A Robustly and Effectively Optimized Pretraining Approach for Masked Autoencoder
ICLR 2023(2023)
摘要
Recently, Masked Image Modeling (MIM) has increasingly reshaped the status quo of self-supervised visual pre-training. This paper does not describe a novel MIM method, but to unravel several fundamental ingredients to robustly and effectively pre-train a Masked AutoEncoder (MAE) with improved downstream performance as a byproduct. We highlight the great significance for the whole autoencoder to encourage high-variance interactions across different tokens, while simultaneously for the reconstructed target to smooth the inter-patch variances. First, at the decoding phase, we apply the standard dropout upon the attention probabilities as noise to randomly mask out the edge connection across different tokens. Otherwise, their shortcut interactions might hinder the emergence of meaningful contextual representation. Second, we point out that the per-patch normalization will fail unless the patch pixels rely on some population statistics to reduce inter-patch variance and then smooth the reconstruction. Third, we show that autoencoders with different capacities encounter the issue to varying degrees and the learnable masked tokens can be employed to manipulate the variance dependent on its inserted position and ratio in the model. The proposed techniques here are simple and effective to benefit the pre-training of a masked autoencoder stably and obtain superior performance across different downstream tasks.
更多查看译文
AI 理解论文
溯源树
样例
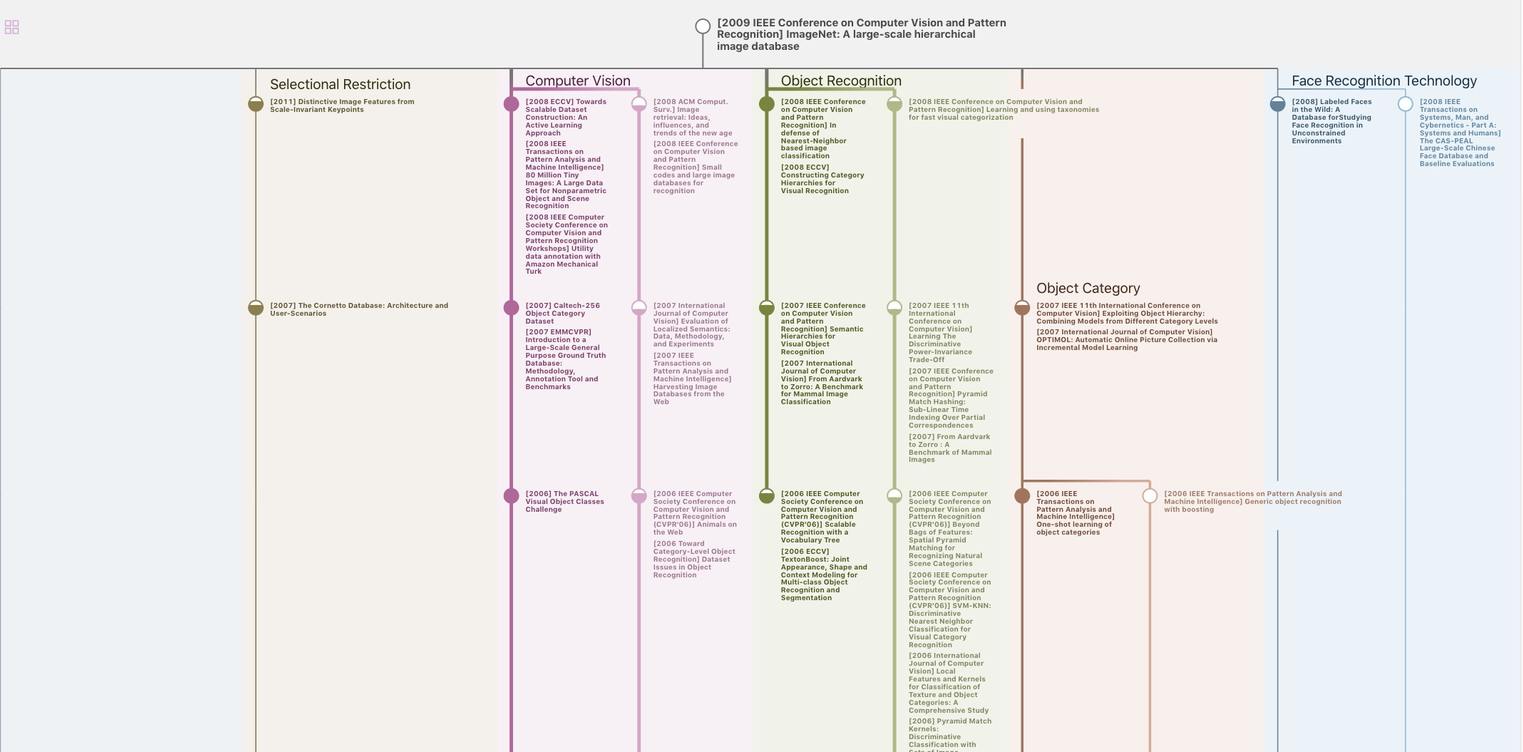
生成溯源树,研究论文发展脉络
Chat Paper
正在生成论文摘要