How Normalization and Weight Decay Can Affect SGD? Insights from a Simple Normalized Model
ICLR 2023(2023)
摘要
Recent works(Li et al., 2020, Wan et al., 2021) characterize an important mechanism of normalized model trained with SGD and WD (Weight Decay), called Spherical Motion Dynamics (SMD), confirming its widespread effects in practice. However, no theoretical study is available on the influence of SMD on the training process of normalized models in literature. In this work, we seek to understand the effect of SMD by theoretically analyzing a simple normalized model, named as Noisy Rayleigh Quotient (NRQ). On NRQ, We theoretically prove SMD can dominate the whole training process via controlling the evolution of angular update (AU), an essential feature of SMD. Specifically, we show: 1) within equilibrium state of SMD, the convergence rate and limiting risk of NRQ are mainly determined by the theoretical value of AU; and 2) beyond equilibrium state, the evolution of AU can interfere the optimization trajectory, causing odd phenomena such as ``escape'' behavior. We further show the insights drawn from NRQ is consistent with empirical observations in experiments on real datasets. We believe our theoretical results shed new light on the role of normalization techniques during the training of modern deep learning models.
更多查看译文
关键词
normalization,stochastic gradient descent,optimization
AI 理解论文
溯源树
样例
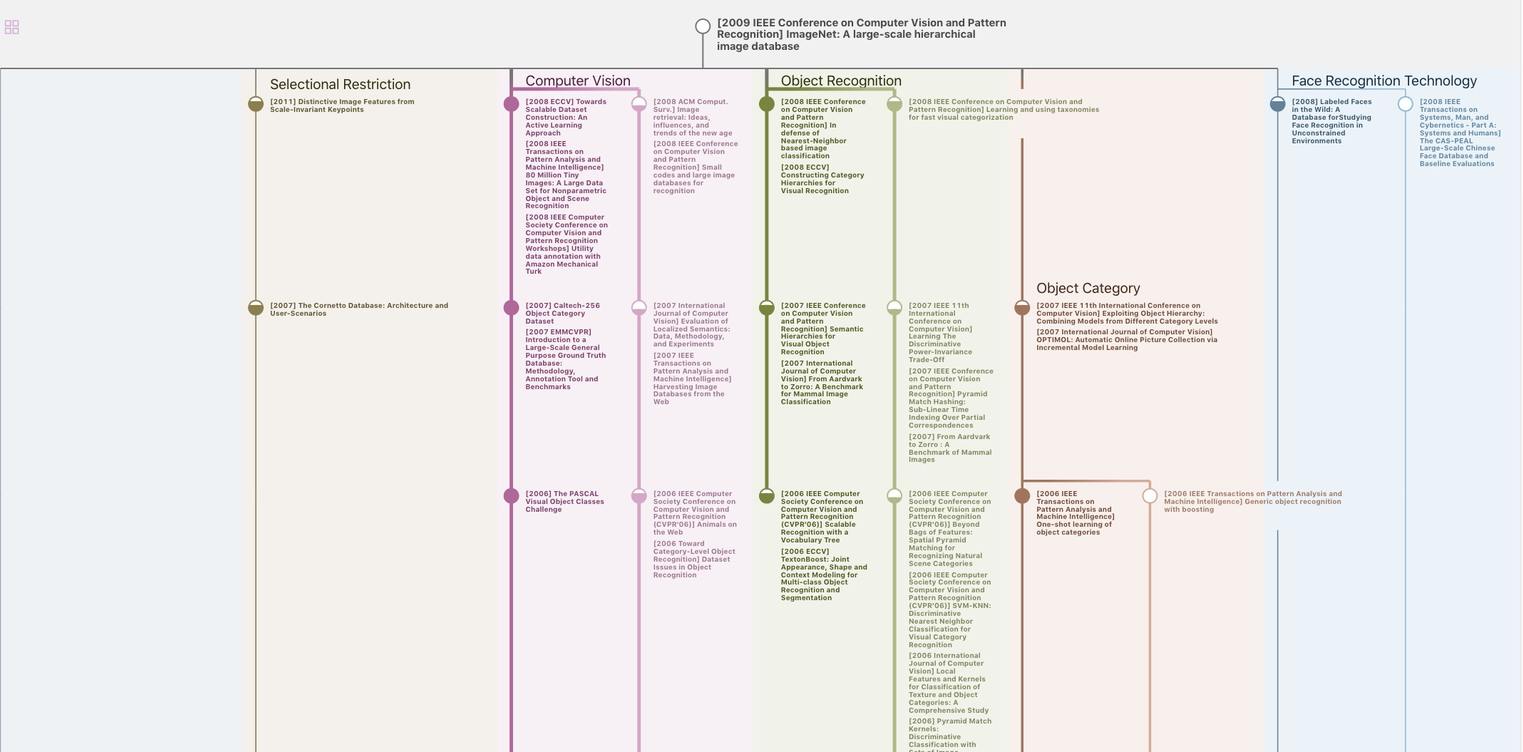
生成溯源树,研究论文发展脉络
Chat Paper
正在生成论文摘要