Learning Efficient Models From Few Labels By Distillation From Multiple Tasks
ICLR 2023(2023)
摘要
We address the challenge of getting efficient yet accurate recognition systems that can be trained with limited labels. Many specialized applications of computer vision (e.g. analyzing X-rays or satellite images) have severe resource constraints both during training and inference. While transfer learning is an effective solution for training on small labeled datasets it still often requires a large base model for fine-tuning. In this paper we present a weighted multi-source distillation method; we distill multiple (diverse) source models trained on different domains, weighted by their relevance for the target task, into a single efficient model using limited labeled data. When the goal is accurate recognition under computational constraints, our approach outperforms both transfer learning from strong ImageNet initializations as well as state-of-the-art semi-supervised techniques such as FixMatch. When averaged over 8 diverse target tasks our method outperform the baselines by 5.6%-points and 4.5%-points, respectively.
更多查看译文
关键词
transfer learning,semi-supervised learning,multi-source distillation
AI 理解论文
溯源树
样例
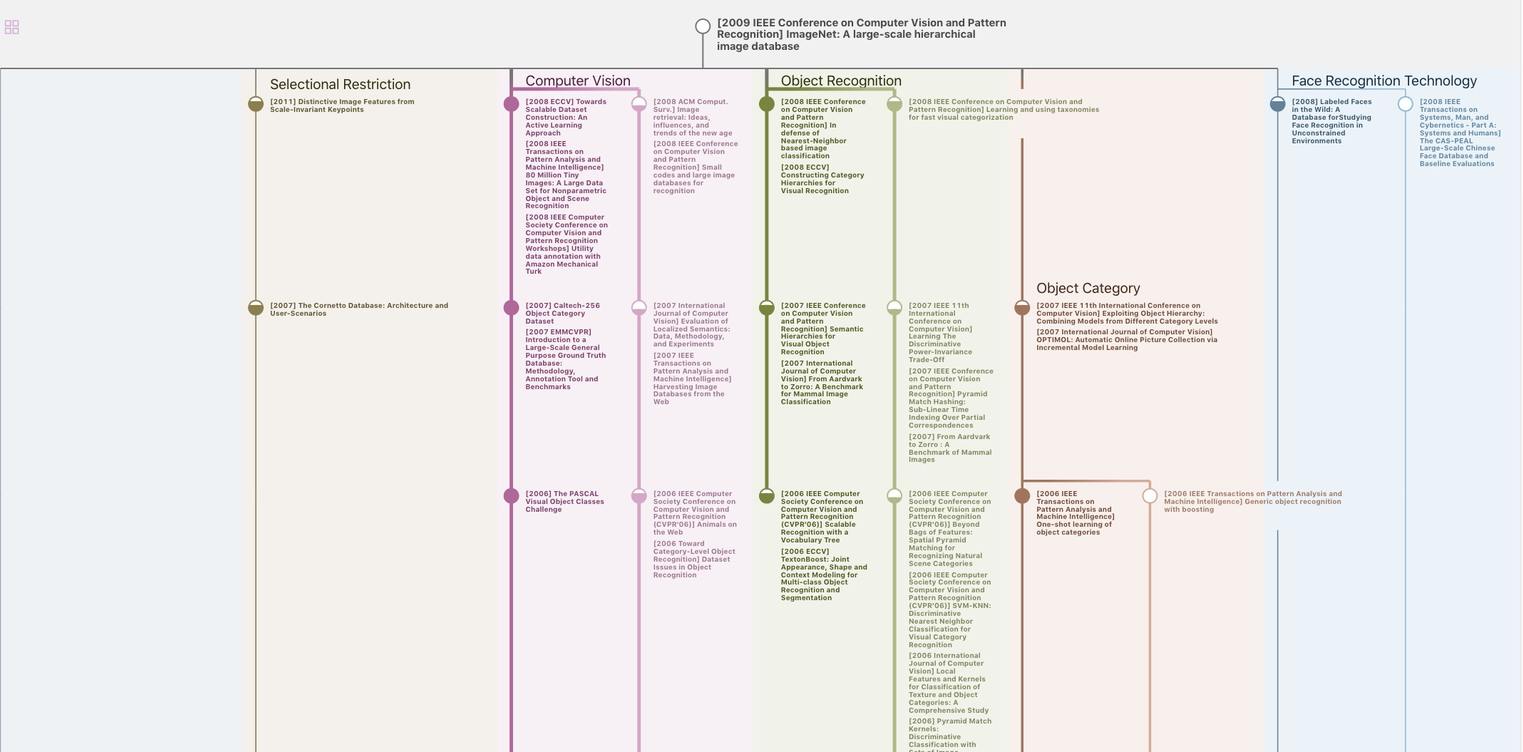
生成溯源树,研究论文发展脉络
Chat Paper
正在生成论文摘要