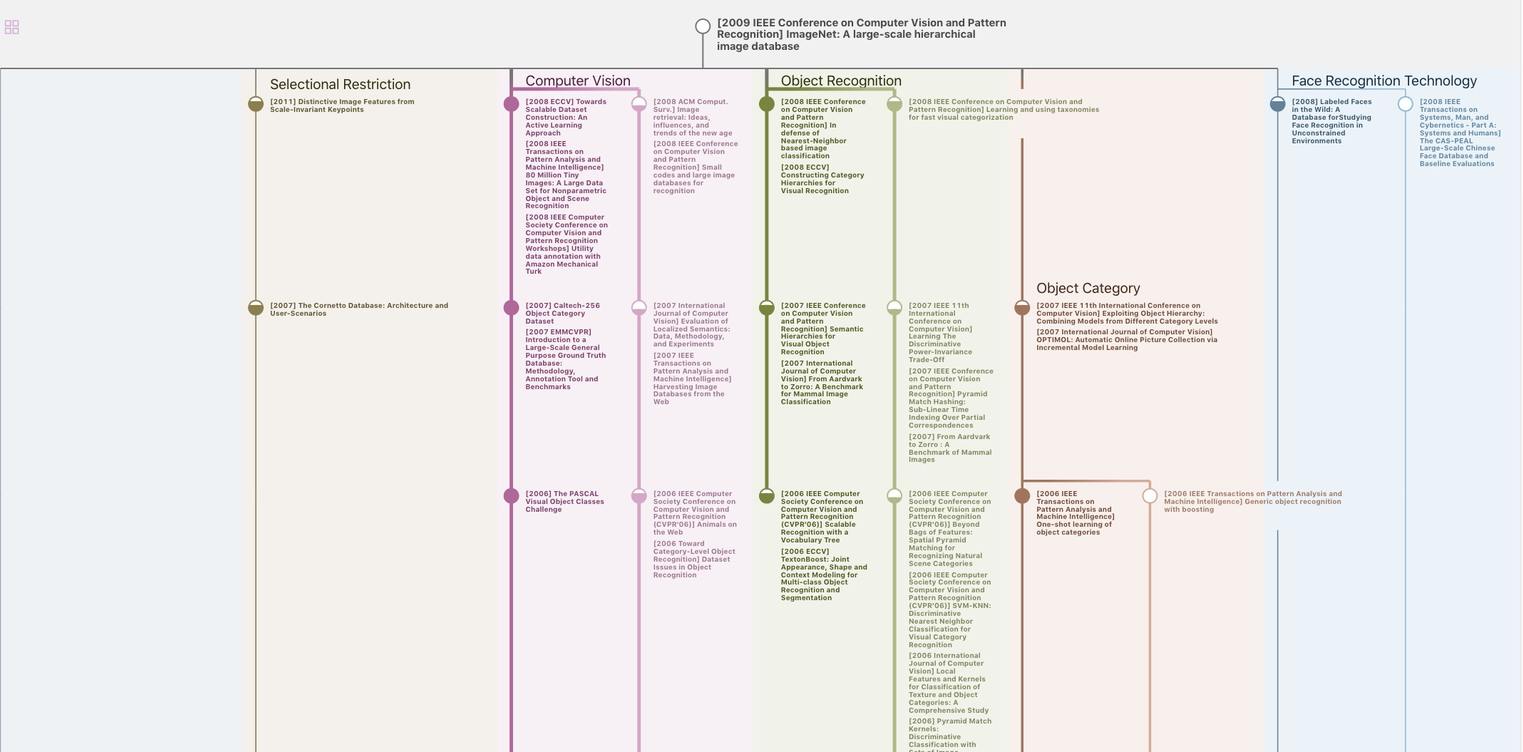
生成溯源树,研究论文发展脉络
Chat Paper
正在生成论文摘要