Topic and Hyperbolic Transformer to Handle Multi-modal Dependencies
ICLR 2023(2023)
摘要
As multi-modal search relies on jointly learning image-text representations and has been investigated in the literature,
our innovation is to develop Chimera, a framework in which to learn their representations and similarities.
Because the core of multi-modal search is learning the modalities in a shared semantic space and measuring their similarities,
search quality depends on which expressive space is utilized in learning.
This motivates us to identify the space that can elucidate their semantic and complex relationships with small information loss.
Novelty is assured by introducing the topic and hyperbolic as spaces,
and performing contrastive/metric learning tasks to ensure the cooperation of these spaces with Transformer.
Experiments show that Chimera empowers pre-trained models for multi-modal search tasks and demonstrate the ability of the layers it introduces.
更多查看译文
关键词
Multi-modal search,Hyperbolic space,Hyperbolic geometry,Lorentz model,Transformer,Topic models
AI 理解论文
溯源树
样例
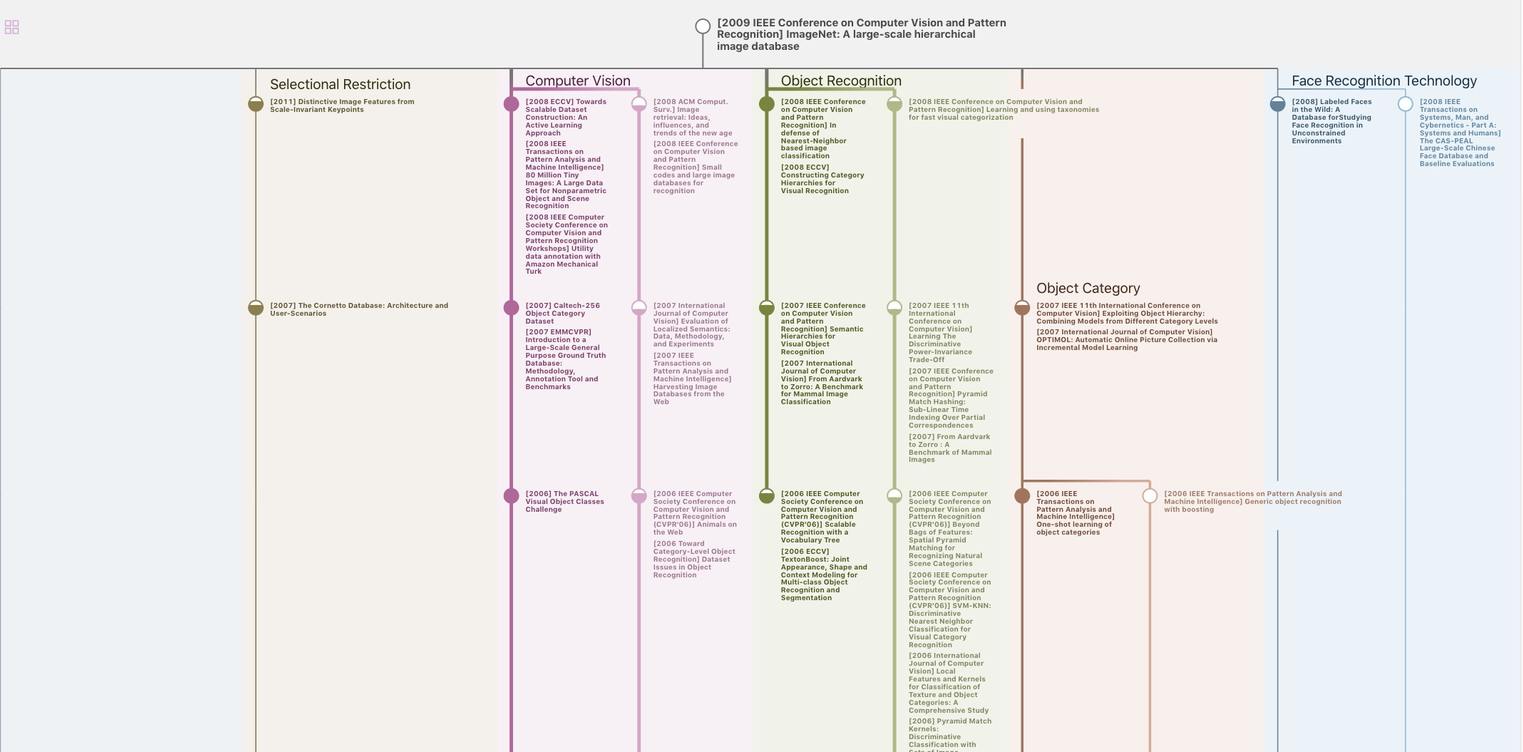
生成溯源树,研究论文发展脉络
Chat Paper
正在生成论文摘要