MULTILEVEL XAI: VISUAL AND LINGUISTIC BONDED EXPLANATIONS
ICLR 2023(2023)
摘要
Applications of deep neural networks are booming in more and more fields but lack transparency due to their black-box nature. Explainable Artificial Intelligence (XAI) is therefore of paramount importance, where strategies are proposed to understand how these black-box models function. The research so far mainly focuses on producing, for example, class-wise saliency maps, highlighting parts of a given image that affect the prediction the most. However, this way does not fully represent the way humans explain their reasoning and, awkwardly, validating these maps is quite complex and generally requires subjective interpretation. In this article, we conduct XAI differently by proposing a new XAI methodology in a multilevel (i.e., visual and linguistic) manner. By leveraging the interplay between the learned representations, i.e., image features and linguistic attributes, the proposed approach can provide salient attributes and attribute-wise saliency maps, which are far more intuitive than the class-wise maps, without requiring per-image ground-truth human explanations. It introduces self-interpretable attributes to overcome the current limitations in XAI and bring the XAI towards human-like level. The proposed architecture is simple in use and can reach surprisingly good performance in both prediction and explainability for deep neural networks thanks to the low-cost per-class attributes.
更多查看译文
关键词
Deep neural networks,Black box,Explainable Artificial Intelligence,Saliency maps
AI 理解论文
溯源树
样例
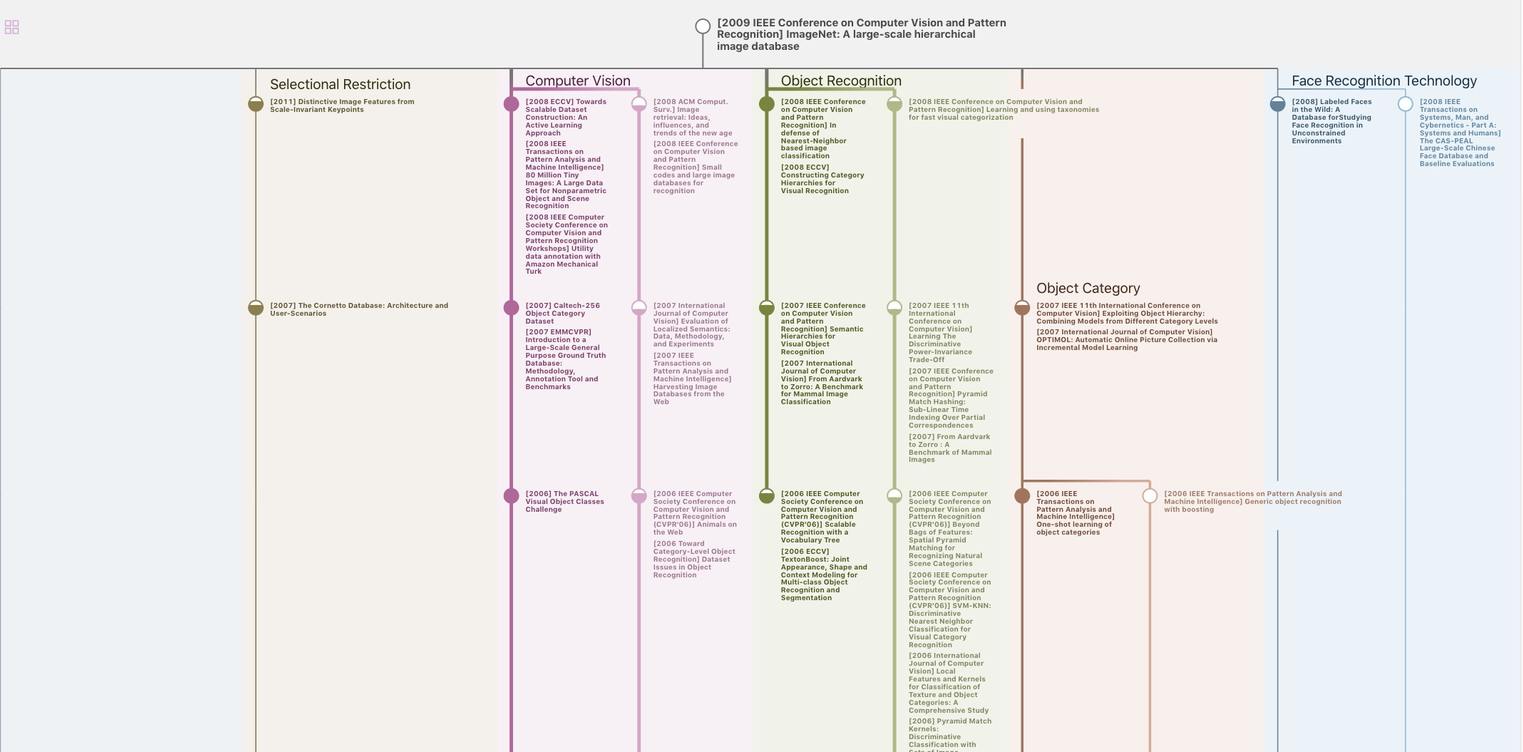
生成溯源树,研究论文发展脉络
Chat Paper
正在生成论文摘要