Self-supervised Speech Enhancement using Multi-Modal Data
ICLR 2023(2023)
摘要
Modern earphones come equipped with microphones and inertial measurement units (IMU). When a user wears the earphone, the IMU can serve as a second modality for detecting speech signals. Specifically, as humans speak to their earphones (e.g., during phone calls), the throat’s vibrations propagate through the skull to ultimately induce a vibration in the IMU. The IMU data is heavily distorted
(compared to the microphone’s recordings), but IMUs offer a critical advantage — they are not interfered by ambient sounds. This presents an opportunity in multi-modal speech enhancement, i.e., can the distorted but uninterfered IMU signal enhance the user’s speech when the microphone’s signal suffers from strong ambient interference?
We combine the best of both modalities (microphone and IMU) by designing a cooperative and self-supervised network architecture that does not rely on clean speech data from the user. Instead, using only noisy speech recordings, the IMU learns to give hints on where the target speech is likely located. The microphone uses this hint to enrich the speech signal, which then trains the IMU to improve subsequent hints. This iterative approach yields promising results, comparable to a supervised denoiser trained on clean speech signals. When clean signals are also available to our architecture, we observe promising SI-SNR improvement. We believe this result can aid speech-related applications in earphones and hearing aids, and potentially generalize to others, like audio-visual denoising.
更多查看译文
关键词
multi-modal,selfsupervise,denoising,iterative algorithm,attention map,expectation maximization,IMU
AI 理解论文
溯源树
样例
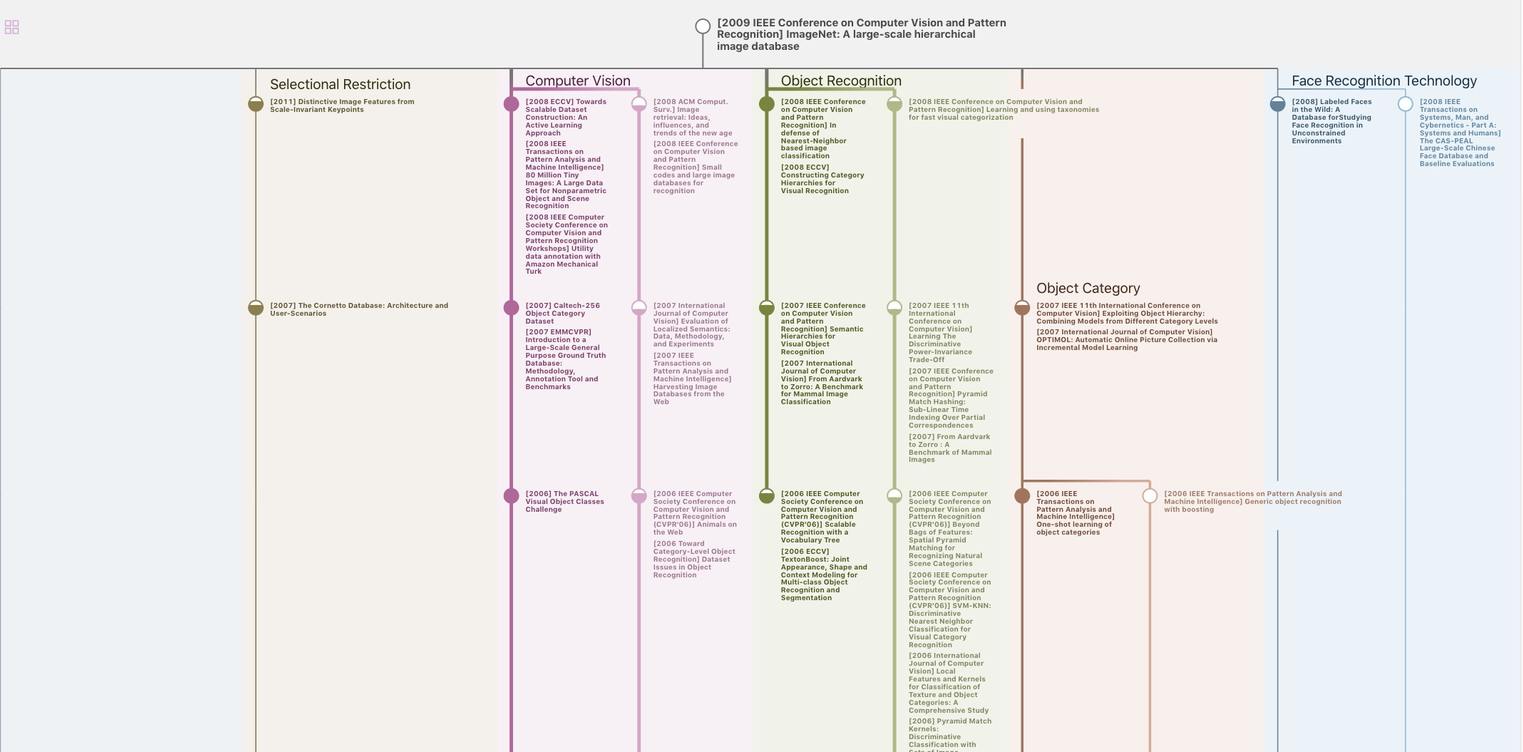
生成溯源树,研究论文发展脉络
Chat Paper
正在生成论文摘要