A Unified Framework for Comparing Learning Algorithms
ICLR 2023(2023)
摘要
We propose a framework for {\em (learning) algorithm comparisons}, wherein the goal is to find similarities and differences between models trained with two different learning algorithms. We begin by formalizing the goal of algorithm comparison as finding {\em distinguishing feature transformations}, input transformations that change the predictions of models trained with one learning algorithm but not the other. We then present a two-stage method for algorithm comparisons based on comparing how models use the training data, leveraging the recently proposed datamodel representations [Ilyas et al., 2022]. We demonstrate our framework through three case studies that compare models trained with/without standard data augmentation, with/without pre-training, and with different optimizer hyperparameters.
更多查看译文
关键词
algorithm comparison,model comparison,data-centric,influence,datamodels,data augmentation,pretraining,learning rate
AI 理解论文
溯源树
样例
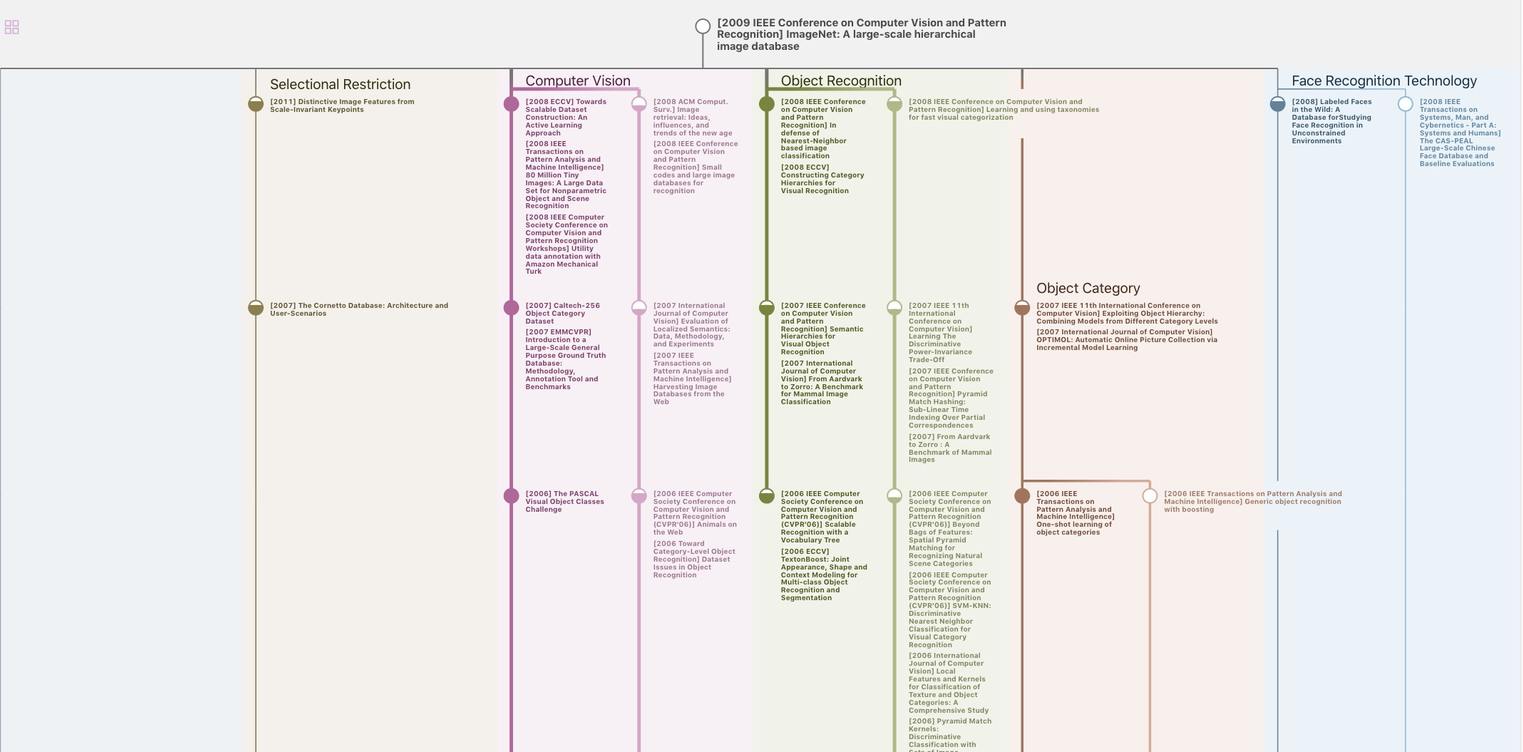
生成溯源树,研究论文发展脉络
Chat Paper
正在生成论文摘要