CAPE: Channel-Attention-Based PDE Parameter Embeddings for SciML
ICLR 2023(2023)
摘要
Scientific Machine Learning (SciML) designs machine learning methods that predict physical systems governed by partial differential equations (PDE). These ML-based surrogate models substitute inefficient and often non-differentiable numerical simulation algorithms and find multiple applications such as weather forecasting, molecular dynamics, and medical applications.
While a number of ML-based methods for approximating the solutions of PDEs have been proposed in recent years, they typically do not consider the parameters of the PDEs, making it difficult for the ML surrogate models to generalize to PDE parameters not seen during training.
We propose a new channel-attention-based parameter embedding (CAPE) component for scientific machine learning models and a simple and effective curriculum learning strategy. The CAPE module can be combined with any kind of ML surrogate model, which can adapt to changing PDE parameters without harming the original model's ability to find approximate solutions to PDEs. The curriculum learning strategy provides a seamless transition between teacher-forcing and fully auto-regressive training.
We compare CAPE in conjunction with the curriculum learning strategy using a PDE benchmark and obtain consistent and significant improvements over the base models. The experiments also show several advantages of CAPE, such as its increased ability to generalize to unseen PDE parameters without substantially increasing inference time and parameter count.
An implementation of the method and experiments are available at \url{https://anonymous.4open.science/r/CAPE-ML4Sci-145B}.
更多查看译文
关键词
machine learning,partial differential equation,attention,generalization
AI 理解论文
溯源树
样例
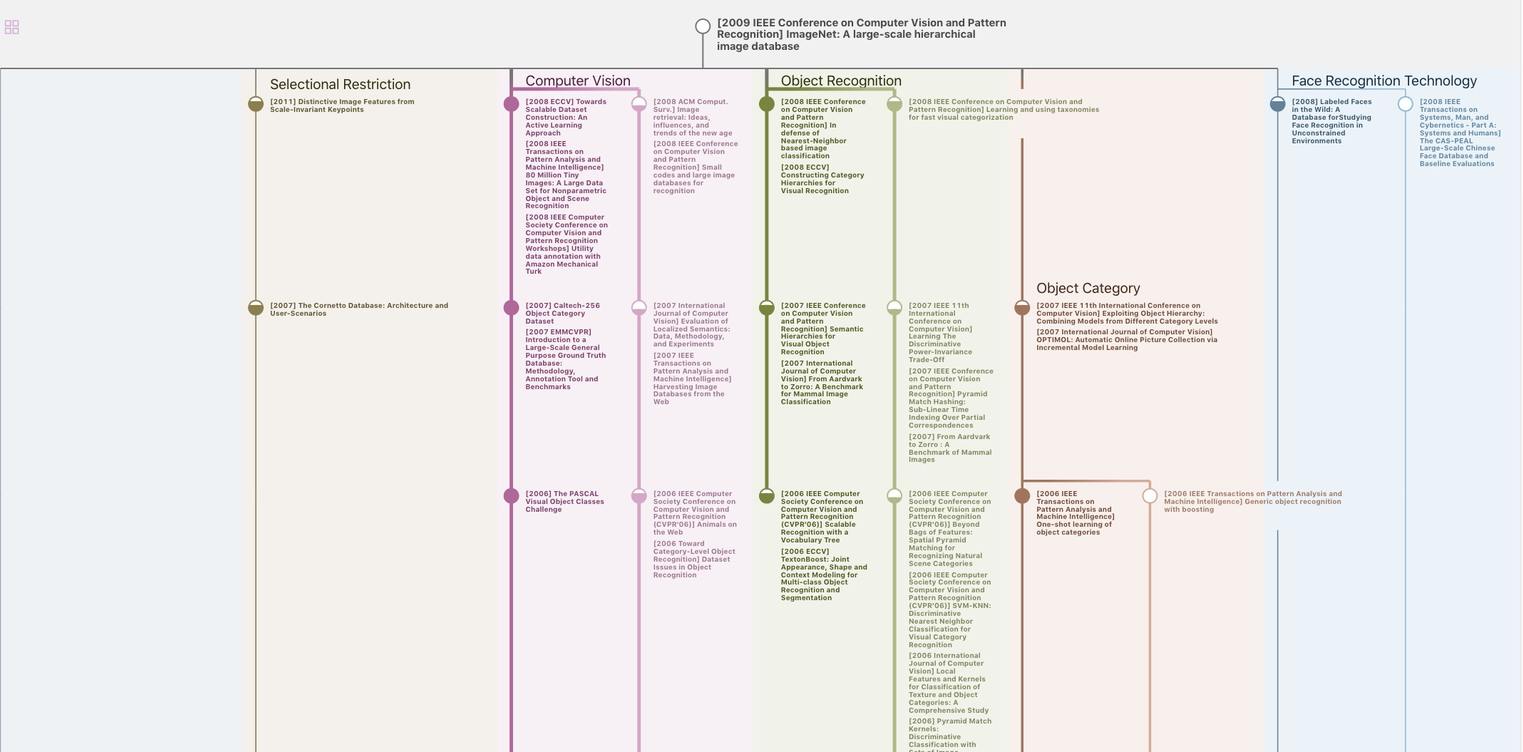
生成溯源树,研究论文发展脉络
Chat Paper
正在生成论文摘要