CAMA: A New Framework for Safe Multi-Agent Reinforcement Learning Using Constraint Augmentation
ICLR 2023(2023)
摘要
With the widespread application of multi-agent reinforcement learning (MARL) in real-life settings, the ability to meet safety constraints has become an urgent problem to solve. For example, it is necessary to avoid collisions to reach a common goal in controlling multiple drones. We address this problem by introducing the Constraint Augmented Multi-Agent framework --- CAMA. CAMA can serve as a plug-and-play module to the popular MARL algorithms, including centralized training, decentralized execution and independent learning frameworks. In our approach, we represent the safety constraint as the sum of discounted safety costs bounded by the predefined value, which we call the safety budget. Experiments demonstrate that CAMA can converge quickly to a high degree of constraint satisfaction and surpasses other state-of-the-art safety counterpart algorithms in both cooperative and competitive settings.
更多查看译文
关键词
Safe,Multi-agent Reinforcement Learning,Augmentation
AI 理解论文
溯源树
样例
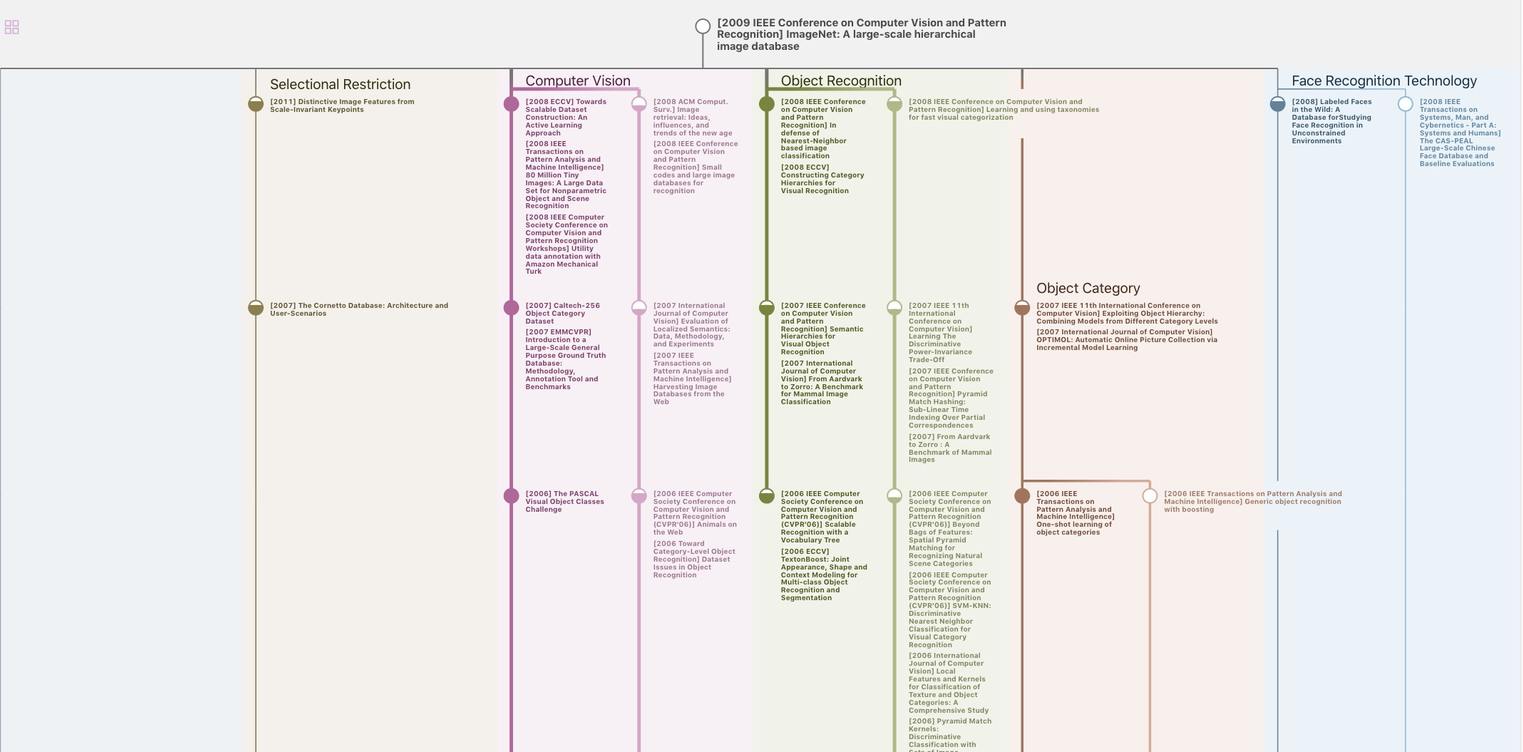
生成溯源树,研究论文发展脉络
Chat Paper
正在生成论文摘要