$Q$-learning with regularization converges with non-linear non-stationary features
ICLR 2023(2023)
摘要
The deep $Q$-learning architecture is a neural network composed of non-linear hidden layers that learn features of states and actions and a final linear layer that learns the $Q$-values of the features. The parameters of both components can possibly diverge. Regularization of the updates is known to solve the divergence problem of fully linear architectures, where features are stationary and known a priori. We propose a deep $Q$-learning scheme that uses regularization of the final linear layer of architecture, updating it along a faster time-scale, and stochastic full-gradient descent updates for the non-linear features at a slower time-scale. We prove the proposed scheme converges with probability 1. Finally, we provide a bound on the error introduced by regularization of the final linear layer of the architecture.
更多查看译文
关键词
Q-learning,Reinforcement Learning,Stochastic Approximation
AI 理解论文
溯源树
样例
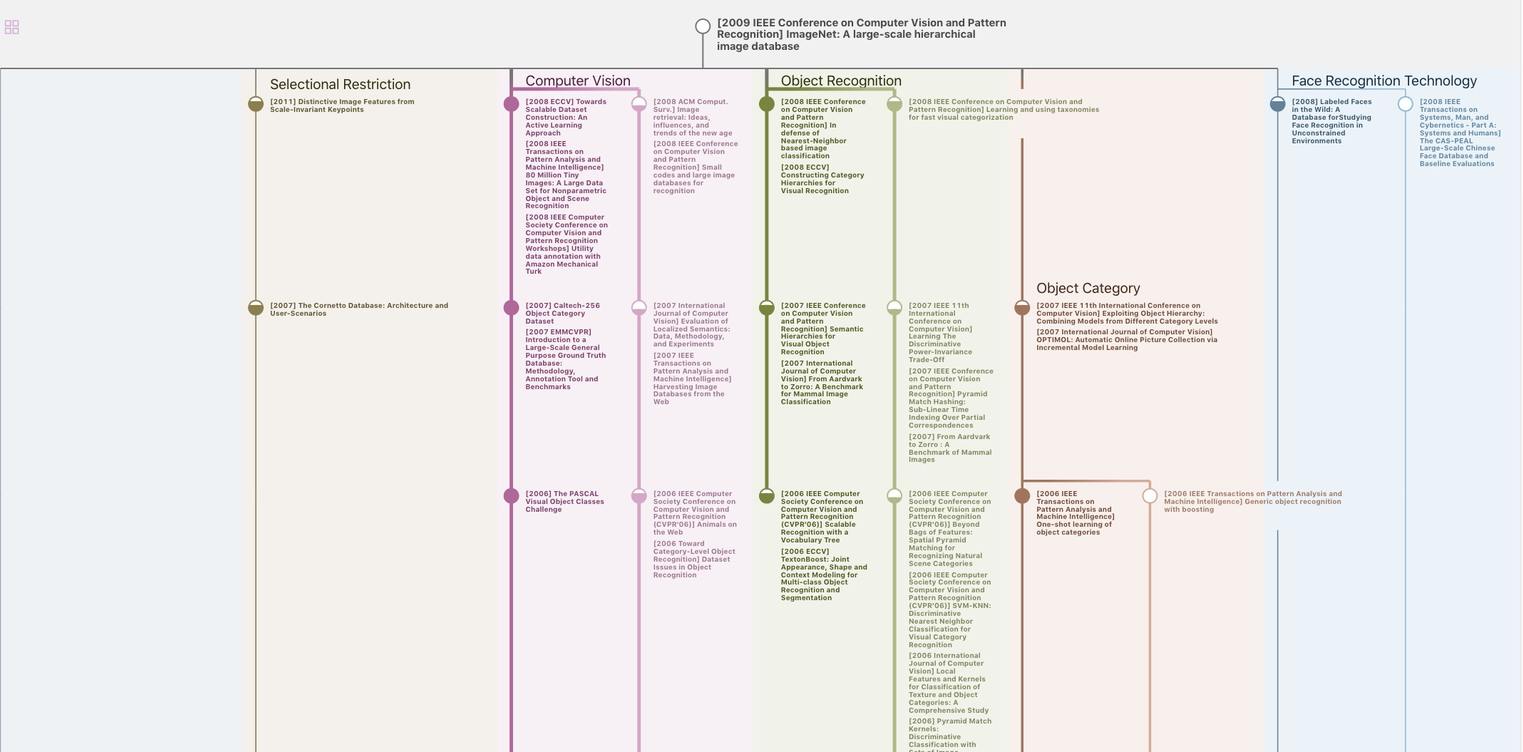
生成溯源树,研究论文发展脉络
Chat Paper
正在生成论文摘要