Pairwise Confidence Difference on Unlabeled Data is Sufficient for Binary Classification
ICLR 2023(2023)
摘要
Learning with confidence labels is an emerging weakly supervised learning paradigm, where training data are equipped with confidence labels instead of exact labels. Positive-confidence (Pconf) classification is a typical learning problem in this context, where we are given only positive data equipped with confidence. However, pointwise confidence may not be accessible in real-world scenarios. In this paper, we dive into a novel weakly supervised learning problem called confidence-difference (ConfDiff) classification. Instead of pointwise confidence, we are given only unlabeled data pairs equipped with confidence difference specifying the difference in the probabilities of being positive. An unbiased risk estimator is derived to tackle the problem, and we show that the estimation error bound achieves the optimal convergence rate. Extensive experiments on benchmark data sets validate the effectiveness of our proposed approaches in leveraging the supervision information of the confidence difference.
更多查看译文
关键词
Weakly supervised learning,binary classification,unbiased risk estimator
AI 理解论文
溯源树
样例
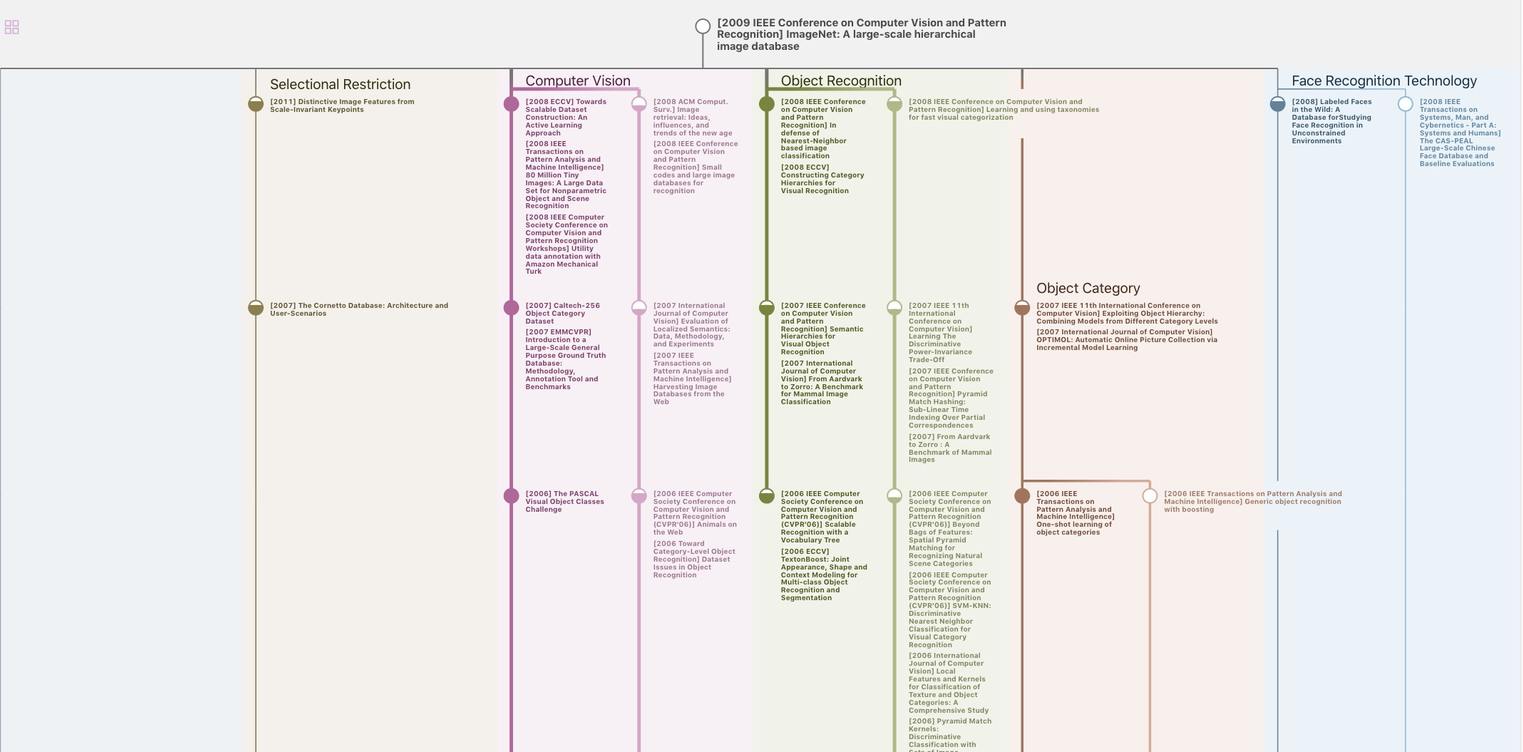
生成溯源树,研究论文发展脉络
Chat Paper
正在生成论文摘要